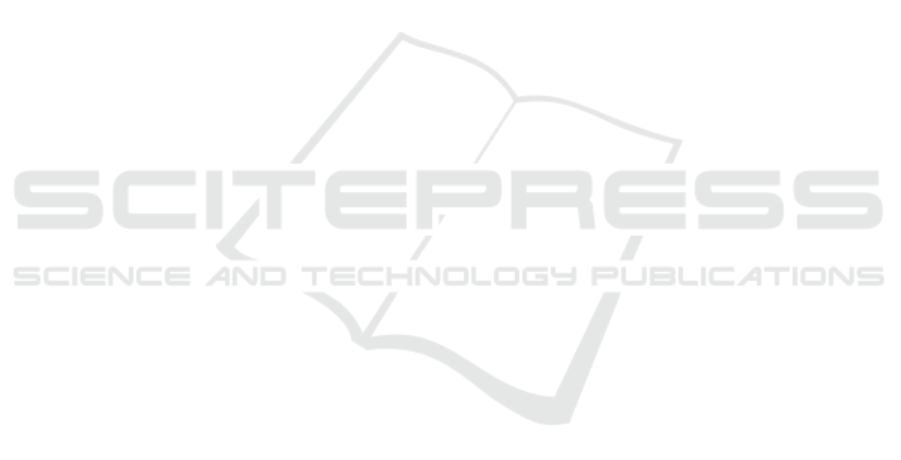
Although (H
¨
onig et al., 2014a) reports a state-
of-the-art result of 71.9% UAR, the dataset used is
smaller and a direct performance comparison cannot
be made.
6 CONCLUSIONS AND FUTURE
WORK
In this paper, we compared the accuracy perfor-
mances of LLD-based group features that comprise
the Sleepiness Sub-Challenge’s baseline feature set
using three different classifiers. Our analysis has re-
vealed the relative discriminating powers of various
group features for a specific classifier as well as aver-
aged over all classifiers. Our top performance, which
achieved improvement over the official baseline, was
obtained using the Random Forest classifier and the
MFCC group feature containing the first three coeffi-
cients. The mentioned MFCC group feature includes
only 6 LLDs out of the 118 that comprise the baseline
feature set.
Future work includes extending the current frame-
work for evaluating relevance for group features in the
context of other paralinguistics tasks as well as devel-
oping feature selection methods that incorporate the
knowledge obtained about group feature relevance in
this paper.
REFERENCES
Breiman, L. (2001). Random forests. Machine Learning,
45(1):5–32.
Chawla, N. V., Bowyer, K. W., Hall, L. O., and Kegelmeyer,
W. P. (2002). Smote: synthetic minority over-
sampling technique. Journal of artificial intelligence
research, pages 321–357.
Davis, S. and Mermelstein, P. (1980). Comparison of para-
metric representations for monosyllabic word recog-
nition in continuously spoken sentences. IEEE trans-
actions on acoustics, speech, and signal processing,
28(4):357–366.
Dhupati, L. S., Kar, S., Rajaguru, A., and Routray, A.
(2010). A novel drowsiness detection scheme based
on speech analysis with validation using simultaneous
eeg recordings. In Automation Science and Engineer-
ing (CASE), 2010 IEEE Conference on, pages 917–
921. IEEE.
Eyben, F. (2016). Real-time speech and music classification
by large audio feature space extraction. Springer.
Eyben, F., W
¨
ollmer, M., and Schuller, B. (2010). opens-
mile: The munich versatile and fast open-source audio
feature extractor. In Proceedings of the international
conference on Multimedia, pages 1459–1462. ACM.
Freund, Y. and Schapire, R. E. (1999). Large Margin Clas-
sification Using the Perceptron Algorithm. Machine
Learning, 37(3):277–296.
Hall, M., Frank, E., Holmes, G., Pfahringer, B., Reutemann,
P., and Witten, I. H. (2009). The weka data min-
ing software: an update. ACM SIGKDD explorations
newsletter, 11(1):10–18.
Hantke, S., Weninger, F., Kurle, R., Ringeval, F., Bat-
liner, A., Mousa, A. E.-D., and Schuller, B. (2016).
I hear you eat and speak: Automatic recognition of
eating condition and food type, use-cases, and im-
pact on asr performance. PloS one, 11(5):e.0154486.
doi:10.1371/journal.pone.0154486.
H
¨
onig, F., Batliner, A., Bocklet, T., Stemmer, G., N
¨
oth, E.,
Schnieder, S., and Krajewski, J. (2014a). Are men
more sleepy than women or does it only look like–
automatic analysis of sleepy speech. In 2014 IEEE In-
ternational Conference on Acoustics, Speech and Sig-
nal Processing (ICASSP), pages 995–999. IEEE.
H
¨
onig, F., Batliner, A., N
¨
oth, E., Schnieder, S., and Kra-
jewski, J. (2014b). Acoustic-prosodic characteristics
of sleepy speech – between performance and interpre-
tation. In Proc. of Speech Prosody, pages 864–868.
Huang, D.-Y., Ge, S. S., and Zhang, Z. (2011). Speaker
State Classification Based on Fusion of Asymmetric
SIMPLS and Support Vector Machines. In INTER-
SPEECH 2011 – 12
th
Annual Conference of the Inter-
national Speech Communication Association, 2011,
Florence, Italy, Proceedings, pages 3301–3304.
Krajewski, J. and Kr
¨
oger, B. J. (2007). Using Prosodic
and Spectral Characteristics for Sleepiness Detection.
In INTERSPEECH 2007 – 8
th
Annual Conference of
the International Speech Communication Association,
August 27-31, Antwerp, Belgium, Proceedings, pages
1841–1844.
Krajewski, J., Wieland, R., and Batliner, A. (2008). An
Acoustic Framework for Detecting Fatigue in Speech
Based Human-Computer-Interaction, pages 54–61.
Springer Berlin Heidelberg, Berlin, Heidelberg.
Lerch, A. (2012). An introduction to audio content analysis:
Applications in signal processing and music informat-
ics. John Wiley & Sons.
McCartt, A. T., Ribner, S. A., Pack, A. I., and Hammer,
M. C. (1996). The scope and nature of the drowsy
driving problem in new york state. Accident Analysis
& Prevention, 28(4):511–517.
Pack, A. I., Pack, A. M., Rodgman, E., Cucchiara, A.,
Dinges, D. F., and Schwab, C. W. (1995). Character-
istics of crashes attributed to the driver having fallen
asleep. Accident Analysis & Prevention, 27(6):769–
775.
Pir, D. and Brown, T. (2015). Acoustic Group Feature Se-
lection Using Wrapper Method for Automatic Eating
Condition Recognition. In INTERSPEECH 2015 –
16
th
Annual Conference of the International Speech
Communication Association, September 6-10, 2015,
Dresden, Germany, Proceedings, pages 894–898.
Pir, D., Brown, T., and Krajewski, J. (2016). Wrapper-
Based Acoustic Group Feature Selection for Noise-
Robust Automatic Sleepiness Classification. In Pro-
ceedings of the 4
th
International Workshop on Speech
Relevant Acoustic Group Features for Automatic Sleepiness Recognition
213