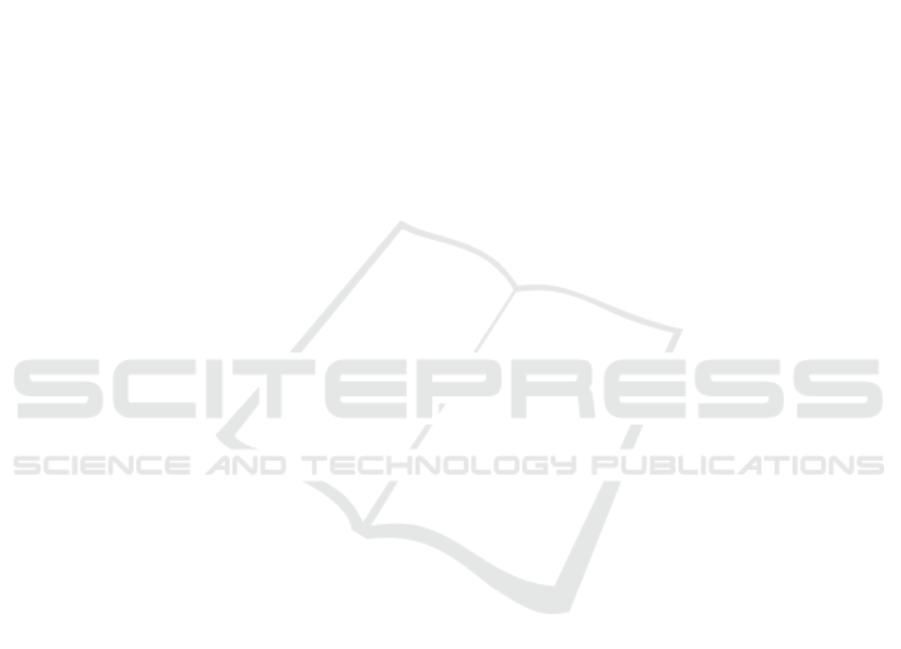
Applying Causal Inference in Educational Data Mining: A Pilot
Study
Walisson Ferreira de Carvalho
1,2
, Bráulio Roberto Gonçalves Marinho Couto
3
, Ana Paula Ladeira
1
,
Osmar Ventura Gomes
1
and Luiz Enrique Zarate
2
1
Centro Universitário UNA, Av. Professor Mário Werneck, 1685, Belo Horizonte, Brazil
2
Pontifícia Universidade Católica de Minas Gerais, Rua Walter Ianni, 255, Belo Horizonte, Brazil
3
Centro Universitário de Belo Horizonte - UniBH, Av. Professor Mário Werneck, 1685, Belo Horizonte, Brazil
Keywords: Causal Inference, Educational Data Mining, e-Learning.
Abstract: Understanding the reasons that leads students to succeed during their course is a challenge for every
Institution of Education, independently of the modality of teaching and learning adopted. In this paper we
use the theory of Causal Inference for analyzing the main factors that causes the success, or failure, of an
engineering student enrolled in an online course of Algorithm . We used data extracted from the Learning
Management System Moodle and, after preprocessing the dataset, analyzed the actions performed by the
students during the six months (20 weeks) that the online course lasted. We concluded that before
submitting an evaluation activity to be assessed, it is important that students analyze the problem
thoroughly. Students that took a little bit longer to submit their work got more chances to be approved.
1 INTRODUCTION
Over the last years a new application of Data Mining
has been emerged and it has been object of studies
for many researchers, the Educational Data Mining
(EDM). This interdisciplinary area of Data Mining
has as its main goal to analyze data from the
education sector in order to solve problems related
to education. According to Romero and Ventura
(2010), although EDM focus on educational data, it
uses techniques of traditional Data Mining.
The Handbook of Educational Data Mining
organized by Romero et al. in 2011 presents some
applications of EDM. Among them, it is possible to
emphasize improvement in quality of the courses,
the opportunity in modeling the profile of students,
increasing performance of students, predicting
performance and others that can improve the quality
of the process of teaching and learning.
Baker and Carvalho (2011) presents a taxonomy
of EDM divided in five sub areas: i) predicting; ii)
clustering; iii) relationship mining; iv) distillation of
data for human judgment; and v) discovery with
models. On the third subarea, Relationship Mining,
according to the authors, the goal is to discover
relationship between variables, being most common
kinds of relationship association, correlation,
sequential pattern and causal mining. In this article
the focus will remain on the causal association
among variables.
Besides the taxonomy, another issue pointed out
by Baker and Carvalho (2011) is the opportunity for
researchers that combine online education and
Educational Data Mining aiming to improve the
process of teaching and learning. This opportunity
emerges from the growth of this modality of
education and the use of Learning Management
System (LMS) or e-learning systems such as Moodle
(https://moodle.com/), Eliademy
(https://eliademy.com/) and others.
In 2011 Judea Pearl won the Alan Turing Award
“For fundamental contributions to artificial
intelligence through the development of a calculus
for probabilistic and causal reasoning.” By causal
reasoning Pearl means that it is necessary to look for
root causes of an event and the importance of
dissociate correlation and causality. After all,
correlation doesn't imply in causation.
The three pillars of Causal Inference theory are
Baysean Network, also created by Pearl in 1985,
structural equation model and "do" operator which
makes possible to make interventions and to
simulate the model. From these pillars and using
454
Ferreira de Carvalho, W., Roberto Gonçalves Marinho Couto, B., Ladeira, A., Ventura Gomes, O. and Zarate, L.
Applying Causal Inference in Educational Data Mining: A Pilot Study.
DOI: 10.5220/0006792504540460
In Proceedings of the 10th International Conference on Computer Supported Education (CSEDU 2018), pages 454-460
ISBN: 978-989-758-291-2
Copyright
c
2019 by SCITEPRESS – Science and Technology Publications, Lda. All rights reserved