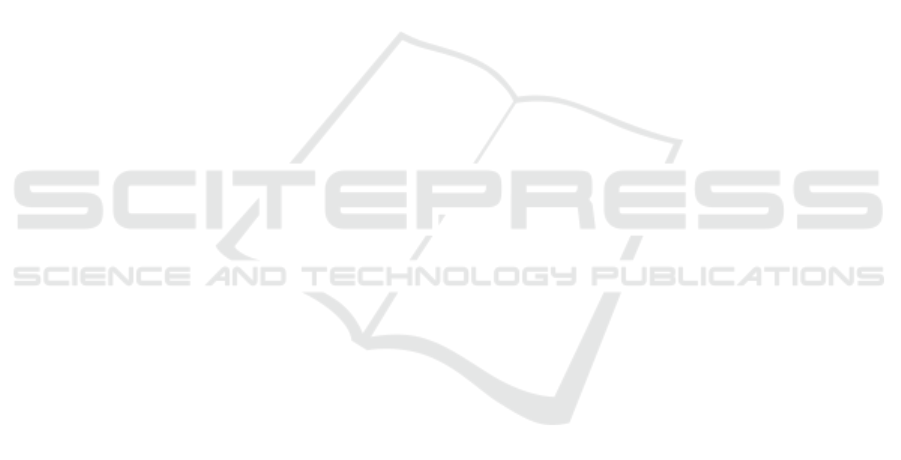
REFERENCES
Amato, G., Carrara, F., Falchi, F., Gennaro, C., Meghini, C.,
and Vairo, C. (2017). Deep learning for decentralized
parking lot occupancy detection. Expert Systems with
Applications, 72(15):327–334.
Arteta, C., Lempitsky, V., Noble, J. A., and Zisserman, A.
(2014). Interactive Object Counting. In European
Conference on Computer Vision, pages 1–15.
Arteta, C., Lempitsky, V., and Zisserman, A. (2016). Coun-
ting in the wild. In European Conference on Computer
Vision, pages 483–498.
Battiato, S., Farinella, G. M., Furnari, A., Puglisi, G., Sni-
jders, A., and Spiekstra, J. (2015). An integrated sy-
stem for vehicle tracking and classification. Expert
Systems with Applications, 42(21):7263–7275.
Battiato, S., Farinella, G. M., Gallo, G., and Giudice, O.
(2018). On-board monitoring system for road traffic
safety analysis. Computers in Industry, 98:208 – 217.
Chan, A. B., Liang, Z. S.-J., and Vasconcelos, N. (2008).
Privacy preserving crowd monitoring: Counting pe-
ople without people models or tracking. In Confe-
rence on Computer Vision and Pattern Recognition,
pages 1–7. IEEE.
Chen, S., Fern, A., and Todorovic, S. (2015). Person count
localization in videos from noisy foreground and de-
tections. In Conference on Computer Vision and Pat-
tern Recognition, pages 1364–1372. IEEE.
Davis, H. and P
´
erusse, R. (1988). Numerical competence in
animals: Definitional issues, current evidence, and a
new research agenda. Behavioral and Brain Sciences,
11(4):561–579.
De Almeida, P. R., Oliveira, L. S., Britto, A. S., Silva, E. J.,
and Koerich, A. L. (2015). PKLot-A robust dataset
for parking lot classification. Expert Systems with Ap-
plications, 42(11):4937–4949.
Di Mauro, D., Battiato, S., Patan
`
e, G., Leotta, M., Maio,
D., and Farinella, G. M. (2016). Learning approaches
for parking lots classification. In International Con-
ference on Advanced Concepts for Intelligent Vision
Systems, pages 410–418. Springer.
Eiselt, A.-K. and Nieder, A. (2013). Representation of ab-
stract quantitative rules applied to spatial and numeri-
cal magnitudes in primate prefrontal cortex. The Jour-
nal of neuroscience: the official journal of the Society
for Neuroscience, 33(17):7526–34.
