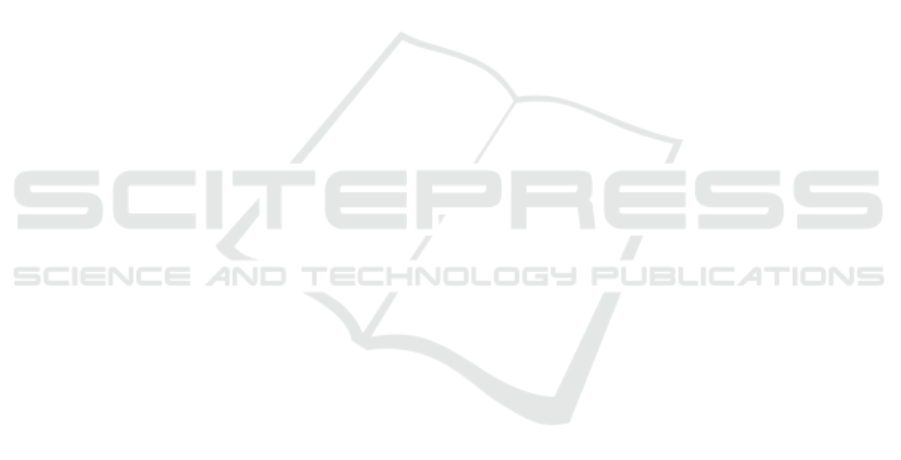
6 CONCLUSIONS
In this paper, we examine a missile attack scenario
combined with a cyber-attack scenario. To do that,
we solve the SIR model using an approximate
solution which reproduces all of the characteristics
of the problem such as the asymmetry, the trends in
time evolution of the parameters
,
and
as well
as their long term behaviours.
It could also be argued that the asymmetric
approximation is in itself an epidemic model in the
same way that the SIR model is an epidemic model.
They are both anchored on similar assumptions. And
they both generate similar results.
We show that the missile defence effectiveness
of the blue force can be critically affected when the
red force launches a cyber-attack at the same time as
a missile attack.
To our knowledge, the degradations of a missile
defence system due to a cyber-attack have not been
explicitly modelled. In the future, we would like to
examine closely how command and control systems
are affected by a cyber-attack. To do that, we will
investigate in depth the complexity of command and
control systems and the nature of cyber-attacks.
REFERENCES
Bailey, N.T.J., 1975. The mathematical theory of
infectious diseases and its applications, Charles
Griffin and Company LTD, 2nd edition, pp. 39-42.
Cranford K.. 2004. Battle Area Region Threatened Model
(BART). NORAD-USNORTHCOM/AN (North
American Aerospace Defense Command – United
States Northern Command/Center for Aerospace
Analysis) Version 5.3.4x. and the CheckThreats
subroutine.
Harko, T., Lobo, F.S.N., and Mak, M.K., 2014. ‘Exact
analytical solutions of the Susceptible-Infected-
Recovered (SIR) epidemic model and of the SIR
model with equal death and birth rates’, Applied
Mathematics and Computation, 236, pp. 184-194.
Hethcote, H., 2000. ‘The mathematics of infectious
diseases’, SIAM Review, Vol. 42, No. 4, pp. 599-653.
Keeling, M.J. and Rohani, P., 2007. Modeling Infectious
Diseases in Humans and Animals, Princeton
University Press, p. 4.
Mathematica 2011. Wolfram Research Inc.
Menq J.-Y., Tuan P.-C. and Liu T.-S., 2007. ‘Discrete
Markov Ballistic Missile Defense System Modeling’,
European Journal of Operational Research, 178, pp.
560–578.
Morris-King, J. and Cam, H., 2015. ‘Ecology-inspired
cyber risk model for propagation of vulnerability
exploitation in tactical edge’, Proceedings of the IEEE
2015 Military Communications Conference
MILCOM'2015, pp. 336-341.
Nguyen B.U., Smith P.A. and Nguyen D., 1997. ‘An
Engagement Model to Optimize Defense Against a
Multiple Attack Assuming Perfect Kill Assessment’,
Naval Research Logistics, 44, pp. 687-697.
Nguyen, B.U., 2017. ‘Modelling cyber vulnerabilities
using epidemic models’, 7th international conference
on simulation and modelling methodologies,
technologies and applications.
Nguyen, B. and Miah, S., 2015. ‘Comparison of metrics
for missile defence between perfect coordination and
no coordination’, DRDC Scientific Report (SR)
DRDC-RDDC-2015-R228.
Press, W.H., Flannery, B.P., Teukolsky, S.A. and
Vetterling W.T., 1992. Numerical recipes in Fortran
77 (The Art of Scientific Computing). Cambridge
University Press, 2nd edition, pp. 343-347.
Smith? R., 2008. Modelling disease ecology with
mathematics, American Institute of Mathematical
Sciences, pp. 14-30.
Soland, R. M., 1987. ‘Optimal Terminal Defense Tactics
when Several Sequential Engagements Are Possible’,
Operations Research, 35, pp. 537-542.
Zou, C.C., Gong, W., and Towsley, D., 2002. Code red
worm propagation modeling and analysis,
Proceedings of the 9th ACM conference on Computer
and communications security, pp. 138-147.
A Simultaneous Cyber-attack and a Missile Attack
499