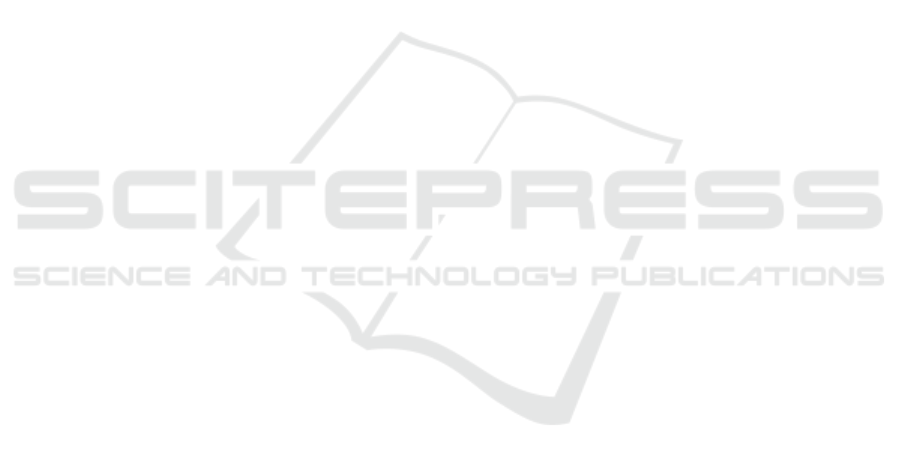
hout any linearizaton and conventional model dis-
cretization. The introduced NdMPC can consider re-
asonable prediction horizon as regular MPC. Furt-
her emphasis will be placed on the selection analy-
sis of adequate numerical method, incremental offset-
free form and on a general point-to-point motion
in unconstrained and constrained robot workspace.
ACKNOWLEDGEMENT
This paper was supported by The Czech Academy of
Sciences, Institute of Information Theory and Auto-
mation.
REFERENCES
Arimoto, S. (1996). Control Theory of Non-linear Mecha-
nical Systems. Oxford Press.
Belda, K., B
¨
ohm, J., and P
´
ı
ˇ
sa, P. (2007). Concepts of
model-based control and trajectory planning for pa-
rallel robots. In Proc. of the 13th IASTED Int. Conf.
on Robotics and Applications, pages 15–20.
Belda, K. and Novotn
´
y, P. (2012). Path simulator for ma-
chine tools and robots. In Proc. of MMAR IEEE Int.
Conf., pages 373–378, Poland.
Belda, K. and Rovn
´
y, O. (2017). Predictive control of 5
DOF robot arm of autonomous mobile robotic system
motion control employing mathematical model of the
robot arm dynamics. In 21st Int.Conf. on Process Con-
trol, pages 339–344.
Belda, K. and Vo
ˇ
smik, D. (2016). Explicit generalized pre-
dictive control of speed and position of PMSM drives.
IEEE Trns. Ind. Electron., 63(2):3889–3896.
Belda, K. and Z
´
ada, V. (2017). Predictive control for offset-
free motion of industrial articulated robots. In Proc.
of MMAR IEEE Int. Conf., pages 688–693, Poland.
Butcher, J. (2016). Numerical Methods for Ordinary Diffe-
rential Equations. Wiley.
Chung, W., Fu, L., and Kr
¨
oger, T. (2016). Motion Control,
pages 163–194. Springer.
Colombo, A. W., Karnouskos, S., Shi, Y., Yin, S., and
Kaynak, O. (2016). Industrial cyber-physical systems
scanning the issue. Proc. of the IEEE, 104(5):899–
903.
Faulwasser, T., Weber, T., Zometa, P., and Findeisen, R.
(2017). Implementation of nonlinear model predictive
path-following control for an industrial robot. IEEE
Tran. Control Syst. Technology, 25(4):1505–1511.
Franklin, G., Powell, J., and Workman, M. (1998). Digi-
tal Control of Dynamic Systems (3rd ed.). Addison
Wesley.
Groothuis, S., Stramigioli, S., and Carloni, R. (2017).
Modeling robotic manipulators powered by varia-
ble stiffness actuators: A graph-theoretic and port-
hamiltonian formalism. IEEE Trans. on Robotics,
33(4):807–818.
Houska, B., Ferreau, H., and Diehl, M. (2011). ACADO
toolkit – An open-source framework for automatic
control and dynamic optimization. Optimal Control
Applications and Methods, 32(3):298–312.
Kirches, C. (2011). Fast numerical methods for mixed-
integer nonlinear model-predictive control. Springer.
Kothare, M. V., Balakrishnan, V., and Morari, M.
(1996). Robust constrained model predictive con-
trol using linear matrix inequalities. Automatica,
32(10):13611379.
Lawson, C. and Hanson, R. (1995). Solving least squares
problems. Siam.
Maciejowski, J. (2002). Predictive Control: With Con-
straints. Prentice Hall.
Negri, G. H., Cavalca, M. S. M., and Celeberto, A. L.
(2017). Neural nonlinear model-based predictive con-
trol with faul tolerance characteristics applied to a ro-
bot manipulator. Int. J. Innovative Computing Infom-
ration and Control, 13(6):1981–1992.
Ordis, A. and Clarke, D. (1993). A state-space descrip-
tion for GPC controllers. J. Systems SCI., 24(9):1727–
1744.
Ostafew, C. J., Schoellig, A. P., Barfoot, T. D., and Col-
lier, J. (2016). Learning-based nonlinear model pre-
dictive control to improve vision-based mobile robot
path tracking. J. Field Robotics, 33(1):133–152.
Othman, A., Belda, K., and Burget, P. (2015). Physical mo-
delling of energy consumption of industrial articulated
robots. In Proc. of the 15th Int. Conf. on Control, Au-
tomation and Systems, pages 784–789, Korea. ICROS.
Siciliano, B., Sciavicco, L., Villani, L., and Oriolo, G.
(2009). Robotics – Modelling, Planning and Control.
Springer.
SPS IPC Drives (2017). Internationale fachmesse f
¨
ur elek-
trische automatisierung, systeme und komponenten.
Online: < https://www.mesago.de/en/SPS/ >.
Wang, L. (2009). Model Predictive Control System Design
and Implementation Using MATLAB. Springer.
Wilson, J., Charest, M., and Dubay, R. (2016). Non-
linear model predictive control schemes with applica-
tion on a 2 link vertical robot manipulator. Robotics
and Computer-Integrated Manufacturing, 41:23–30.
Z
´
ada, V. and Belda, K. (2017). Application of hamiltonian
mechanics to control design for industrial robotic ma-
nipulators. In Proc. of MMAR IEEE Int. Conf., pages
390–395, Poland.
Zanelli, A., Domahidi, A., Jerez, J., and Morari, M.
(2017). FORCES NLP: an efficient implementation
of interior-point methods for multistage nonlinear
nonconvex programs. Int. J. Control, pages 1–17.
ICINCO 2018 - 15th International Conference on Informatics in Control, Automation and Robotics
80