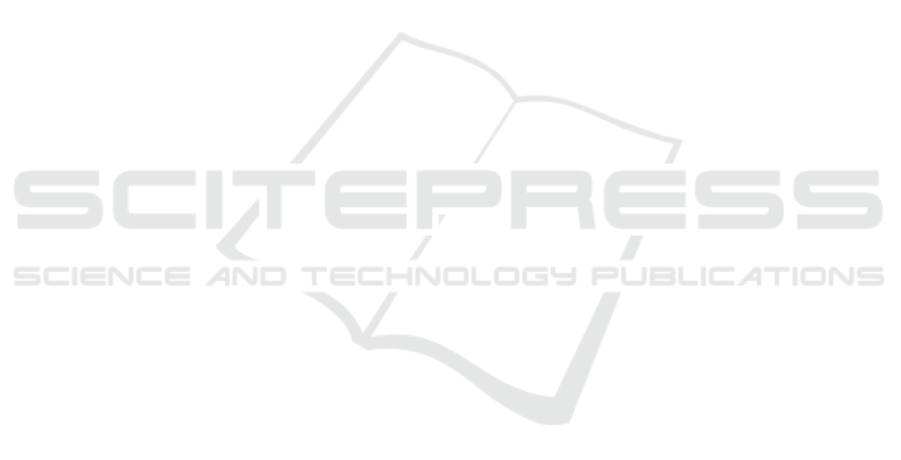
ACKNOWLEDGEMENTS
The present study was developed in the scope of
the Smart Green Homes Project [POCI-01-0247-
FEDER-007678], a co-promotion between Bosch
Termotecnologia S.A. and the University of Aveiro.
It is financed by Portugal 2020 under the Competitive-
ness and Internationalization Operational Program,
and by the European Regional Development Fund.
This work was also partially supported by research
grant SFRH/BD/94270/2013.
REFERENCES
Abowd, G. D., Dey, A. K., Brown, P. J., Davies, N., Smith,
M., and Steggles, P. (1999). Towards a better under-
standing of context and context-awareness. In Proc.
of the 1st international symposium on Handheld and
Ubiquitous Computing, pages 304–307.
Ankerst, M., Breunig, M. M., Kriegel, H.-P., and Sander, J.
(1999). OPTICS: ordering points to identify the clus-
tering structure. ACM SIGMOD Record, 28(2):49–60.
Antunes, M., Gomes, D., and Aguiar, R. L. (2016). Scalable
semantic aware context storage. Future Generation
Computer Systems, 56:675–683.
Antunes, M., Gomes, D., and Aguiar, R. L. (2017a). To-
wards IoT data classification through semantic fea-
tures. Future Generation Computer Systems.
Antunes, M., Gomes, D., Barraca, J. P., and Aguiar, R. L.
(2017b). Vehicular dataset for road assessment condi-
tions. In Procedings in the third IEEE Annual Inter-
national Smart Cities Conference (ISC2 2017).
Arthur, D. and Vassilvitskii, S. (2007). K-means++: The
advantages of careful seeding. In Proceedings of the
Eighteenth Annual ACM-SIAM Symposium on Dis-
crete Algorithms, SODA ’07, pages 1027–1035. So-
ciety for Industrial and Applied Mathematics.
Chen, K.-C. and Lien, S.-Y. (2014). Machine-to-machine
communications: Technologies and challenges. Ad
Hoc Networks, 18:3–23.
Datta, S. K., Bonnet, C., Costa, R. P. F. D., and Härri, J.
(2016). Datatweet: An architecture enabling data-
centric iot services. In 2016 IEEE Region 10 Sym-
posium (TENSYMP), pages 343–348.
Dempster, A. P., Laird, N. M., and Rubin, D. B. (1977).
Maximum likelihood from incomplete data via the
EM algorithm. Journal of the Royal Statistical So-
ciety, Series B, 39(1):1–38.
Dey, A. K. (2001). Understanding and using context. Per-
sonal and Ubiquitous Computing, 5(1):4–7.
Ester, M., Kriegel, H.-P., Sander, J., and Xu, X. (1996).
A density-based algorithm for discovering clusters a
density-based algorithm for discovering clusters in
large spatial databases with noise. In Proceedings of
the Second International Conference on Knowledge
Discovery and Data Mining, KDD’96, pages 226–
231. AAAI Press.
Fantacci, R., Pecorella, T., Viti, R., and Carlini, C. (2014).
Short paper: Overcoming iot fragmentation through
standard gateway architecture. In 2014 IEEE World
Forum on Internet of Things (WF-IoT), pages 181–
182.
Jarmasz, M. and Szpakowicz, S. (2004). Roget’s thesaurus
and semantic similarity. In Recent Advances in Nat-
ural Language Processing III, page 111. John Ben-
jamins Publishing Company.
Jesus, R., Antunes, M., Gomes, D., and Aguiar, R. (2017).
Extracting knowledge from stream behavioural pat-
terns. In Proceedings of the 2nd International Con-
ference on Internet of Things, Big Data and Security.
SCITEPRESS - Science and Technology Publications.
Lloyd, S. (1982). Least squares quantization in pcm. In-
formation Theory, IEEE Transactions on, 28(2):129–
137.
Miller, G. A. and Charles, W. G. (1991). Contextual corre-
lates of semantic similarity. Language and Cognitive
Processes, 6(1):1–28.
Perera, C., Zaslavsky, A., Christen, P., and Georgakopoulos,
D. (2014). Context aware computing for the internet
of things: A survey. IEEE Communications Surveys
Tutorials, 16(1):414–454.
Pham, D. T., Dimov, S. S., and Nguyen, C. D. (2005). Se-
lection of k in k-means clustering. Proceedings of the
Institution of Mechanical Engineers, Part C: Journal
of Mechanical Engineering Science, 219(1):103–119.
Ridler, T. and Calvard, S. (1978). Picture thresholding using
an iterative selection method. IEEE Transactions on
Systems, Man, and Cybernetics, 8(8):630–632.
Robert, J., Kubler, S., Traon, Y. L., and Främling, K. (2016).
O-mi/o-df standards as interoperability enablers for
industrial internet: A performance analysis. In IECON
2016 - 42nd Annual Conference of the IEEE Industrial
Electronics Society, pages 4908–4915.
Rousseeuw, P. J. (1987). Silhouettes: A graphical aid to
the interpretation and validation of cluster analysis.
Journal of Computational and Applied Mathematics,
20:53–65.
Satopaa, V., Albrecht, J., Irwin, D., and Raghavan, B.
(2011). Finding a "kneedle" in a haystack: Detecting
knee points in system behavior. In 2011 31st Interna-
tional Conference on Distributed Computing Systems
Workshops. IEEE.
Schubert, E., Sander, J., Ester, M., Kriegel, H. P., and Xu,
X. (2017). DBSCAN revisited, revisited. ACM Trans-
actions on Database Systems, 42(3):1–21.
Tibshirani, R., Walther, G., and Hastie, T. (2001). Estimat-
ing the number of clusters in a data set via the gap
statistic. Journal of the Royal Statistical Society: Se-
ries B (Statistical Methodology), 63(2):411–423.
Ward, J. H. (1963). Hierarchical grouping to optimize an
objective function. Journal of the American Statistical
Association, 58(301):236–244.
Wortmann, F., Flüchter, K., et al. (2015). Internet of
things. Business & Information Systems Engineering,
57(3):221–224.
The Impact of Clustering for Learning Semantic Categories
327