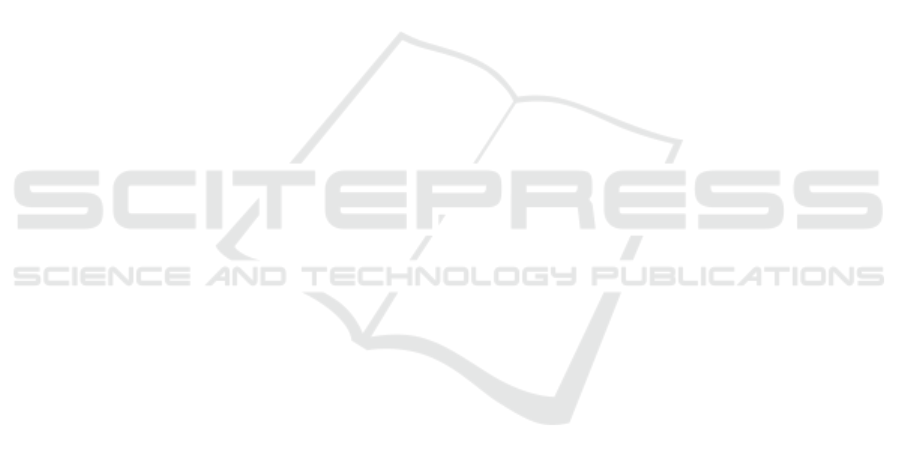
by optical recognition of car plates (Ta et al., 2015).
From the point of view of the driver, she simply drives
through the toll collection point and her account is
debited automatically. Such systems can be further
enhanced with sensor fusion, for example to collect
toll based on the number of occupants in the vehicle
(Nakagawa, 2015a) or to become multi-purpose traf-
fic management solutions (He et al., 2015).
The problem of enabling automatic payments be-
comes more complex, when there is a need to iden-
tify humans, not just objects such as car. An example
of such case is public transport, where each passen-
ger should be ticketed according to her travel. Many
existing solutions require the use of contactless chip
cards or smartphone applications – both of which re-
quire active participation of the passenger in the tick-
eting process (Nakagawa, 2015b). A fully passive
ticketing solutions, so called implicit ticketing, is pos-
sible with BLE (Bluetooth Low Energy) technology,
where the passenger only needs to carry a special BLE
token or a capable smartphone and the payment is per-
formed in the background (Narzt et al., 2015)(Narzt
et al., 2016).
The concept of passive transactions can be ex-
ploited also in the context of citizens using city ser-
vices not related directly to the city infrastructure, for
example: ordering services or documents at a mu-
nicipal office or buying products or services at a lo-
cal retail store. One of notable examples of auto-
matic payment systems in the retail domain is Google
Hands Free (Google, 2015b) proposed by Google.
The Hands Free application uses Bluetooth Low En-
ergy, WiFi, location services, and other sensors on the
user’s device to detect user presence near PoS. This
enables the user to pay hands-free, without getting
out the smartphone or opening the application. Dur-
ing the transaction, verbal declaration of participation
is required from the user (the system is not fully pas-
sive), and identity verification with initials and pro-
file photo from the cashier. Google is also running
early experiments using automated facial identifica-
tion to further simplify the checkout process with in-
store camera.
The payment system that is based on facial recog-
nition to larger extent, called Zero-Effort Payments
(ZEP), has been proposed recently in (Smowton et al.,
2014), where authors interestingly discuss system
evaluation results. The face identification results are
promising, but the recognition is human assisted, i.e.
a ranking of 5 most probable identities is presented
to the human operator and he/she manually chooses
the right one. The recognition process demands a
heavy computational load since a number of faces
are tracked at given moment. Also authors point out
the low face recognition accuracy without support-
ing localization device. In their system BLE localiza-
tion devices are used to significantly reduce the face
recognition error rate. Therefore, it must be noticed
that both Google Hands Free and ZEP are not device-
less systems, i.e. although a user does not need to ma-
nipulate with her device during the payment process,
she needs to carry switched on device during identifi-
cation and customer service.
Also Uniqul system (Anh Tran et al., 2016) has
been deployed to provide fully deviceless payments
authorized with user face image. However, it requires
the user to type a PIN number when face identifica-
tion has a low confidence level or tap the confirma-
tion button on the in-shop tablet in the opposite case.
Therefore, it cannot be considered as fully passive ap-
proach from the end-user perspective.
There are also payment systems based on face
recognition that utilize end-user smartphone camera.
MasterCard has proposed a simple to use mobile so-
lution (Bowyer, 2015) that allows customers to au-
thenticate their online purchases using their own face
images. It refers to the selfie phenomenon, which is
natural for a number of end-users. The application
verifies image authenticity by detecting eyes blink-
ing during image acquisition. MasterCard’s approach
is designed for online shopping, not brick-and-mortar
trade. Contrarily, Lucova, using BLE technology pro-
poses a system called FreshX (Lucnova, 2015) that
also is based on selfie face image authorization, how-
ever it is dedicated for brick-and-mortar cafeterias.
Such approaches, although natural, are neither de-
viceless nor passive, and security concerns can be se-
rious.
There is a number of research and industry effort
related to seamless payments focused on other bio-
metrics than face, e.g. fingerprints, or palm recogni-
tion. For instance Liquid Pay(LiquidPay, 2015) iden-
tifies customers by their fingerprints and, for extra se-
curity, by veins and electrical signals emitted by the
human body. It has been installed in restaurants, fit-
ness clubs and theme parks. Payment systems based
on the Fujitsu PalmSecure technology (Fujitsu, 2011)
recognizing vein patterns in whole palm are being
tested by (Biyo, 2014) or (Lee, 2015) in many cafe-
terias. However, those technologies, although device-
less, stable and relatively mature, cannot be perceived
as passive.
Also, there are significant advancements in the
field of NFC-based contactless payments for EMV
smart cards that have become de facto standard (Al-
liance, 2012) for low-risk transaction authorization in
brick-and-mortar retail trade. Nowadays, this tech-
nology migrates from smartcard to mobile device as
Face-based Passive Customer Identification Combined with Multimodal Context-aware Payment Authorization: Evaluation at Point of Sale
557