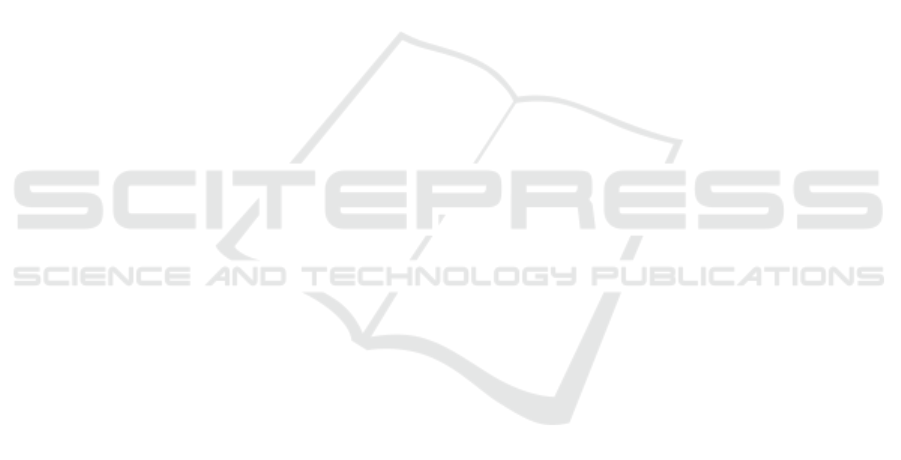
is a significant difference between the performances
in the two groups
3.3 Finding Correlation between the
Metrics
Group 3 has examined the affective e-learning sys-
tem by watching the e-learning materials while us-
ing the three biometric sensors (EEG, eye-tracker,
and camera) to recognize their emotions and con-
trol the e-learning materials delivery during the ex-
periment. The API collected in total, the average of
445,812(reading) x 5 (subjects) = 2,229,060 rows of
raw data during the experiment. However, 122,598
(5.5 percent of the 2,229,060 samples) rows of the raw
data were excluded because of they were invalid (mis-
reading of the data by one or more sensors because
of the users action like eye blinking, head movement,
face turned away from camera, etc.). A combina-
tion of different metrics used by the three sensors
was used by the API to control the delivery of the e-
learning materials and decide whether to change the
materials or not according to a predefined threshold
for each metric. Finally, an eye tracker were used dur-
ing the experiment to detect whether the user is look-
ing into the display or not, hence, detect the level of
engagement. Scatter plots and correlation coefficient
r have been used to find a relationship and measure its
strength and direction between the different metrics
used to detect the emotions and level of engagement
of the affective e-learning system user.
Table 6 summarizes the results of the correlation
coefficient r between the facial expressions metrics
and the EEG metric (Where green, blue, and red col-
ors represent strong, moderate, and weak correlation
in order). The table shows the results of the correla-
tion tests between two groups of metrics representing
two biometric sensors. The EEG metric (long term
excitement) has a strong relationship with two facial
expressions metrics (positive & joy), moderate nega-
tive relationship with other two metrics (anger & sad-
ness), and weak or no relationship with the last two
(negative and fear). The second EEG metric (short
term excitement) shows a weak or no relationship
with any of the facial expressions metrics. The third
EEG metric (frustration) has a moderate negative re-
lationship with three facial expressions metrics (pos-
itive, joy, and fear), and the last EEG metric has a
moderate positive relationship with (positive and joy).
This can be a good indicator that the EEG met-
rics can be replaced by a combination of the five re-
lated metrics (positive, joy, anger, fear, and sadness).
The sixth facial expression metric was not correlated
with any EEG metrics and had no significant change
in value when the participants emotion change de-
tected during the experiment, hence, it can be dis-
carded from the metrics list. Also, it was found that
the two metrics (positive and joy) have the exact val-
ues during the experiment, which means we can dis-
card one of them and use the other. Finally, the fear
metric is not appropriate to be used in the learning
and education context. It may be more suitable for
playing horror games for example, therefore it can be
discarded. On the other hand, the EEG metric (short
term excitement) can be discarded as it had no cor-
relation with any of the facial expression metrics and
no significant change in the participants emotion de-
tection.
In conclusion, the correlation tests show that the
EEG metrics (Long term excitement, frustration, and
engagement) can be replaced by a combination of
three facial expressions metrics (positive, anger, and
sadness).
3.4 Pilot Study Interpretation
• The ANOVA data analysis shows that there is a
significant change in the students performance us-
ing three different approaches.
• Using t-Test analysis, shows that there is no dif-
ference when using traditional education and e-
learning. Comparing the traditional education
with the affective e-learning didnt show much
difference as well. However, comparing the e-
learning with the affective e-learning approaches,
shows a significance change in the performance.
This indicates that using affective e-learning may
enhance the efficiency of e-learning which is the
answer for the second research question.
• In the pilot study, the API used two facial expres-
sions metrics to detect the participants emotions
and change the delivery of the affective e-learning
system materials. These metrics were (joy) and
(sadness) (with a threshold of 0.5 for each). Af-
ter the experiment, analyzing the collected data
shows the learning materials have been changed
three times. It is found that each time the materi-
als have changed, there was a significant change
in the value of the EEG metric (Long term excite-
ment), which has dropped by about 33 percent of
its average value. Also, the EEG metric (Frustra-
tion), which has increased by about 17 percent of
its average value. Finally, the EEG metric (En-
gagement), which has increased by about 13 per-
cent of its average value. This can add a value to
the validity of the facial expressions sensor met-
rics.
Measuring the Engagement of the Learner in a Controlled Environment using Three Different Biosensors
283