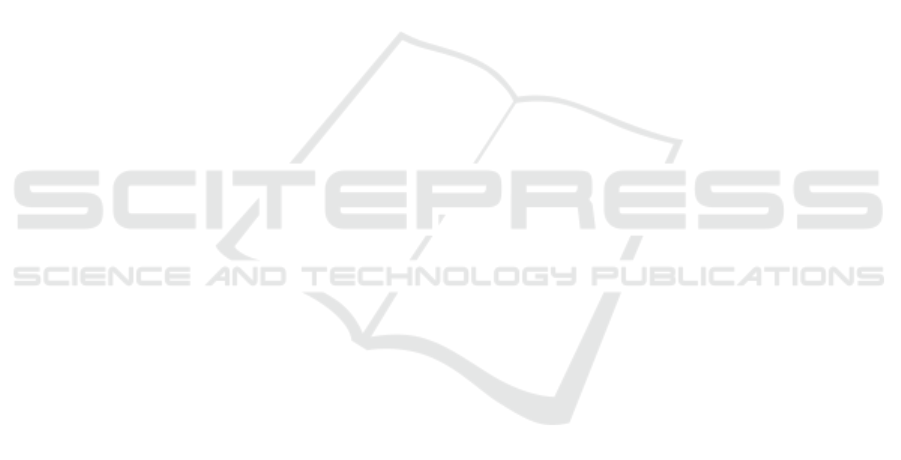
is ongoing in many dimensions of society, and data
value has been extensively explored in the context
of data value chains. Yet, due both to the multi-
dimensionality of data value and to the relevance of
context in quantifying it, there is no consensus of
what characterises data value or how to model it.
In this paper we identify a set of use cases with
the aim of illustrating scenarios where a data value
model can be applied. From these use cases we also
extract a number of requirements that such a vocabu-
lary should cater for. We therefore define the Data
Value Vocabulary (DaVe); a light-weight vocabulary
that enables the representation of data value quantifi-
cation results as linked data. This vocabulary can be
extended with custom data value dimensions that cha-
racterise data value in a specific context. It also allows
for the integration of diverse metrics that span many
data value dimensions and which most likely pertain
to a range of different tools in different formats. We
lead out a preliminary evaluation by (1) leading out
a structured analysis on the features of the ontology,
and (2) by applying the vocabulary to a use case to
validate its usability and capability of modelling data
value in context.
By enabling the comprehensive representation of
data value, DaVe allows users to monitor and assess
the value of data as it occurs within any data value
chain, as data is being exploited. This will in turn
enable the effective management of value, and hence
efficient exploitation of data.
ACKNOWLEDGEMENTS
This research has received funding from the ADAPT
Centre for Digital Content Technology, funded un-
der the SFI Research Centres Programme (Grant
13/RC/2106) and co-funded by the European Regi-
onal Development Fund.
REFERENCES
Ahituv, N. (1980). A Systematic Approach toward Asses-
sing the Value of an Information System. MIS Quar-
terly, 4(4):61.
Al-Saffar, S. and Heileman, G. L. (2008). Semantic Im-
pact Graphs for Information Valuation. In Procee-
dings of the Eighth ACM Symposium on Document
Engineering, DocEng ’08, pages 209–212, New York,
NY, USA. ACM.
Attard, J., Orlandi, F., and Auer, S. (2017). Exploiting the
value of data through data value networks. In Procee-
dings of the 10th International Conference on Theory
and Practice of Electronic Governance ICEGOV’17,
pages 475–484.
Brennan, R., Attard, J., and Helfert, M. (2018). Manage-
ment of data value chains, a value monitoring capabi-
lity maturity model. In Proceedings of 20th Interna-
tional Conference on Enterprise Information Systems
(ICEIS 2018). To Appear.
Castelfranchi, C. (2016). In search of a principled theory of
the value’ of knowledge. SpringerPlus, 5(1):1617.
Chen, Y. (2005). Information Valuation for Information Li-
fecycle Management. In Second International Con-
ference on Autonomic Computing (ICAC’05), pages
135–146. IEEE.
Cri
´
e, D. and Micheaux, A. (2006). From customer data
to value: What is lacking in the information chain?
Journal of Database Marketing & Customer Strategy
Management, 13(4):282–299.
Cyganiak, R., Reynolds, D., and Tennison, J. (2014). The
rdf data cube vocabulary. W3c recommendation,
World Wide Web Consortium (W3C).
Davenport, T. H. (2014). How strategists use big data
to support internal business decisions, discovery and
production. Strategy & Leadership, 42(4):45–50.
Debattista, J., Lange, C., and Auer, S. (2014). Represen-
ting dataset quality metadata using multi-dimensional
views. In Proceedings of the 10th International Con-
ference on Semantic Systems - SEM ’14, pages 92–99,
New York, New York, USA. ACM Press.
Demirkan, H. and Delen, D. (2013). Leveraging the capa-
bilities of service-oriented decision support systems:
Putting analytics and big data in cloud. Decision Sup-
port Systems, 55(1):412 – 421.
Even, A., Shankaranarayanan, G., and Berger, P. D. (2010).
Evaluating a model for cost-effective data quality ma-
nagement in a real-world CRM setting.
Francois, P., Manning, J. G., Whitehouse, H., Brennan, R.,
Currie, T., Feeney, K., and Turchin, P. (2016). A Ma-
croscope for Global History. Seshat Global History
Databank: a methodological overview. Digital Hu-
manities Quarterly, 10(4).
Grau, B. C., Horrocks, I., Motik, B., Parsia, B., Patel-
Schneider, P., and Sattler, U. (2008). Owl 2: The next
step for owl. Web Semant., 6(4):309–322.
Gruber, T. R. (1995). Toward principles for the design of
ontologies used for knowledge sharing. Int. J. Hum.-
Comput. Stud., 43(5-6):907–928.
Jin, H., Xiong, M., and Wu, S. (2008). Information
Value Evaluation Model for ILM. In 2008 Ninth
ACIS International Conference on Software Engineer-
ing, Artificial Intelligence, Networking, and Paral-
lel/Distributed Computing, pages 543–548. IEEE.
Klann, J. G. and Schadow, G. (2010). Modeling the
Information-value Decay of Medical Problems for
Problem List Maintenance. In Proceedings of the 1st
ACM International Health Informatics Symposium,
IHI ’10, pages 371–375, New York, NY, USA. ACM.
Latif, A., Us Saeed, A., Hoefler, P., Stocker, A., and Wag-
ner, C. (2009). The Linked Data Value Chain: A Lig-
htweight Model for Business Engineers. In Procee-
A Semantic Data Value Vocabulary Supporting Data Value Assessment and Measurement Integration
143