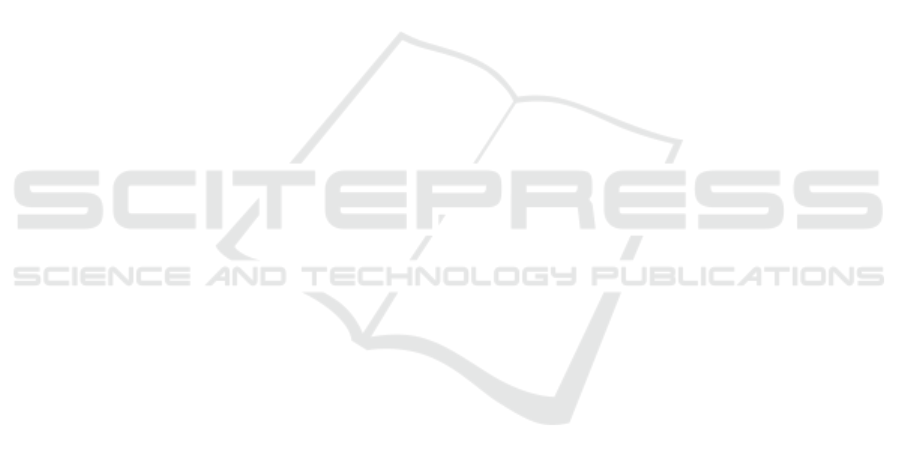
on Computational Color Imaging, pages 105–121.
Springer.
Comaniciu, D. and Meer, P. (2002). Mean shift: A robust
approach toward feature space analysis. Pattern Anal-
ysis and Machine Intelligence, IEEE Transactions on,
24(5):603–619.
Corchs, S., Gasparini, F., and Schettini, R. (2014). No ref-
erence image quality classification for jpeg-distorted
images. Digital Signal Processing, 30:86–100.
Corchs, S. E., Ciocca, G., Bricolo, E., and Gasparini,
F. (2016). Predicting complexity perception of real
world images. PloS one, 11(6):e0157986.
Dan-Glauser, E. S. and Scherer, K. R. (2011). The geneva
affective picture database (gaped): a new 730-picture
database focusing on valence and normative signifi-
cance. Behavior research methods, 43(2):468.
Ghadiyaram, D. and Bovik, A. C. (2015). Feature maps
driven no-reference image quality prediction of au-
thentically distorted images. In Human Vision and
Electronic Imaging XX, volume 9394, page 93940J.
International Society for Optics and Photonics.
Ghadiyaram, D. and Bovik, A. C. (2016). Massive online
crowdsourced study of subjective and objective pic-
ture quality. IEEE Transactions on Image Processing,
25(1):372–387.
Gu, K., Zhai, G., Yang, X., and Zhang, W. (2015). Using
free energy principle for blind image quality assess-
ment. IEEE Transactions on Multimedia, 17(1):50–
63.
Hasler, D. and Suesstrunk, S. E. (2003). Measuring col-
orfulness in natural images. In Electronic Imaging
2003, pages 87–95. International Society for Optics
and Photonics.
Junior, O. L., Delgado, D., Gonc¸alves, V., and Nunes, U.
(2009). Trainable classifier-fusion schemes: An ap-
plication to pedestrian detection. In Intelligent Trans-
portation Systems, 2009. ITSC’09. 12th International
IEEE Conference on, pages 1–6. IEEE.
Larson, E. C. and Chandler, D. M. (2010). Most appar-
ent distortion: full-reference image quality assessment
and the role of strategy. Journal of Electronic Imag-
ing, 19(1):011006–011006.
Liu, L., Hua, Y., Zhao, Q., Huang, H., and Bovik, A. C.
(2016). Blind image quality assessment by relative
gradient statistics and adaboosting neural network.
Signal Processing: Image Communication, 40:1–15.
