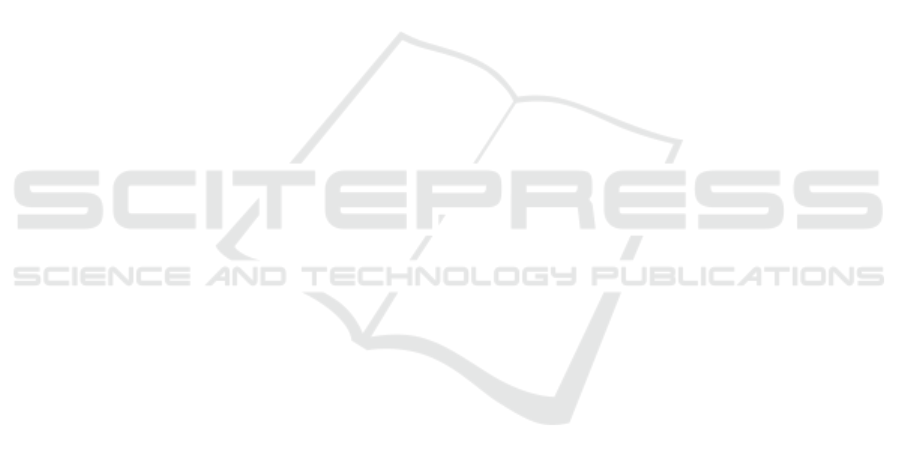
Software Development’, in Agile 2008 Conference.
IEEE, pp. 83–94.
Elliott, R. and Rob (2014) ‘Do students like the flipped class-
room? An investigation of student reaction to a flipped
undergraduate IT course’, in 2014 IEEE Frontiers in
Education Conference (FIE) Proceedings. IEEE, pp. 1–7.
Fryer, L. (2006) ‘Bots as Language Learning Tools’,
Language, Learning and Technology, 10.
Fryer, L. K., Ainley, M., Thompson, A., Gibson, A., &
Sherlock, Z. (2017). Stimulating and sustaining interest
in a language course: An experimental comparison of
Chatbot and Human task partners. Computers in Human
Behavior, 75, 461–468.
Hammond, J. S. (2002) ‘Learning by the Case Method’,
(June), pp. 1–3. Available at: https://www.study.net/
sample/pdf/Learning_by_the_Case_Method.pdf
(Accessed: 6 December 2017).
Hevner, A. and Chatterjee, S. (2010) Design Research in
Information Systems, Theory and Practice. Springer.
Holstein, K., McLaren, B. M. and Aleven, V. (2017)
‘Intelligent tutors as teachers’ aides’, in Proceedings of
the Seventh International Learning Analytics &
Knowledge Conference on - LAK ’17. New York, New
York, USA: ACM Press, pp. 257–266.
Klemm, W. R. (2002). Software Issues for Applying
Conversation Theory For Effective Collaboration Via the
Internet. Retrieved from http://www.cvm.tamu.edu/
wklemm/Files/ConversationTheory.pdf
Lo, C. K. and Hew, K. F. (2017) ‘A critical review of flipped
classroom challenges in K-12 education: possible
solutions and recommendations for future research’,
Research and Practice in Technology Enhanced
Learning, 12(1), p. 4.
Lo, C. K., Hew, K. F. and Chen, G. (2017) ‘Toward a set of
design principles for mathematics flipped classrooms: A
synthesis of research in mathematics education’,
Educational Research Review. Elsevier, 22, pp. 50–73.
Maderer, J. (2017) Jill Watson, Round Three Georgia Tech
course prepares for third semester with virtual teaching
assistants. Available at:
http://www.news.gatech.edu/2017/01/09/jill-watson- round-
three (Accessed: 2 December 2017).
Mclaren, B. M., Scheuer, O. and Mikkátko, J. (2010)
‘Supporting Collaborative Learning and E-Discussions
Using Artificial Intelligence Techniques’, International
Journal of Artificial Intelligence in Education. IOS Press,
20, pp. 1–46.
McNeal, M. (2017) A Siri for Higher Ed Aims to Boost
Student Engagement | EdSurge News. Available at:
https://www.edsurge.com/news/2016-12-07-a-siri-for-
higher-ed-aims-to-boost-student-engagement (Accessed:
30 November 2017).
Pereira, J. and Juanan (2016) ‘Leveraging chatbots to
improve self-guided learning through conversational
quizzes’, in Proceedings of the Fourth International
Conference on Technological Ecosystems for Enhancing
Multiculturality - TEEM ’16. New York, New York,
USA: ACM Press, pp. 911–918.
Perlman, D. J. (2013) ‘Effective teaching and motivation:
application of self-determination theory’, Journal of
Research, Policy & Practice of Teachers & Teacher
Education, 3 (2), pp. 31–37.
PwC Digital Services (2017) ‘Bot.Me: A revolutionary
partnership’. Available at: https://www.pwc.com/us/
en/press-releases/assets/img/bot-me.pdf (Accessed: 17
November 2017).
Ryan, R. M. and Deci, E. L. (2000) ‘Self-determination
theory and the facilitation of intrinsic motivation, social
development, and well-being.’, The American
psychologist, 55(1), pp. 68–78.
Searle, J. R. (1969). Speech act theory. Cambridge:
Cambridge UP.
Seifert, T. L. (2004) ‘Understanding student motivation Self-
efficacy theory’, Educational Research, 46(2).
Sheppard, M. E. et al. (2017) ‘Using a flipped classroom and
the perspective of families to teach medical students about
children with disabilities and special education’, Disability
and Health Journal. Elsevier, 10(4), pp. 552–558.
Srba, I. and Bielikova, M. (2015) ‘Dynamic Group
Formation as an Approach to Collaborative Learning
Support’, IEEE Transactions on Learning Technologies,
8(2), pp. 173–186.
Taha, W., Hedstrom, L.-G., Xu, F., Duracz, A., Bartha, F. A.,
Zeng, Y., … Gunjan, G. (2016) ‘Flipping a first course
on cyber- physical systems’, in Proceedings of the 2016
Workshop on Embedded and Cyber-Physical Systems
Education - WESE ’16. New York, New York, USA:
ACM Press, pp. 1–8.
Tileston, D. W. (2010) ‘What Is Motivation and Why Does
It Matter So Much?’, in What Every Teacher Should
Know About Student Motivation. 2nd edn. Corwin Press,
p.14. Available at: https://us.sagepub.com/sites/default/
files/upm- binaries/34159_Chapter_One.pdf (Accessed:
19 November 2017).
Tissenbaum, M., Matuk, C., Berland, M., Lyons, L., Cocco,
F., Linn, M., … Dillenbourg, P. (2016) ‘Real-time
visualization of student activities to support classroom
orchestration’. International Society of the Learning
Sciences (ISLS).
Vatrapu, R., Teplovs, C., Fujita, N., & Bull, S. (2011)
‘Towards visual analytics for teachers’ dynamic
diagnostic pedagogical decision- making’, in
Proceedings of the 1st International Conference on
Learning Analytics and Knowledge -LAK ’11. New
York, New York, USA: ACM Press, p. 93.
Wang, F. H. (2017) ‘An exploration of online behaviour
engagement and achievement in flipped classroom
supported by learning management system’, Computers
& Education. Pergamon, 114, pp. 79–91.
Winograd, T., & Flores, F. (1986). Understanding computers
and cognition: A new foundation for design. Intellect
Books.
Yilmaz, R. (2017) ‘Exploring the role of e-learning readiness
on student satisfaction and motivation in flipped
classroom.
Enhance Classroom Preparation for Flipped Classroom using AI and Analytics
483