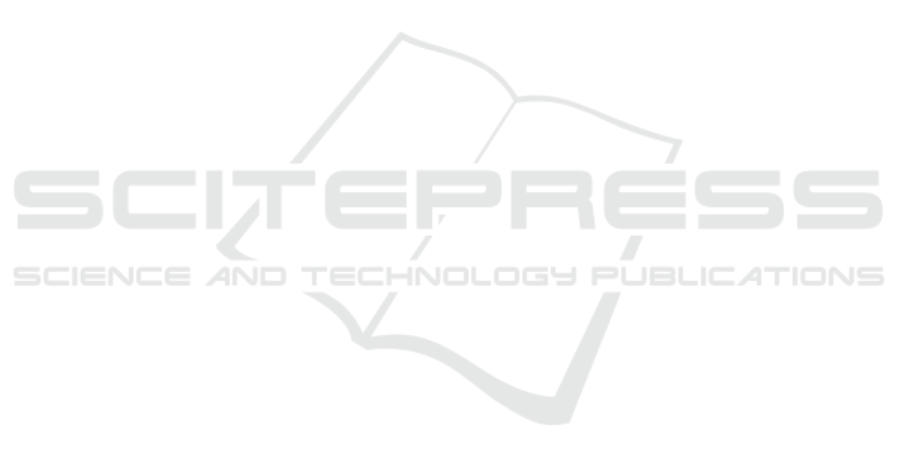
designed several networks (with a different number
of hidden layers) with the aim to investigate whether
increasing the number of hidden layers we are able
to obtain better performances. The best classification
performances are obtained with the models trained us-
ing the MLP algorithm with 1 (MLP 1 in Table 4) and
3 hidden layers (MLP 3 in Table 4): the weighted pre-
cision obtained is 0.974 for both the classifiers, while
the recall is equal to 0.966 for the MLP 1 classifica-
tion and 0.965 for the MLP 3 one. The performances
dramatically decrease when are considered 5 hidden
layers.
4 CONCLUSIONS AND FUTURE
WORK
Nowadays the safety of cars and passengers relies
on the communication mechanism provided by the
CAN bus, a serial data communication to permits the
communication between the several components in-
side modern vehicles. In order to increase the safety
of modern cars in this paper we proposed a method
to identify attacks targeting the CAN bus exploiting
deep learning algorithms. We demonstrated the ef-
fectiveness of the proposed method evaluating a real-
world dataset containing CAN messages related to
four attacks (i.e., dos, fuzzy, rpm and gear) messages
and normal messages gathered from a real vehicle.
We obtained the best results using deep learning net-
works trained with the MLP classification algorithm
with 1 and 3 hidden layers, reaching a weighted preci-
sion equal to 0.974 and weighted recall equal to 0.966
for the MLP classification with one hidden layer and
equal to 0.965 for the MLP classification with three
hidden layers.
As future work we plan to evaluate the proposed
method to a more extensive set of attacks, to ver-
ify the effectiveness of the proposed method in the
identification of a more widespread set of attacks.
Furthermore, we will investigate the adoption of for-
mal methods with the aim to localize the attack CAN
packets with the aim to prevent the malicious injec-
tion. Another line consists in integrating the actual
framework with emerging big data trends (e.g., (Cuz-
zocrea et al., 2009; Cuzzocrea, 2006; Cuzzocrea et al.,
2013)).
ACKNOWLEDGMENTS
This work has been partially supported by H2020
EU-funded projects NeCS and C3ISP and EIT-Digital
Project HII and PRIN “Governing Adaptive and Un-
planned Systems of Systems” and the EU project Cy-
berSure 734815.
REFERENCES
Al-Kahtani, M. S. (2012). Survey on security attacks in ve-
hicular ad hoc networks (vanets). In Signal Process-
ing and Communication Systems (ICSPCS), 2012 6th
International Conference on, pages 1–9. IEEE.
Baccouche, M., Mamalet, F., Wolf, C., Garcia, C., and
Baskurt, A. (2011). Sequential deep learning for hu-
man action recognition. In International Workshop
on Human Behavior Understanding, pages 29–39.
Springer.
Bernardi, M. L., Cimitile, M., Martinelli, F., and Mercaldo,
F. (2018). Driver and path detection through time-
series classification. Journal of Advanced Transporta-
tion, 2018.
Cuzzocrea, A. (2006). Accuracy control in compressed
multidimensional data cubes for quality of answer-
based OLAP tools. In 18th International Conference
on Scientific and Statistical Database Management,
SSDBM 2006, 3-5 July 2006, Vienna, Austria, Pro-
ceedings, pages 301–310.
Cuzzocrea, A., Fortino, G., and Rana, O. F. (2013). Man-
aging data and processes in cloud-enabled large-scale
sensor networks: State-of-the-art and future research
directions. In 13th IEEE/ACM International Sympo-
sium on Cluster, Cloud, and Grid Computing, CCGrid
2013, Delft, Netherlands, May 13-16, 2013, pages
583–588.
Cuzzocrea, A., Furfaro, F., and Sacc
`
a, D. (2009). En-
abling OLAP in mobile environments via intelligent
data cube compression techniques. J. Intell. Inf. Syst.,
33(2):95–143.
Ding, L., Fang, W., Luo, H., Love, P. E., Zhong, B., and
Ouyang, X. (2018). A deep hybrid learning model to
detect unsafe behavior: Integrating convolution neural
networks and long short-term memory. Automation in
Construction, 86:118–124.
Kwak, B. I., Woo, J., and Kim, H. K. (2016). Know your
master: Driver profiling-based anti-theft method. In
PST 2016.
Martinelli, F., Mercaldo, F., Nardone, V., Orlando, A., and
Santone, A. (2018). Whos driving my car? a machine
learning based approach to driver identification. In
ICISSP.
Martinelli, F., Mercaldo, F., Nardone, V., and Santone, A.
(2017). Car hacking identification through fuzzy logic
algorithms. In Fuzzy Systems (FUZZ-IEEE), 2017
IEEE International Conference on, pages 1–7. IEEE.
Samara, G., Al-Salihy, W. A., and Sures, R. (2010). Se-
curity issues and challenges of vehicular ad hoc net-
works (vanet). In New Trends in Information Science
and Service Science (NISS), 2010 4th International
Conference on, pages 393–398. IEEE.
SECRYPT 2018 - International Conference on Security and Cryptography
320