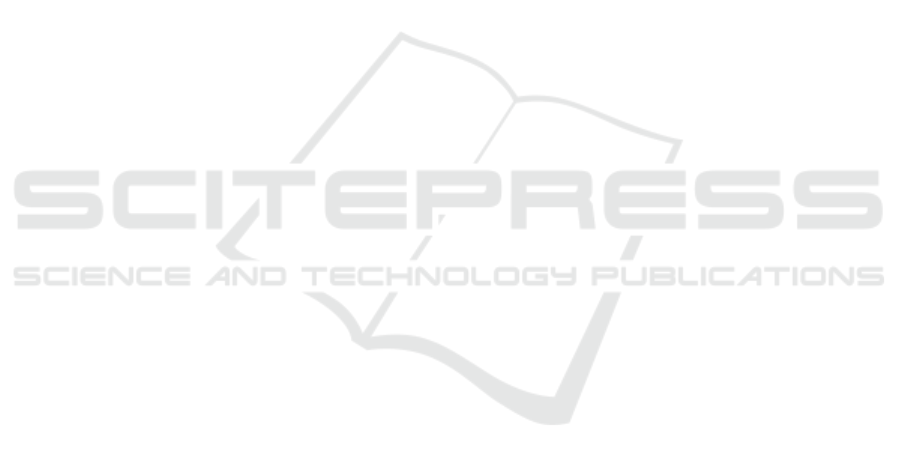
of potential solutions to the problem. For this, a user
interface needs to be designed, which allows people to
plan their own itineraries with the agent, so that more
scenarios are covered. In addition to more scenarios,
the review of people’s own agendas will be more use-
ful as people have a stronger opinion about their own
mobility itineraries. Missing functionality and fea-
tures can, therefore, be more easily detected. Research
on the design on such an interface has already been
started in (Wienken et al., 2017). Future work should
evaluate these design guidelines and implement a user
interface for the agenda planner so that people can
interact with it. A user interface, however, may not
be restricted to a graphical user interface. Currently
intelligent personal assistants, such as Amazon Alexa,
Apple’s Siri, and Google Assistant are becoming more
popular. They allow users to interact with software
using natural speech. Such a electronic personal agent
may support the user then in planning their mobility-
agenda. As the personal assistant already has access
to the calendar and the preferences of the user, it al-
ready has much of the required information. These
personal agents already individually support the user
in agenda planning and mobility planning, but an inte-
grated solution that combines these aspects is missing.
The intelligent agent will act more like a recommender
system in such a scenario, because it does not automat-
ically compute the whole agenda, but suggests date
and locations for certain tasks of the user.
The other aspect of future work focuses on the
optimization problem itself. Furthermore, the men-
tioned drawbacks and missing functionalities have to
be addressed in future work, so that people can in-
struct the agent more precisely in how it should plan
the mobility-oriented agenda. The algorithm’s runtime
also has to be improved, as waiting for 5 minutes in an
interactive scenario for the answer of the agent is unre-
alistic. Additionally, the algorithm can be designed to
respect user profiles, which we have not regarded at all.
A user profile may either be obtained by explicitly let-
ting the user complete a survey which then constructs
the user profile or by learning the user profile while
she interacts with the agent.
ACKNOWLEDGMENTS
This work was partially funded by the German Fed-
eral Ministry of Transport and Digital Infrastructure
(BMVI) for the project “Digitalisierte Mobilit
¨
at – die
Offene Mobilit
¨
atsplattform” (19E16007B).
REFERENCES
Baena-Toquero, M. J., Muros-Cobos, J. L., Rodr
´
ıguez-
Valenzuela, S., and Holgado-Terriza, J. A. (2014). To-
wards sustainability in multi-modal urban planners. In
Connected Vehicles and Expo (ICCVE), 2014 Interna-
tional Conference on, pages 492–497. IEEE.
Boussier, S., Feillet, D., and Gendreau, M. (2007). An exact
algorithm for team orienteering problems. 4OR: A
Quarterly Journal of Operations Research, 5(3):211–
230.
Garcia, A., Vansteenwegen, P., Arbelaitz, O., Souffriau, W.,
and Linaza, M. T. (2013). Integrating public transporta-
tion in personalised electronic tourist guides. Comput-
ers & Operations Research, 40(3):758–774.
Gavalas, D., Konstantopoulos, C., Mastakas, K., Pantziou,
G., and Vathis, N. (2015). Heuristics for the time depen-
dent team orienteering problem: Application to tourist
route planning. Computers & Operations Research,
62:36–50.
Golden, B. L., Levy, L., and Vohra, R. (1987). The orien-
teering problem. Naval Research Logistics, 34(3):307–
318.
Gunawan, A., Yuan, Z., and Lau, H. C. (2014). A mathe-
matical model and metaheuristics for time dependent
orienteering problem. In Proceedings of the 10th In-
ternational Conference of the Practice and Theory of
Automated Timetabling, pages 202–217. Research Col-
lection School Of Information Systems.
Li, F., Cheng, D., Hadjieleftheriou, M., Kollios, G., and
Teng, S.-H. (2005). On trip planning queries in spatial
databases. In International Symposium on Spatial and
Temporal Databases, pages 273–290. Springer.
Louren
c¸
o, H. R., Martin, O. C., and Stutzle, T. (2003). It-
erated local search. International series in operations
research and management science, pages 321–354.
Lu, E. H.-C., Chen, H.-S., and Tseng, V. S. (2017). An
efficient framework for multirequest route planning in
urban environments. IEEE Transactions on Intelligent
Transportation Systems, 18(4):869–879.
Sharifzadeh, M., Kolahdouzan, M., and Shahabi, C. (2008).
The optimal sequenced route query. The VLDB Journal
— The International Journal on Very Large Data Bases,
17(4):765–787.
Vansteenwegen, P. and Van Oudheusden, D. (2007). The
mobile tourist guide: an or opportunity. OR insight,
20(3):21–27.
Wienken, T., Kr
¨
omker, H., and Spundflasch, S. (2017).
Agenda planning-design guidelines for holistic mobil-
ity planning. In International Conference on Human-
Computer Interaction, pages 713–720. Springer.
Wienken, T., Mayas, C., H
¨
orold, S., and Kr
¨
omker, H. (2014).
Model of mobility oriented agenda planning. In Inter-
national Conference on Human-Computer Interaction,
pages 537–544. Springer.
Zografos, K. G. and Androutsopoulos, K. N. (2008). Al-
gorithms for itinerary planning in multimodal trans-
portation networks. IEEE Transactions on Intelligent
Transportation Systems, 9(1):175–184.
Flexible Multicriterial Agenda Planning in Public Transit Systems
567