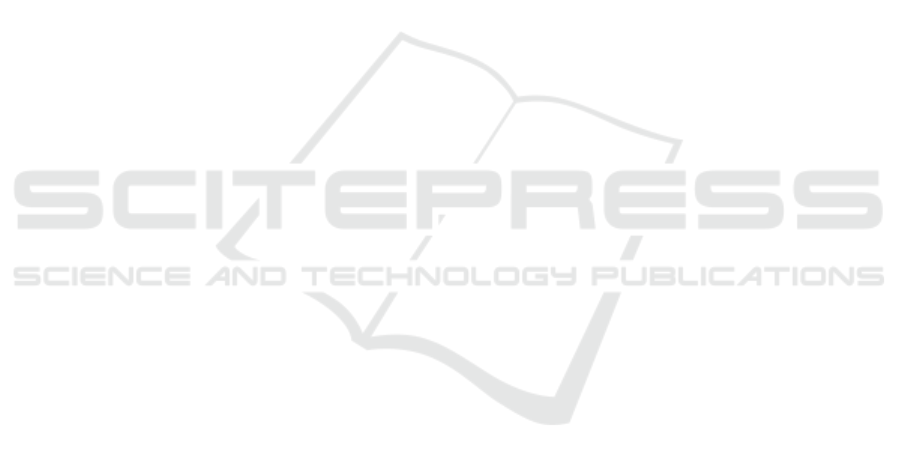
3.6 Wealth Gap Widen
Schmitz (2014) claimed that the use of big data in the
credit reporting field can foster the discrimination,
due to the data analysis subjective rules the machine
will give an aid to the smartest customers with the
best services and best remedial measure, which will
widen the gap between the wealthy and poor
individuals. As the Hurley and Adebayo (2016)
explained in the report, the big data tools will increase
the risk of creating a system of "creditworthiness by
association", which combine the individuals’
families, religions, sexual orientation and other
relevant information. All information will figure out
the individual’s credit score, whether you can pay for
a load is not determined by how much you can earn,
but the group you are in, which will further aggravate
discrimination. Like the example of Kevin Johnson,
he became the proxies of sensitive information like
the race and vulnerable attributes. In this example, the
big data could not eliminate the prejudice, but
aggravate the existed bias.
4 CONCLUSION
In this paper, we report the grey area about the use of
big data, particularly for credit reporting system that
has biases and selection on vulnerable groups of
people. Big data enthusiasts argue that all the data can
be managed by credit reporting and the big data will
lead us to live happily and comfortably. While we can
find is that the data maybe objective, the process of
data categorization and evaluation can be subjective.
Moreover, the ways data will be collected or
diagnosed largely depend on the existing social
resources. Individuals who have contributed their
data to the credit reporting system, have no or few
effective actions to modify the “unreasonable” data.
The vulnerable people will be likely to be trapped in
a vicious circle by precision marketing or have been
considered as low credit groups due to potential
discrimination in income, social status, race, politics
or religion. On the other hand, the wealthy people can
possess the best remedial measures and excellent
services. While we need to use the big data to build
the completeness and soundness of the credit
reporting system. However, we should also pay
attention to ethical issues raised by the big data and
find betters to manage and treat each individual fairly
and equally.
REFERENCES
Alloway, T., 2015. Big data: Credit where credit's due
[Online]. Available from:
https://www.ft.com/content/7933792e-a2e6-11e4-
9c06-00144feab7de (Accessed: 2017).
Angwin, J., 2014. Dragnet nation: A quest for privacy,
security, and freedom in a world of relentless
surveillance. Macmillan.
Barocas, S., Selbst, A. D., 2016. ‘Big data's disparate
impact’, Cal. L. Rev., 104, p. 671.
Bilogrevic, I., Freudiger, J., De Cristofaro, E., & Uzun, E.
(2014) ‘What’s the gist? privacy-preserving
aggregation of user profiles’, In: KUTYŁOWSKI, M.
& VAIDYA, J. (eds.) Computer Security - ESORICS
2014: 19th European Symposium on Research in
Computer Security, Wroclaw, Poland, September 7-11,
2014. Proceedings, Part II. Cham: Springer
International Publishing.
Bureau, C. F. P., 2012. Analysis of Differences between
Consumer-and Creditor-Purchased Credit Scores.
Calders, T. & Custers, B., 2013. ‘What Is Data Mining and
How Does It Work?’ In: Custers, B., Calders, T.,
Schermer, B. & Zarsky, T. (eds.) Discrimination and
Privacy in the Information Society: Data Mining and
Profiling in Large Databases. Berlin, Heidelberg.
Carrns, A., 2015. New Credit Score Systems Could Open
Lending to More Consumers [Online]. Available from:
http://lippmancpas.com/new-credit-score-systems-
could-open-lending-to-more-consumers (Accessed:
2017).
Cetorelli, N., & Gambera, M., 2001. ‘Banking market
structure, financial dependence and growth:
International evidence from industry data’, The Journal
of Finance, 56(2), pp. 617-648.
Citron, D. K., & Pasquale, F., 2014. ‘The scored society:
due process for automated predictions’, Wash. L. Rev.,
89, 1.
Commission, F. T., 2013. Section 319 of the Fair and
Accurate Credit Transactions Act of 2003: Fifth Interim
Federal Trade Commission Report to Congress
Concerning the Accuracy of Information in Credit
Reports.
Guzelian, C. P., Stein, M. A., & Akiskal, H. S., 2015.
‘Credit Scores, Lending, and Psychosocial Disability’,
BUL Rev., 95, p.1807.
Hilda, J. J., Srimathi, C., & Bonthu, B., 2016. ‘A review on
the development of big data analytics and effective data
visualization techniques in the context of massive and
multidimensional data’, Indian Journal of Science and
Technology, 9(27), pp.1-13.
Hurley, M., & Adebayo, J., 2016. ‘Credit scoring in the era
of big data’, Yale JL & Tech., 18, p.148.
Jacobs, S., 2015.Report: More Than Half of Mobile
Location Data is Inaccurate [Online]. Available from:
http://streetfightmag.com/2015/05/14/report-more-
than-half-of-mobilelocation-data-is -inaccurate
(Accessed: 2017).
Big data: a tool for fighting discrimination and empowering
groups (2014) [Online]. Available from:
A Discussion Paper on the Grey Area – The Ethical Problems Related to Big Data Credit Reporting
353