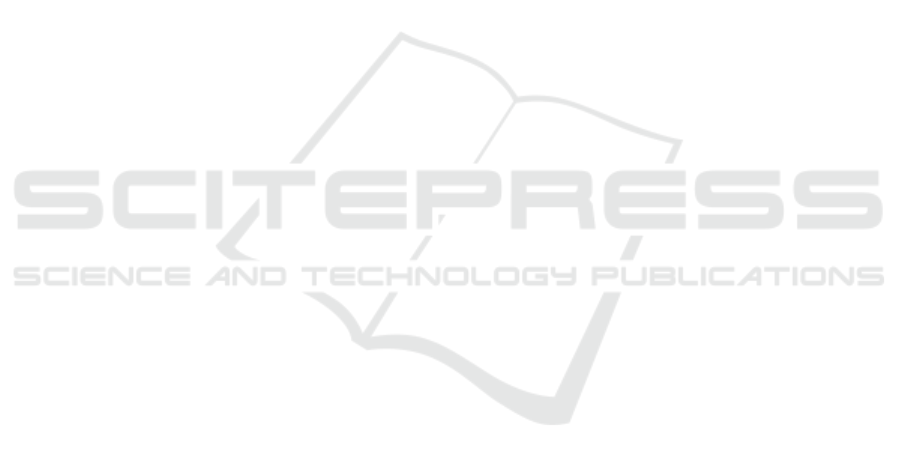
5 CONCLUSIONS
In general, only the one specific LMS can be analy-
zed efficiently using the proposed CAST algorithm.
The wide range of uncontrollable factors (samples
from two different students’ groups, dissimilar in-
terfaces, etc.) significantly reduce the precision of
quantitative detected user behavior difference in both
LMS. Despite the impact of uncontrollable factors,
the CAST algorithm reduces the overestimation of the
learners’ activity.
After the application of the CAST algorithm to
LMS data, we expect to work further in the derivation
of the ”machine learning” models, useful for LMSs’
automation and dynamic adaptation to students’ and
teachers’ needs.
The trend to use Open edX more versus Moodle
can be easily verified by visual data analysis. This
lead to the categorical conclusion that students in the
Open edX environment in comparison with Moodle
LMS are more active.
Some other, not discussed in the paper benefits of
Open edX (as Python assessments coding or LaTeX
formulae writing options) implies more engaged stu-
dents, and better-trained employees in the future. This
is the strategic business decision.
ACKNOWLEDGEMENTS
This research has been supported by a grant from
the international European ERA-NET Project Futu-
rICT2.0 funded under the FLAG-ERA Joint Transna-
tional Call 2016.
REFERENCES
Benjamin, A. (2011). Successful Remembering and
Successful Forgetting: A Festschrift in Honor of Ro-
bert A. Bjork. Taylor & Francis.
Bjork, R. (1994). Memory and meta-memory considerati-
ons in the training of human beings.
Cennamo, K. S. (2012). Learning from Video, pages 1876–
1879. Springer US, Boston, MA.
Coppola, G. and O’Higgins, N. (2015). Youth and the Cri-
sis: Unemployment, Education and Health in Europe.
Routledge Studies in Labour Economics. Taylor &
Francis.
Cornelius-White, J. H. D. and Motschnig, R. (2012).
Person-Centered Learning, pages 2596–2599. Sprin-
ger US, Boston, MA.
DeGroot, M. and Schervish, M. (2013). Probability and
Statistics: Pearson New International Edition. Pear-
son Education Limited.
Ifenthaler, D. and Seel, N. M. (2012). Stochastic Models
of Learning, pages 3192–3194. Springer US, Boston,
MA.
Kapenieks, K. (2013). ebig3 system portal, available at:
http://ebig3.eu/v2/.
Kumar, V. and Reinartz, W. (2006). Customer relationship
management: a database approach. Wiley.
Maniar, N. J. (2012). Video-Based Learning, pages 3403–
3405. Springer US, Boston, MA.
Mosteller, F. (1958). Stochastic models for the learning pro-
cess. Proceedings of the American Philosophical So-
ciety, 102(1):53–59.
Okoshi, T., Ramos, J., Nozaki, H., Nakazawa, J., Dey,
A. K., and Tokuda, H. (2015). Reducing users’ per-
ceived mental effort due to interruptive notifications
in multi-device mobile environments. In Proceedings
of the 2015 ACM International Joint Conference on
Pervasive and Ubiquitous Computing, UbiComp ’15,
pages 475–486, New York, NY, USA. ACM.
Pijeira D
´
ıaz, H. J., Santofimia Ruiz, J., Ruip
´
erez-Valiente,
J. A., Mu
˜
noz Merino, P. J., and Delgado Kloos, C.
(2016). A demonstration of analyse: A learning ana-
lytics tool for open edx. In Proceedings of the Third
(2016) ACM Conference on Learning @ Scale, L@S
’16, pages 329–330, New York, NY, USA. ACM.
Romero, C., Ventura, S., and Garc
´
ıa, E. (2008). Data mi-
ning in course management systems: Moodle case
study and tutorial. Comput. Educ., 51(1):368–384.
Seel, N. M., editor (2012). Blended Online Learning, pages
465–465. Springer US, Boston, MA.
Sikos, L. (2011). Web Standards: Mastering HTML5, CSS3,
and XML. Apressus Series. Apress.
TULVING, E. and Craik, F. (2000). The Oxford Handbook
of Memory. Oxford Library of Psychology Series. Ox-
ford University Press.
Wickham, H. and Grolemund, G. (2016). R for Data
Science: Import, Tidy, Transform, Visualize, and Mo-
del Data. O’Reilly Media.
Zumel, N. and Mount, J. (2014). Practical Data Science
with R. Manning Publications Company.
CSEDU 2018 - 10th International Conference on Computer Supported Education
512