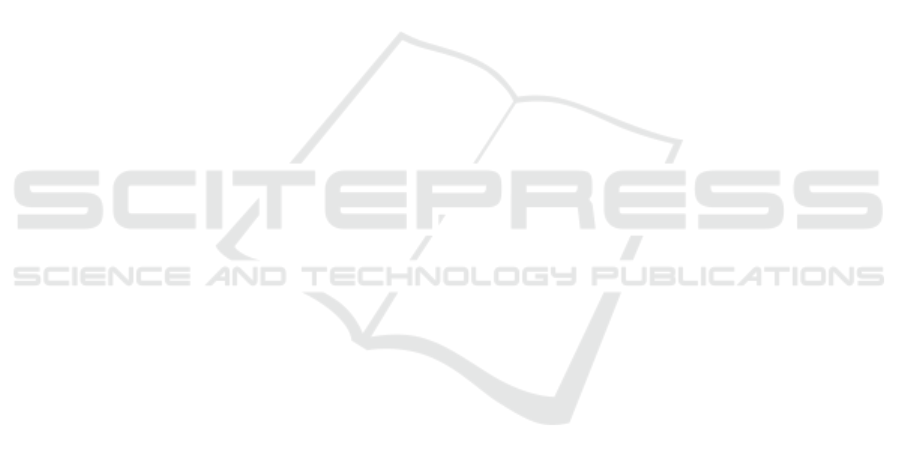
topic. Our view of this topic and the validation of the
problem will be presented in the Section 3. Section 4
presents the discussion and results obtained. Finally,
the conclusions of this study will be presented in the
Section 5.
2 BACKGROUND
The monitoring of the activities performed by ageing
people may be performed in controlled or
uncontrolled environments. Firstly, the controlled
environments considered in this study are the smart
environments (e.g., smart homes), where the ageing
people are living, equipped with several sensors for
the recognition of the activities. Finally, the
uncontrolled environments considered in this study
are the different environments in real life, using the
mobile devices for the data acquisition and further
recognition of the activities.
Smart environments used for the recognition of
the activities performed by ageing people may be
equipped with cameras, temperature sensors,
altimeter sensors, accelerometer sensors, contact
switches, pressure sensors and Radio-frequency
identification (RFID) sensors. The recognition of the
activities in these environments are performed using
server-side processing methods. Botia et al. (2012)
used the cameras for the recognition of the presence
of the ageing people in home office, kitchen, living
room and outdoor spaces, and several activities,
including making coffee, walking on stairs and
working on a computer.
In (Chernbumroong, Cang, Atkins, and Yu,
2013), the authors used the altimeter, accelerometer
and temperature sensors for the recognition of
brushing teeth, feeding, dressing, sleeping, walking,
lying, ironing, walking on stairs, sweeping, washing
dishes and watching TV. (Kasteren and Krose, 2007)
implemented a method that used pressure sensors,
accelerometer sensors and contact switches for the
recognition of bathing, eating and toileting activities.
The accelerometers and RFID sensors may be
used for the recognition of pushing a shopping cart,
sitting, standing, walking, phone calling, taking
picture, running, lying, wiping, switching on skin
conditioner, hand shaking, reading, jumping and hair
brushing activities (Hong, Kim, Ahn, and Kim,
2008).
Other studies making use of only one type of
sensors available in smart environments. Firstly,
other authors used only accelerometer for the
recognition of making coffee, brushing teeth and
boiling water activities (Liming, Hoey, Nugent,
Cook, and Zhiwen, 2012). Secondly, other authors
used only RFID sensors for the recognition of phone
calling, preparing a tea, preparing a meal, making
soft-boiled eggs, using the bathroom, taking out the
trash, setting the table, eating, drinking, preparing
orange juice, cleaning the table, cleaning a toilet,
cleaning the kitchen, making coffee, sleeping,
getting a drink, getting a snack, using a dishwasher,
using a microwave, taking a shower, adjusting the
thermostat, using a washing machine, using the
toilet, vacuuming, leaving the house, reading,
receiving a guest, boiling a pot of tea, doing laundry,
boiling water, brushing hair, shaving face, washing
hands, watching TV and brushing teeth activities
(Cheng, Tsai, Liao, and Byeon, 2009; Danny,
Matthai, and Tanzeem, 2005; Hoque and Stankovic,
2012). Finally, other authors used ZigBee wireless
sensors for the recognition of watching TV,
preparing a meal and preparing a tea activities
(Suryadevara, Quazi, and Mukhopadhyay, 2012).
Related to the use of the data acquired from the
mobile devices, the implemented methods for the
recognition of activities may be implemented locally
on the mobile devices as a mobile application or
server-side, requiring a constant network connection.
Another challenge in the use of the mobile devices
for the recognition of activities is related to the
positioning of the mobile device, that affects the
reliability of the recognition methods. In addition,
the use of these devices should be adapted to the
hardware condition of these devices, such as limited
processing, battery, and storage capabilities.
The most used sensor for the recognition of
activities is the accelerometer sensor embedded in
the mobile devices, enabling the recognition of
several activities, including rowing, walking,
walking on stairs, jumping, jogging, running, lying,
standing, getting up, cycling, sitting, falling and
travelling with different transportation facilities
(Büber and Guvensan, 2014; Cardoso, Madureira,
and Pereira, 2016; Ivascu, Cincar, Dinis, and Negru,
2017; Khalifa, Lan, Hassan, Seneviratne, and Das,
2017; Tsai, Yang, Shih, and Kung, 2015).
The combination of the data acquired from the
accelerometer and the Global Positioning System
(GPS) receiver embedded on the mobile devices can
increase the number and accuracy of the recognition
of activities, including the sitting, standing, walking,
lying, walking on stairs, cycling, falling, jogging,
running, playing football and rowing (Ermes,
Parkka, Mantyjarvi, and Korhonen, 2008; Fortino,
Gravina, and Russo, 2015; Zainudin, Sulaiman,
Mustapha, and Perumal, 2015).
HSP 2018 - Special Session on Healthy and Secure People
270