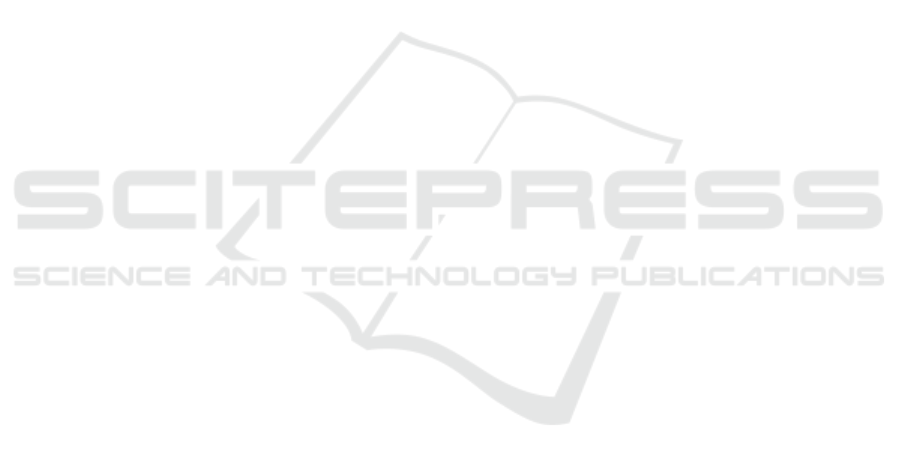
mentioning that the objective of this section is not to
assess the impacts of introducing a NCT. Instead, we
intend to evaluate whether the MGF can distinguish
G
c
and G
m
according to the influence of G
n
. We have
conducted a sensitivity analysis between networks.
The goal is to identify if the NCT keeps the communi-
cation flow provided by CCT or if it introduces alter-
native and significant changes in the communication
flows.
In the first scenario described in Table 2 we explo-
red the number of communication flows in the NCT
of courses under a small, medium, and large scale.
Regarding betweenness, Figure 4(a) indicates a signi-
ficant difference for the correlation, when the growth
ratio is greater than 60%. Additionally, in terms of
closeness, both median (Figure 4(b)) and correlation
(Figure 4(c)) presents a significant difference when
the growth ratio are greater than 40% and 55%, re-
spectively.
We also explored the second scenario for cour-
ses, in which we vary the number of groups inside
G
c
. Figure 5(a) indicates a significant difference for
the betweenness correlation when the growth increa-
ses. They were reached after a growth of 65%. In
fact, the growth threshold for a significant difference
occurs later when the group size is moderate or low.
When it comes to closeness, both median analysis (Fi-
gure 5(b)) and correlation analysis (Figure 5(c)) pre-
sent a significant differences when growth is greater
than 40%. This is interesting as it indicates an incre-
ase in the number of messages in G
m
and a difference
in the network communication topology, as well.
In our third evaluation scenario, we explored the
number of communication flows in the NCT and the
number of groups inside G
c
of a Medium Course un-
der small, medium, and large scale. Regarding be-
tweenness, as depicted in Figures 6(a) and 6(b), we
observe a significant difference for the median and
correlation as the growth ratio reaches 35% and 60%,
respectively. A similar behavior occurs with close-
ness. Figure 6(c) indicates a significant difference for
the closeness median when the growth is greater than
35%. In fact, for the medium size case, only when re-
aching an increase higher than 70% we found a clear
significant difference. Before this value, we observed
an oscillatory behavior around the significance thres-
hold.
6 CONCLUSION
This paper proposes a Mixed Graph Framework
(MGF), which aims at providing a set of quantita-
tive approaches to analyze the complementary of a
new communication tool (NCT) with relation to a cur-
rent communication tool (CCT) in a learning platform
(LP). This is done by measuring when the NCT brings
significant differences in the overall educational com-
munication flow concerning the usage of the CCT.
We model CCT and NCT communication interacti-
ons as the weighted graphs G
c
and G
n
, respectively.
From these graphs, the MGF computes a mixed graph
(G
m
) that combines both G
c
and G
n
considering their
usage. Our approach is then able to identify changes
in overall communication within the educational.
We also evaluated the proposed MGF using synt-
hetic data from which we have conducted a sensi-
tive analysis. The sensitivity analysis is used to com-
pare the weighted closeness and betweenness of both
G
c
and G
m
. Our approach can identify whether G
n
is providing any changes in the entire communica-
tion flow. It is worth mentioning that our method
does not propose adopting the NCT as a replacement
for the CCT to promote communication empower-
ment. Instead, the goal of MGF is to aid managers in
a decision-making process, giving them elements to
conduct what-if analysis while deploying NCTs and
measuring its influence in the entire set of communi-
cation solutions adopted in the educational.
We considered the evolution of a single network
over time including time notion in the proposed fra-
mework as a promising future research. As well as
performing case studies with networks of different si-
zes, which is a useful analysis for educational institu-
tions with scenarios of reorganizations, mergers and
divisions of courses.
ACKNOWLEDGMENTS
The authors would like to thank CNPq, CAPES, and
FAPERJ for partially funding this research.
REFERENCES
Ahuja, R. K., Magnanti, T. L., and Orlin, J. B. (1993). Net-
work Flows: Theory, Algorithms, and Applications.
Pearson, Englewood Cliffs, N.J, 1 edition edition.
Barab
´
asi, A.-L. and Albert, R. (1999). Emergence of sca-
ling in random networks. Science, 286(5439):509–
512.
Benevenuto, F., Rodrigues, T., Cha, M., and Almeida, V.
(2009). Characterizing user behavior in online social
networks. In Proceedings of the ACM SIGCOMM In-
ternet Measurement Conference, IMC, pages 49–62.
Cadima, R., Ferreira, C., Monguet, J., Ojeda, J., and Fer-
nandez, J. (2010). Promoting social network aware-
CSEDU 2018 - 10th International Conference on Computer Supported Education
152