Everingham, M., Van Gool, L., Williams, C. K., Winn, J.,
and Zisserman, A. (2010). The pascal visual object
classes (voc) challenge. International journal of com-
puter vision, 88(2):303–338.
Ferrari, A., Lombardi, S., and Signoroni, A. (2017). Bacte-
rial colony counting with convolutional neural net-
works in digital microbiology imaging. Pattern Re-
cognition, 61:629–640.
Fiaschi, L., Koethe, U., Nair, R., and Hamprecht, F. A.
(2012). Learning to count with regression forest and
structured labels. In International Conference on Pat-
tern Recognition, pages 2685–2688.
Google (2018). Google Maps. https://www.google.it/maps/
@37.5264537,15.0741852,230m/data=!3m1!1e3?hl=en.
[Online; accessed 12-March-2017].
Jia, Y., Shelhamer, E., Donahue, J., Karayev, S., Long, J.,
Girshick, R., Guadarrama, S., and Darrell, T. (2014).
Caffe: Convolutional architecture for fast feature em-
bedding. arXiv preprint arXiv:1408.5093.
Kaufman, E. L., Lord, M. W., Reese, T. W., and Volkmann,
J. (1949). The discrimination of visual number. The
American journal of psychology, 62(4):498–525.
Lempitsky, V. and Zisserman, A. (2010). Learning to Count
Objects in Images. In Advances in Neural Information
Processing Systems, pages 1324–1332.
Li, M., Zhang, Z., Huang, K., and Tan, T. (2008). Estima-
ting the number of people in crowded scenes by MID
based foreground segmentation and head-shoulder de-
tection. In International Conference on Pattern Re-
cognition, pages 1–4.
Loy, C. C., Chen, K., Gong, S., and Xiang, T. (2013).
Crowd Counting and Profiling: Methodology and
Evaluation, pages 347–382. Springer, New York, NY.
Ng, L. L. and Chua, H. S. (2012). Vision-based activities
recognition by trajectory analysis for parking lot sur-
veillance. In International Conference on Circuits and
Systems, pages 137–142.
Nieder, A. (2016). The neuronal code for number. Nature
Reviews Neuroscience, 17(6):366–382.
Rabaud, V. and Belongie, S. (2006). Counting Crowded
Moving Objects. In Conference on Computer Vision
and Pattern Recognition, pages 705–711. IEEE.
Raymond, J.-F. (2001). Traffic analysis: Protocols, attacks,
design issues, and open problems. In Designing Pri-
vacy Enhancing Technologies, pages 10–29.
Ren, S., He, K., Girshick, R., and Sun, J. (2015). Faster R-
CNN: Towards real-time object detection with region
proposal networks. In Advances in neural information
processing systems, pages 91–99.
Russakovsky, O., Deng, J., Su, H., Krause, J., Satheesh,
S., Ma, S., Huang, Z., Karpathy, A., Khosla, A.,
Bernstein, M., Berg, A. C., and Fei-Fei, L. (2015).
ImageNet Large Scale Visual Recognition Challenge.
International Journal of Computer Vision (IJCV),
115(3):211–252.
Simonyan, K. and Zisserman, A. (2014). Very deep con-
volutional networks for large-scale image recognition.
arXiv preprint arXiv:1409.1556.
United Nations (2008). World urbanization prospects: The
2007 revision. United Nations Publications.
Wu, Q., Huang, C., Wang, S.-y., Chiu, W.-c., and Chen, T.
(2007). Robust parking space detection considering
inter-space correlation. In International Conference
on Multimedia and Expo, pages 659–662. IEEE.
Zhang, C., Li, H., Wang, X., and Yang, X. (2015). Cross-
scene crowd counting via deep convolutional neural
networks. In Conference on Computer Vision and Pat-
tern Recognition, pages 833–841. IEEE.
SIGMAP 2018 - International Conference on Signal Processing and Multimedia Applications
336