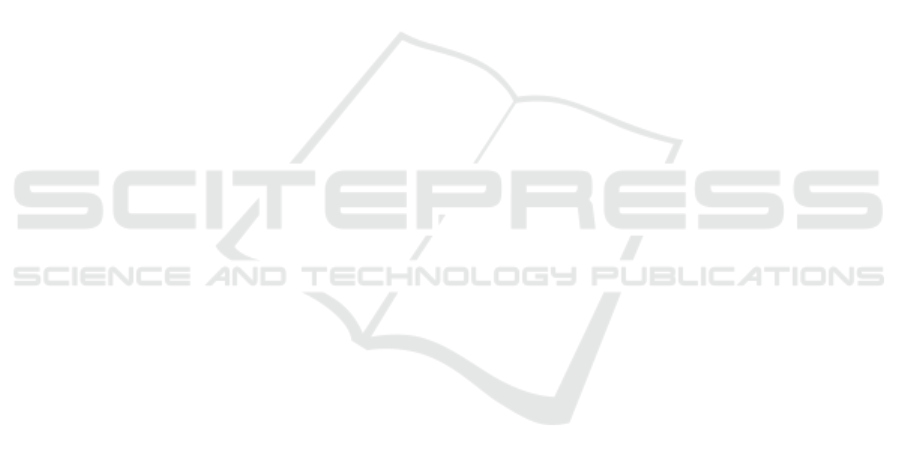
REFERENCES
Aggarwal, C. C. (2017). Outlier Analysis. Springer Inter-
national Publishing.
Ahmed, M., Mahmood, A. N., and Hu, J. (2016). A survey
of network anomaly detection techniques. Journal of
Network and Computer Applications, 60:19–31.
Broy, M. (2006). Challenges in automotive software en-
gineering. In Proceedings of the 28th International
Conference on Software Engineering, ICSE ’06, pages
33–42, New York, NY, USA. ACM.
Chandola, V., Banerjee, A., and Kumar, V. (2009).
Anomaly detection: A survey. ACM computing sur-
veys (CSUR), 41(3):15.
Hawkins, D. M. (1980). Identification of outliers, vol-
ume 11. Springer.
Hawkins, S., He, H., Williams, G., and Baxter, R. (2002).
Outlier detection using replicator neural networks. In
DaWaK, volume 2454, pages 170–180. Springer.
Hoppe, T., Kiltz, S., and Dittmann, J. (2009). Applying in-
trusion detection to automotive IT-early insights and
remaining challenges. Journal of Information Assur-
ance and Security (JIAS), 4:226–235.
IEEE-P802.3ch (2017). IEEE 802.3 Multi-
Gig Automotive Ethernet PHY Task Force.
http://www.ieee802.org/3/ch/index.html. Accessed:
2017-11-28.
IEEE Std 802.3bp-2016 (2016). IEEE Standard for Ether-
net Amendment 4: Physical Layer Specifications and
Management Parameters for 1 Gb/s Operation over a
Single Twisted-Pair Copper Cable.
IEEE Std 802.3bw-2015 (2015). IEEE Standard for Ether-
net Amendment 1: Physical Layer Specifications and
Management Parameters for 100 Mb/s Operation over
a Single Balanced Twisted Pair Cable (100BASE-T1).
Kleberger, P., Olovsson, T., and Jonsson, E. (2011). Se-
curity aspects of the in-vehicle network in the con-
nected car. In 2011 IEEE Intelligent Vehicles Sympo-
sium (IV), pages 528–533.
Lakhina, A., Crovella, M., and Diot, C. (2005). Mining
anomalies using traffic feature distributions. In ACM
SIGCOMM Computer Communication Review, vol-
ume 35, pages 217–228. ACM.
Larson, U. E., Nilsson, D. K., and Jonsson, E. (2008). An
approach to specification-based attack detection for
in-vehicle networks. In IEEE Intelligent Vehicles Sym-
posium, Proceedings, pages 220–225.
Lazarevic, A., Ertoz, L., Kumar, V., Ozgur, A., and Sri-
vastava, J. (2003). A comparative study of anomaly
detection schemes in network intrusion detection. In
Proceedings of the 2003 SIAM International Confer-
ence on Data Mining, pages 25–36. SIAM.
Mahalanobis, P. C. (1936). On the generalised distance in
statistics. Proceedings of the National Institute of Sci-
ences of India, 1936, pages 49–55.
Mantere, M., Uusitalo, I., Sailio, M., and Noponen, S.
(2012). Challenges of machine learning based mon-
itoring for industrial control system networks. In
2012 26th International Conference on Advanced In-
formation Networking and Applications Workshops
(WAINA), pages 968–972. IEEE.
Marchetti, M., Stabili, D., Guido, A., and Colajanni, M.
(2016). Evaluation of anomaly detection for in-
vehicle networks through information-theoretic algo-
rithms. In 2016 IEEE 2nd International Forum on
Research and Technologies for Society and Indus-
try Leveraging a better tomorrow (RTSI), pages 1–6.
IEEE.
Pevn
´
y, T. (2016). Loda: Lightweight on-line detector of
anomalies. Machine Learning, 102(2):275–304.
Sch
¨
olkopf, B., Platt, J. C., Shawe-Taylor, J., Smola, A. J.,
and Williamson, R. C. (2001). Estimating the support
of a high-dimensional distribution. Neural computa-
tion, 13(7):1443–1471.
Shyu, M.-l., Chen, S.-c., Sarinnapakorn, K., and Chang, L.
(2003). A novel anomaly detection scheme based on
principal component classifier. In Proceedings of the
IEEE Foundations and New Directions of Data Min-
ing Workshop, in conjunction with the Third IEEE In-
ternational Conference on Data Mining (ICDM03).
Citeseer.
Stolfo, S. J. (1999). KDD cup 1999 dataset. UCI KDD
repository. http://kdd.ics.uci.edu.
Taylor, A., Japkowicz, N., and Leblanc, S. (2015).
Frequency-based anomaly detection for the automo-
tive can bus. In 2015 World Congress on Indus-
trial Control Systems Security (WCICSS), pages 45–
49. IEEE.
Theissler, A. (2017). Detecting known and unknown faults
in automotive systems using ensemble-based anomaly
detection. Knowledge-Based Systems, 123:163–173.
Vapnik, V. N. (1995). The Nature of Statistical Learning
Theory. Springer, 8(6):187.
Weber, M., Klug, S., Sax, E., Maas, J., and Zimmer,
B. (2018). Embedded Hybrid Anomaly Detection
for Automotive CAN Communication. To be pub-
lished in: Embedded Real Time Software and Systems
(ERTS
2
) 2018.
Xiao, Y., Wang, H., Zhang, L., and Xu, W. (2014). Two
methods of selecting Gaussian kernel parameters for
one-class SVM and their application to fault detection.
Knowledge-Based Systems, 59:75–84.
An Extended Hybrid Anomaly Detection System for Automotive Electronic Control Units Communicating via Ethernet
473