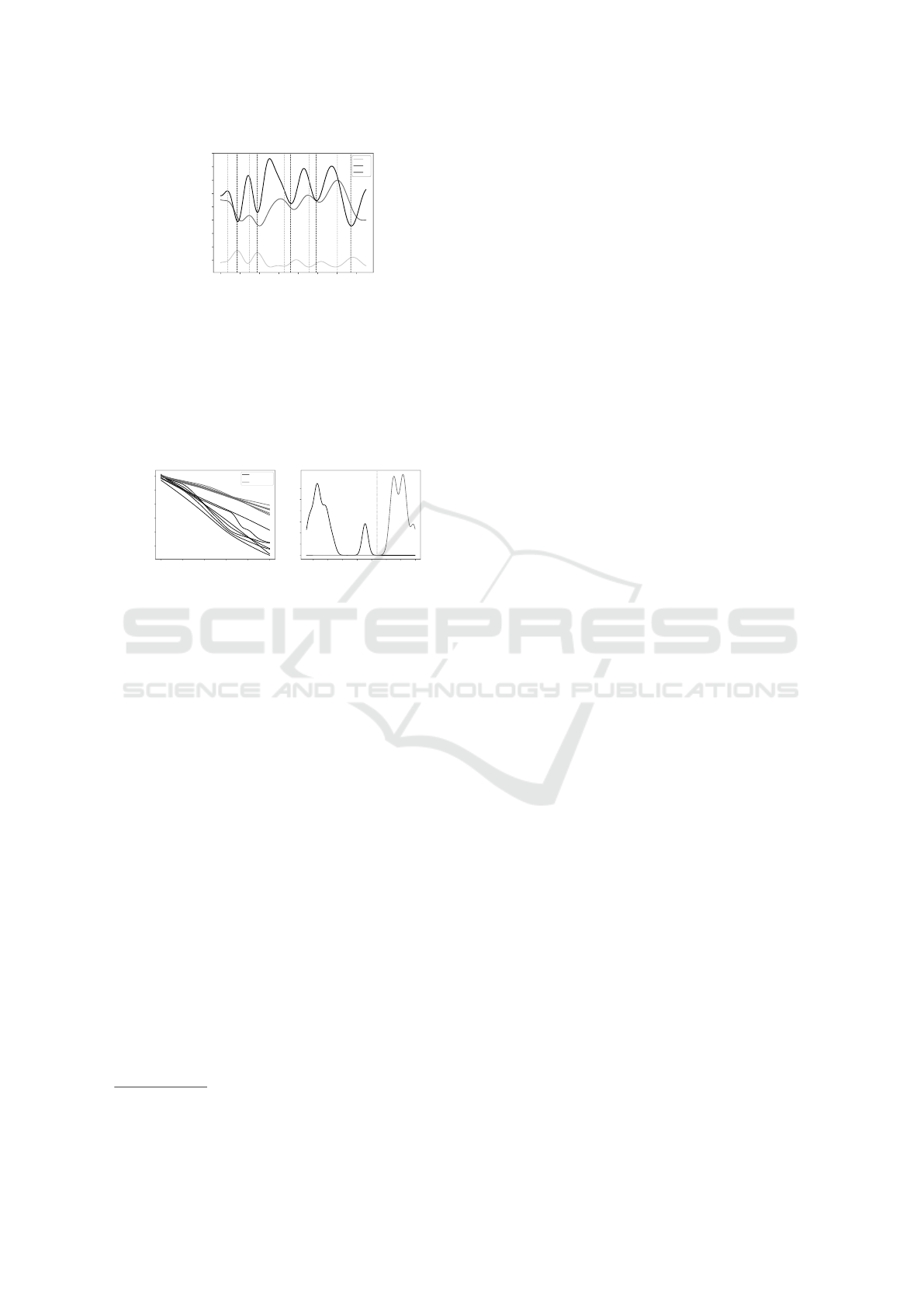
b
v
a
Values normalized
Time[ms]
48000 52000 56000 60000
0.2
0.3
0.4
0.5
0.6
0.7
0.8
0.9
0.1
Figure 10: Found IVN brake situations.
side of Figure 11
9
and got the result the PDF of µ(v)
on the right-hand side of Figure 11. The decision tree
has, like in the simulation, a depth of one. So the
PDFs were examined and result that even in the real
IVN sensor data, there are features with optimal se-
lectivity, like the µ(v).
