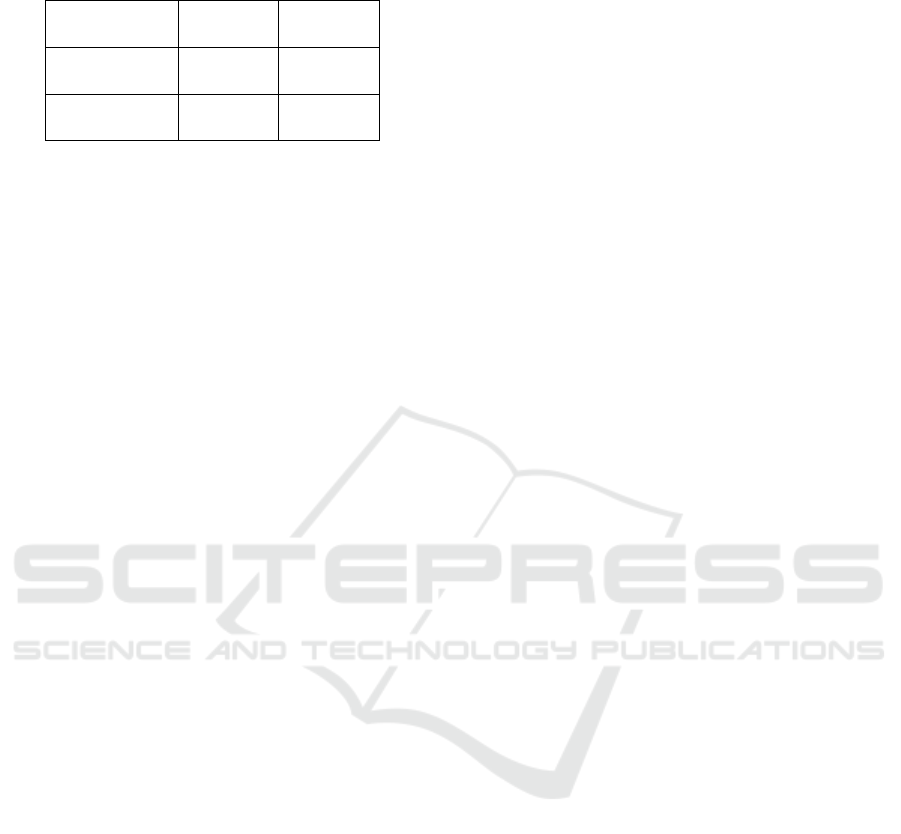
Table 4: Comparison of present paper with (Seehapoch and
Wongthanavasu, 2013).
Features
Accuracy
EmoDB
Accuracy
KeioESD
Seehapoch et
al., 2013
78.04% 89.23%
Present paper
method
71.23% 83.33%
explicitly stated.
The accuracy scores obtained are really close to
those observed in (Seehapoch and Wongthanavasu,
2013). The chosen features allow to perform a good
classification, especially when it comes to determine
if there is stress or not.
6 CONCLUSION
The main goal of this work, which was to detect stress
through speech analysis, has been completed on three
different datasets: i) EmoDB (German), ii) KeioESD
(Japanese) and iii) RAVDESS (English). The use
of the mean energy, the mean intensity and MFCCs
proved to be good features for speech analysis, espe-
cially the MFCCs. The best way to use these MFC co-
efficients is the computation of the mean and the stan-
dard deviation of each of them, instead of using them
as a single feature which can lead to very large fea-
ture sets. Neural Networks show the best results even
if Support Vector Machines are really close. Both al-
gorithms perform really well for such classification
problem.
To conclude, it is interesting to note that the length
of audio files does not have a big impact. The results
for the EmoDB and KeioESD datasets are really close
even if the audio length is not the same (about 3 sec-
onds for the first one and about half a second for the
latter).
The results obtained were satisfying but there is
however room for improvement. To increase the accu-
racy scores, features such as formants, MFCCs deltas
or speech rate could be added to the feature set and
thus used for classification. More time could also be
spent on algorithms optimization. A set of parame-
ters with a range of value have been chosen but this
range could be increased and more parameters could
be used for a better tuning. To finish, acquiring bet-
ter datasets with much more data would be ideal. The
fact that the data is spoken by actors does not exactly
reflect what he is feeling. Because of the complexity
of emotions and the effects behind, having data from
a lot of different people in real life situations would
probably give interesting results.
ACKNOWLEDGEMENTS
We would like to thank the AIR @ en-japan Company
who made this research possible and the precious ad-
vice given by the AIR members Salah Hawila, Maik
Vlcek and Roy Tseng.
REFERENCES
Keio university japanese emotional speech database (keio-
esd). http://research.nii.ac.jp/src/en/Keio-ESD.html.
Accessed: 2018-03-29.
Banse, R. and Scherer, K. R. (1996). Acoustic profiles in
vocal emotion expression. Journal of personality and
social psychology, 70(3):614.
Boersma, P. and Weenink, D. (2006). Praat manual. Ams-
terdam: University of Amsterdam, Phonetic Sciences
Department.
Burkhardt, F., Paeschke, A., Rolfes, M., Sendlmeier, W. F.,
and Weiss, B. (2005). A database of german emotional
speech. In Ninth European Conference on Speech
Communication and Technology.
Johnstone, T. (2017). The effect of emotion on voice pro-
duction and speech acoustics.
Lanjewar, R. B., Mathurkar, S., and Patel, N. (2015). Imple-
mentation and comparison of speech emotion recogni-
tion system using gaussian mixture model (gmm) and
k-nearest neighbor (k-nn) techniques. Procedia Com-
puter Science, 49:50–57.
Livingstone, S. R., Peck, K., and Russo, F. A. (2012).
Ravdess: The ryerson audio-visual database of emo-
tional speech and song. In Annual meeting of the
canadian society for brain, behaviour and cognitive
science, pages 205–211.
Lyons, J. (2015). Mel frequency cepstral coefficient (mfcc)
tutorial. Practical Cryptography.
Mori, S., Moriyama, T., and Ozawa, S. (2006). Emo-
tional speech synthesis using subspace constraints in
prosody. In Multimedia and Expo, 2006 IEEE Inter-
national Conference on, pages 1093–1096. IEEE.
Moriyama, T., Mori, S., and Ozawa, S. (2009). A synthe-
sis method of emotional speech using subspace con-
straints in prosody. Journal of Information Processing
Society of Japan, 50(3):1181–1191.
Seehapoch, T. and Wongthanavasu, S. (2013). Speech emo-
tion recognition using support vector machines. In
Knowledge and Smart Technology (KST), 2013 5th In-
ternational Conference on, pages 86–91. IEEE.
SIGMAP 2018 - International Conference on Signal Processing and Multimedia Applications
398