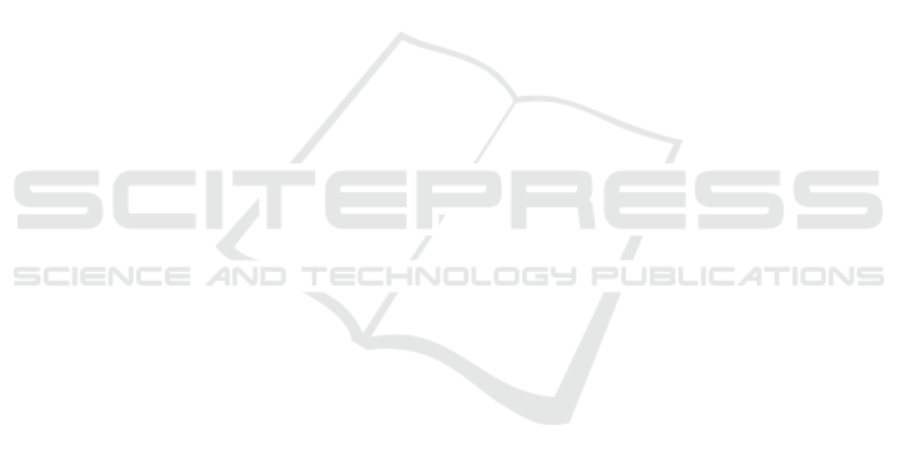
3 observations by the attacker. If l = 200 concepts by
pictures, 2000 concepts are required to have a good
security up to N = 3 observations and ≈ 10
280
pic-
tures are possible. If l = 100 concepts by pictures,
1000 concepts are required to have a good security
up to N = 2 observations and ≈ 10
140
pictures are
possible. This represents many possible pictures with
many concepts in each picture just to resist to only 2
or 3 observations.
Moreover, human limits and hardware limits are
not taken into account in this paper. But they repre-
sent important limitation in the implementation. Gen-
erating pictures on the fly would be an interesting so-
lution, as it would solve many problems of HSA. An
attacker won’t be able to know the picture database
since it does not exist and the device won’t have to
store all pictures. So she would need detect concepts
in pictures. To our knowledge the best methods in-
volve machine learning algorithms. That requires a
characterisation step, with a numerous data samples.
Another question is how to generate a picture with
100 concepts such that a human is able to retrieve her
concept in this picture?
In practice, it is often better to have no security
at all and be fully aware of it rather than having a
false feeling of protection relying on weak security.
An HSA user is less alert than a standard PIN code
user against shoulder surfing attacks. Since they feel
protected against this kind of attacks, they are more
careless in hiding their authentication. Yet security
breaks down quickly (3 observations), so they should
not relax their attention.
REFERENCES
Aviv, A. J., Sapp, B., Blaze, M., and Smith, J. M. (2012).
Practicality of accelerometer side channels on smart-
phones. In Proceedings of the 28th Annual Computer
Security Applications Conference, ACSAC ’12, pages
41–50, New York, NY, USA. ACM.
Brostoff, S. and Sasse, M. A. (2000). Are passfaces more
usable than passwords? a field trial investigation. In
People and Computers XIVUsability or Else!, pages
405–424. Springer.
Dhamija, R., Perrig, A., et al. (2000). Deja vu-a user study:
Using images for authentication. In USENIX Security
Symposium, volume 9, pages 4–4.
Enck, W., Gilbert, P., Chun, B.-G., Cox, L. P., Jung, J., Mc-
Daniel, P., and Sheth, A. N. (2010). Taintdroid: An
information-flow tracking system for realtime privacy
monitoring on smartphones. In Proceedings of the 9th
USENIX Conference on Operating Systems Design
and Implementation, OSDI’10, pages 1–6, Berkeley,
CA, USA. USENIX Association.
Felt, A. P., Finifter, M., Chin, E., Hanna, S., and Wagner, D.
(2011). A survey of mobile malware in the wild. In
Proceedings of the 1st ACM Workshop on Security and
Privacy in Smartphones and Mobile Devices, SPSM
’11, pages 3–14, New York, NY, USA. ACM.
Hayashi, E., Dhamija, R., Christin, N., and Perrig, A.
(2008). Use your illusion: secure authentication us-
able anywhere. In Proceedings of the 4th symposium
on Usable privacy and security, pages 35–45. ACM.
Jermyn, I., Mayer, A., Monrose, F., Reiter, M. K., and Ru-
bin, A. D. (1999). The design and analysis of graphi-
cal passwords. USENIX Association.
Owusu, E., Han, J., Das, S., Perrig, A., and Zhang, J.
(2012). Accessory: Password inference using ac-
celerometers on smartphones. In Proceedings of
the Twelfth Workshop on Mobile Computing Systems
& Applications, HotMobile ’12, pages 9:1–9:6,
New York, NY, USA. ACM.
Renaud, K., Mayer, P., Volkamer, M., and Maguire, J.
(2013). Are graphical authentication mechanisms as
strong as passwords? In Computer Science and In-
formation Systems (FedCSIS), 2013 Federated Con-
ference on, pages 837–844. IEEE.
Salembier, P., Zouinar, M., H
´
eron, R., Mathias, C., Lorant,
G., and Wary, J.-P. (2016). Experimental studies of
a graphical authentication system based on semantic
categorisation. In Actes de la 28i
`
eme conf
´
erence fran-
cophone sur l’Interaction Homme-Machine, pages
134–143. ACM.
Simon, L. and Anderson, R. (2013). Pin skimmer: Inferring
pins through the camera and microphone. In Proceed-
ings of the Third ACM Workshop on Security and Pri-
vacy in Smartphones & Mobile Devices, SPSM
’13, pages 67–78, New York, NY, USA. ACM.
Stavrou, A., Benameur, A., and Johnson, R. (2017). All
your sms and contacts belong to adups and others.
Proceedings of the Black Hat USA.
Suo, X., Zhu, Y., and Owen, G. S. (2005). Graphical pass-
words: A survey. In Computer security applications
conference, 21st annual, pages 10–pp. IEEE.
Tao, H. and Adams, C. (2008). Pass-go: A proposal to im-
prove the usability of graphical passwords. IJ Network
Security, 7(2):273–292.
Wiedenbeck, S., Waters, J., Birget, J.-C., Brodskiy, A., and
Memon, N. (2005). Passpoints: Design and longitu-
dinal evaluation of a graphical password system. In-
ternational journal of human-computer studies, 63(1-
2):102–127.
Xu, Z., Bai, K., and Zhu, S. (2012). Taplogger: Infer-
ring user inputs on smartphone touchscreens using on-
board motion sensors. In Proceedings of the Fifth
ACM Conference on Security and Privacy in Wireless
and Mobile Networks, WISEC ’12, pages 113–124,
New York, NY, USA. ACM.
Yue, Q., Ling, Z., Fu, X., Liu, B., Yu, W., and Zhao, W.
(2014). My google glass sees your passwords! Pro-
ceedings of the Black Hat USA.
Zouinar, M., Mathias, C., Lorant, G., and Wary, J.-
P. (2016). Evaluation ergonomique d’un syst
`
eme
d’authentification graphique.
Theoretical Security Evaluation of the Human Semantic Authentication Protocol
339