Velocity in km/h
Pos
Neg
Normalized time
0.5 0.6 0.7 0.8 0.9 1.0
15
16
17
18
19
20
Pos Neg
0.0
0.2
0.4
0.6
0.8
1.0
Probability
1.2
µ(v)
-5.5 -4.5 -3.5 -2.5
Figure 11: Modeled brake examples and resulting PDF.
7 CONCLUSIONS
The experimental results show that the adjusted
method of (Hira and Deshpande, 2016) performs very
well with a simulated situation and situations derived
from real traces of IVN sensor data, shown in Sec-
tion 6. It is able to learn defined situations and find
them in unknown time series.
The automatic fault detection, in the case of me-
chanical wear, is verified with a positive result in a
simulated situation as well as in real traces of IVN
sensor data. Therefore we used positive examples
from test rides and modeled negative examples.
According to the results, the method of modeling
causal relationships between time series can be ap-
plied to sensor signals of IVNs very well. It has to be
adjusted in the future work in order to make it more
robust and decrease the cost in means of computation
time, by applying new techniques like machine learn-
ing algorithms in intermediate steps of the method.
All previously manually defined variables have to be
decided automatically.
9
Samples are divided into small sub windows in order to
get more training data.
REFERENCES
Alippi, C., Roveri, M., and Trov, F. (2014). Learning causal
dependencies to detect and diagnose faults in sensor
networks. In 2014 IEEE Symposium on Intelligent
Embedded Systems (IES), pages 34–41.
Burckhardt, M. (1991). Fahrwerkstechnik: Bremsdynamik
und Pkw-Bremsanlagen. Vogel-Verlag.
Deb, A. K., Kordes, A., and Vermeulen, H. G. H. (2013).
Flexray network runtime error detection and contain-
ment. US Patent 20140047282A1.
Gomeringer, R., Heinzler, M., Kilgus, R., Menges, V.,
N
¨
aher, F., Oesterle, S., Scholer, C., Stephan, A., and
Wieneke, F. (2014). Tabellenbuch Metall. Europa-
Fachbuchreihe f
¨
ur Metallberufe. Europa-Lehrmittel.
Hira, S. and Deshpande, P. S. (2016). Mining precise cause
and effect rules in large time series data of socio-
economic indicators. SpringerPlus, 5(1):1625.
Kerschen, G., Boe, P. D., Golinval, J.-C., and Worden, K.
(2005). Sensor validation using principal component
analysis. Smart Materials and Structures, 14(1):36.
Klimkowski, K. (2016). An artificial neural networks ap-
proach to stator current sensor faults detection for
dtc-svm structure. Power Electronics and Drives, 1
(36)(1).
Kordes, A., Hozhabrpour, H., Wism
¨
uller, R., and Grze-
gorzek, M. (2018). Vehicle-independent interpretation
of sensor signals without a-priori knowledge of their
semantics. In press, VDE GMM-Fachtagung AmE Au-
tomotive meets Electronics, Dortmund, Germany.
Kordes, A., Vermeulen, B., Deb, A., and Wahl, M. (2014).
Startup error detection and containment to improve
the robustness of hybrid flexray networks. In Proceed-
ings of the conference on Design, Automation & Test
in Europe, page 5. European Design and Automation
Association.
Korte, M., Holzmann, F., Kaiser, G., Scheuch, V., and Roth,
H. (2012). Design of a Robust Plausibility Check for
an Adaptive Vehicle Observer in an Electric Vehicle,
pages 109–119. Springer Berlin Heidelberg, Berlin,
Heidelberg.
Mitschke, M. and Wallentowitz, H. (2014). Dynamik der
Kraftfahrzeuge. Springer Vieweg.
Mosteller, F. (1968). Association and estimation in con-
tingency tables. Journal of the American Statistical
Association, 63(321):1–28.
Rosich, A., Frisk, E., Aslund, J., Sarrate, R., and Nejjari, F.
(2012). Fault diagnosis based on causal computations.
IEEE Transactions on Systems, Man, and Cybernetics
- Part A: Systems and Humans, 42(2):371–381.
Shirahama, K., K
¨
oping, L., and Grzegorzek, M. (2016).
Codebook approach for sensor-based human activity
recognition. In Proceedings of the 2016 ACM Interna-
tional Joint Conference on Pervasive and Ubiquitous
Computing: Adjunct. ACM.
Tucker, A. and Liu, X. (2004). A bayesian network ap-
proach to explaining time series with changing struc-
ture. Intell. Data Anal., 8(5):469–480.
Xu, X., Hines, J. W., and Uhrig, R. E. (1999). Sensor vali-
dation and fault detection using neural networks.
VEHITS 2018 - 4th International Conference on Vehicle Technology and Intelligent Transport Systems
544