Mack, M. and Oliva, A. (2004). Computational estimation
of visual complexity. In the 12th Annual Object, Per-
ception, Attention, and Memory Conference.
Minhas, R., Mohammed, A. A., Wu, Q. J., and Sid-Ahmed,
M. A. (2009). 3d shape from focus and depth map
computation using steerable filters. In International
Conference Image Analysis and Recognition, pages
573–583. Springer.
Mittal, A., Moorthy, A. K., and Bovik, A. C. (2012).
No-reference image quality assessment in the spatial
domain. IEEE Transactions on Image Processing,
21(12):4695–4708.
Mittal, A., Soundararajan, R., and Bovik, A. C. (2013).
Making a completely blind image quality analyzer.
IEEE Signal Processing Letters, 20(3):209–212.
Moorthy, A. K. and Bovik, A. C. (2010). A two-step
framework for constructing blind image quality in-
dices. IEEE Signal processing letters, 17(5):513–516.
Muijs, R. and Kirenko, I. (2005). A no-reference block-
ing artifact measure for adaptive video processing. In
Proceedings of the 13th European Signal Processing
Conference 2005.
Ojala, T., Pietik
¨
ainen, M., and Harwood, D. (1996). A com-
parative study of texture measures with classification
based on featured distributions. Pattern recognition,
29(1):51–59.
Pan, F., Lin, X.and Rahardja, S., Lin, W., Ong, E., Yao, S.,
Lu, Z., and Yang, X. (2004). Locally-adaptive algo-
rithm for measuring blocking artifacts in images and
videos. In Proceedings of the International Sympo-
sium on Circuits and Systems, volume 3, pages 925–
928. IEEE.
Rosenholtz, R., Li, Y., and Nakano, L. (2007). Measuring
visual clutter. Journal of vision, 7(2):17–17.
Saad, M. A., Bovik, A. C., and Charrier, C. (2010). A dct
statistics-based blind image quality index. IEEE Sig-
nal Processing Letters, 17(6):583–586.
Schettini, R., Gasparini, F., Corchs, S., Marini, F., Capra,
A., and Castorina, A. (2010). Contrast image cor-
rection method. Journal of Electronic Imaging,
19(2):023005–023005.
Sheikh, H. R., Sabir, M. F., and Bovik, A. C. (2006). A sta-
tistical evaluation of recent full reference image qual-
ity assessment algorithms. IEEE Transactions on im-
age processing, 15(11):3440–3451.
Siahaan, E., Hanjalic, A., and Redi, J. A. (2018). Semantic-
aware blind image quality assessment. Signal Pro-
cessing: Image Communication, 60:237–252.
Tamura, H., Mori, S., and Yamawaki, T. (1978). Textu-
ral features corresponding to visual perception. Sys-
tems, Man and Cybernetics, IEEE Transactions on,
8(6):460–473.
Triantaphillidou, S., Allen, E., and Jacobson, R. (2007). Im-
age quality comparison between jpeg and jpeg2000.
ii. scene dependency, scene analysis, and classifica-
tion. Journal of Imaging Science and Technology,
51(3):259–270.
Van Der Linde, I. and Doe, R. M. (2012). Influence of affec-
tive image content on subjective quality assessment.
JOSA A, 29(9):1948–1955.
Wang, Z., Bovik, A. C., and Evans, B. L. (2000). Blind
measurement of blocking artifacts in images. In Proc.
International Conference on Image Processing, vol-
ume 3, pages 981–984. IEEE.
Wang, Z., Sheikh, H., and Bovik, A. C. (2002). No-
reference perceptual quality assessment of jpeg com-
pressed images. In Proc. International Conference on
Image Processing, volume 1, pages 477–480. IEEE.
Wu, H. and Yuen, M. (1997). A generalized block-edge im-
pairment metric for video coding. IEEE Signal Pro-
cessing Letters, 4:317–320.
Quality Assessment of JPEG-distorted Face Images: Influence of Affective Content
393