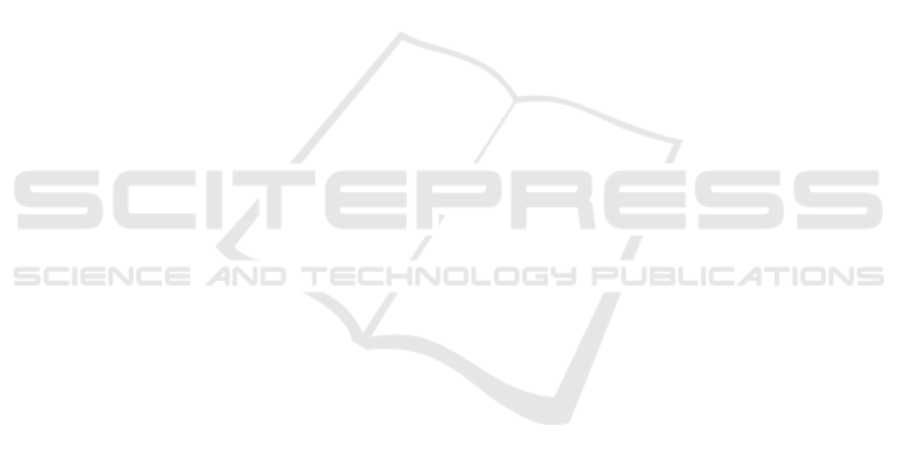
the use of renewable energy through real-time pricing.
Appl. Energy, 113:11–21.
Gela
ˇ
zanskas, L. and Gamage, K. A. A. (2016). Distributed
Energy Storage Using Residential Hot Water Heaters.
Energies, 9(3):127.
Gr
¨
unewald, P. and Torriti, J. (2013). Demand response from
the non-domestic sector: Early UK experiences and
future opportunities. Energy Policy, 61:423–429.
He, X., Keyaerts, N., Azevedo, I., Meeus, L., Hancher,
L., and Glachant, J.-M. (2013). How to engage con-
sumers in demand response: A contract perspective.
Util. Policy, 27:108–122.
Hewicker, C., Hogan, M., and Mogren, A. (2012). Po-
wer Perspectives 2030: On the road to a decarbonised
power sector. Technical report, Roadmap 2050, Den
Haag.
Huber, M., Dimkova, D., and Hamacher, T. (2014). Integra-
tion of wind and solar power in Europe: Assessment
of flexibility requirements. Energy, 69:236–246.
Hurtado, L., Mocanu, E., Nguyen, P., and Kling, W. (2015).
Comfort-constrained demand flexibility management
for building aggregations using a decentralized appro-
ach. In SmartGreens 2015 - 4th Int. Conf. Smart Cities
Green ICT Syst., pages 157–166, Lisbon.
Hurtado, L. A., Mocanu, E., Nguyen, P. H., Gibescu, M.,
and Kamphuis, I. G. (2017). Enabling cooperative be-
havior for building demand response based on exten-
ded joint action learning. IEEE Trans. Ind. Informa-
tics, PP(99):1.
Kalsi, K., Chassin, F., and Chassin, D. (2011). Aggregated
modeling of thermostatic loads in demand response:
A systems and control perspective. In Decis. Cont-
rol Eur. Control Conf. (CDC-ECC), 2011 50th IEEE
Conf., pages 15–20, Orlando. IEEE.
Klaassen, E., Frunt, J., and Slootweg, J. (2014). Method for
Evaluating Smart Grid Concepts and Pilots. In IEEE
Young Res. Symp. 2014 (YRS 2014), pages 1–6, Ghent.
EESA.
Labeeuw, W., Stragier, J., and Deconinck, G. (2015). Po-
tential of Active Demand Reduction With Residential
Wet Appliances: A Case Study for Belgium. IEEE
Trans. Smart Grid, 6(1):315–323.
Lampropoulos, I., Kling, W. L., Ribeiro, P. F., and van den
Berg, J. (2013). History of demand side management
and classification of demand response control sche-
mes. 2013 IEEE Power Energy Soc. Gen. Meet., pages
1–5.
Liu, W., Wu, Q., Wen, F., and Ostergaard, J. (2014). Day-
Ahead Congestion Management in Distribution Sys-
tems Through Household Demand Response and Dis-
tribution Congestion Prices. Smart Grid, IEEE Trans.,
5(6):2739–2747.
Ma, K., Hu, G., and Spanos, C. J. (2015). A Coope-
rative Demand Response Scheme Using Punishment
Mechanism and Application to Industrial Refrige-
rated Warehouses. IEEE Trans. Ind. Informatics,
11(6):1520–1531.
Maharashtra State Blood Transfusion Council (2014). Pre-
servation and Storage of Blood.
Matthews, B. and Craig, I. (2013). Demand side mana-
gement of a run-of-mine ore milling circuit. Control
Eng. Pract., 21(6):759–768.
MatWeb (2017). Unifrax Excelfrax 200 VIP Vacuum Insu-
lation Panel.
Mitra, S., Sun, L., and Grossmann, I. E. (2013). Opti-
mal scheduling of industrial combined heat and power
plants under time-sensitive electricity prices. Energy,
54:194–211.
Morales Gonz
´
alez, R., Shariat Torbaghan, S., Gibescu, M.,
and Cobben, S. (2016). Harnessing the Flexibility of
Thermostatic Loads in Microgrids with Solar Power
Generation. Energies, 9(7):547.
Samad, T. and Kiliccote, S. (2012). Smart grid technolo-
gies and applications for the industrial sector. Comput.
Chem. Eng., 47:76–84.
Ton, D. and Smith, M. (2012). The U.S. Department of
Energy’s Microgrid Initiative. Electr. J., 25(8):84–94.
Verzijlbergh, R. and Lukszo, Z. (2013). Conceptual model
of a cold storage warehouse with PV generation in a
smart grid setting. In 2013 10th IEEE Int. Conf. Net-
working, Sens. Control. ICNSC 2013, pages 889–894,
Evry. IEEE.
Wessling, F. and Blackshear, P. (1973). The Thermal Pro-
perties of Human Blood During the Freezing Process.
Heat Transf., 95(2):246–249.
Wilson, M. B., Luck, R., and Mago, P. J. (2015). A
First-Order Study of Reduced Energy Consumption
via Increased Thermal Capacitance with Thermal
Storage Management in a Micro-Building. Energies,
8:12266–12282.
Yin, R., Kara, E. C., Li, Y., DeForest, N., Wang, K., Yong,
T., and Stadler, M. (2016). Quantifying flexibility of
commercial and residential loads for demand response
using setpoint changes. Appl. Energy, 177:149–164.
Yoon, J. H., Baldick, R., and Novoselac, A. (2014). Dy-
namic Demand Response Controller Based on Real-
Time Retail Price for Residential Buildings. IEEE
Trans. Smart Grid, 5(1):121–129.
Zaparoli, E. L. and de Lemos, M. (1996). Simulation of
Transient Response of Domestic Refrigeration Sys-
tems. In Int. Refrig. Air Cond. Conf., pages 495–500,
West Lafayette. Purdue University.
Zavala, V. M. (2013). Real-time optimization strategies for
building systems. Ind. Eng. Chem. Res., 52(9):3137–
3150.
Demand Response of Medical Freezers in a Business Park Microgrid
129