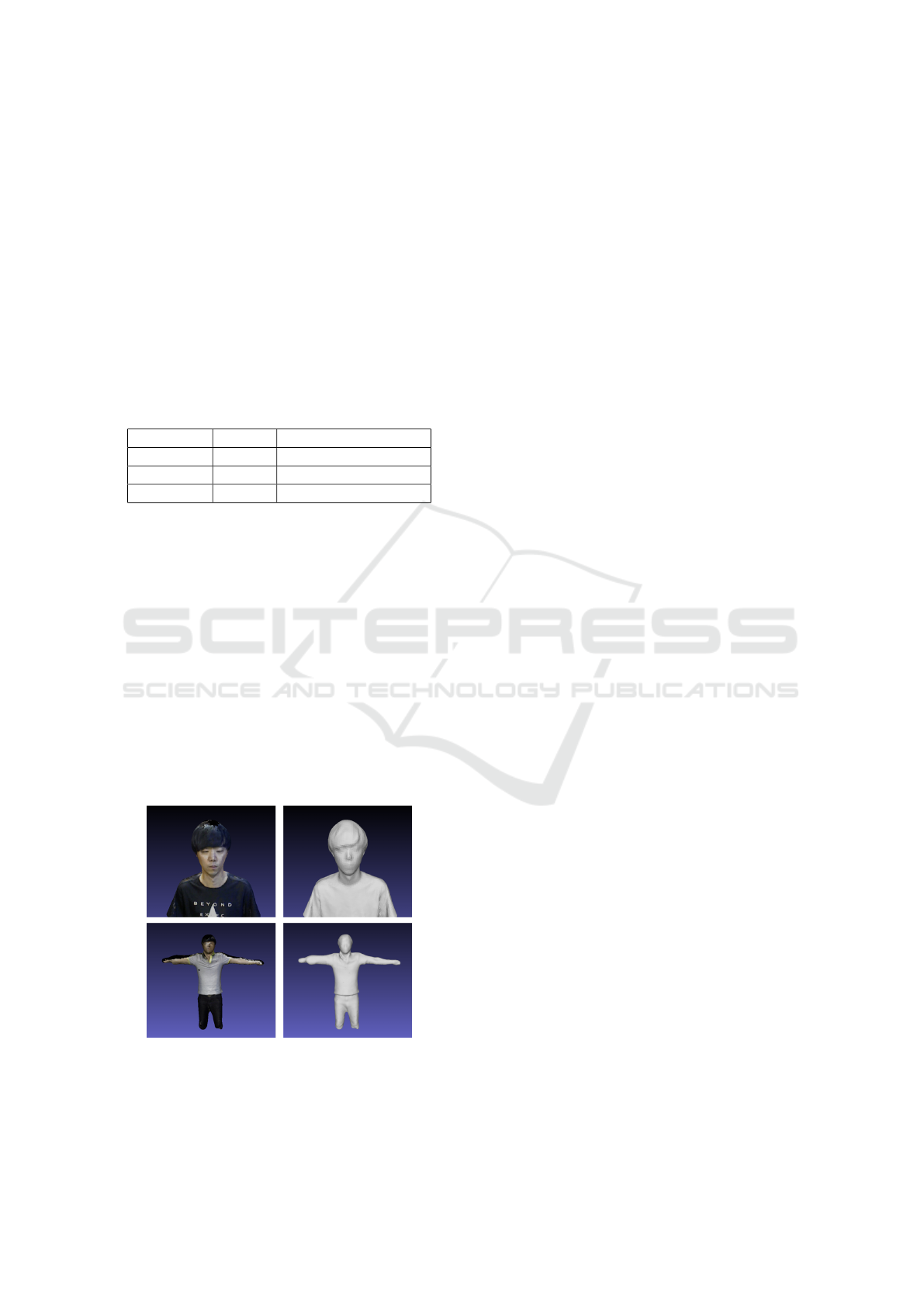
tial pose estimation step in real-time is 512x512x512,
and its area is determined experimentally between 1.2
∼ 1.8m in width and height. We obtained depth and
color data by moving the sensor in 360
◦
around the
model. Table.1 summarizes the nearest distance er-
ror between neighbor points for three different data
sets. Figure 5 shows the experiment model we used
and pose estimation results between our proposed and
ICP method. We can confirm that the points are
more precisely aligned in the mesh model created us-
ing our method compared to ICP method. Figure 6
shows reconstruction results and their texture map-
pings done using color information corresponding to
each keyframe in Data 1 data set.
Table 1: The result of Pose estimation refinement.
Data name Frames Nearest distance error
Data 1 773 0.73
Data 2 972 0.81
Data 3 704 0.89
5 CONCLUSIONS
In this paper, we described an accurate real time 3D
pose estimation and refinement method using depth
and color information of a single hand-held sensor.
We first described about initial pose estimation of the
sensor at real time. We selected keyframes and esti-
mated their pose more robustly using Point-to-Plane
ICP algorithm. The accuracy of the estimated pose is
evaluated through experiment results such as 3D mesh
modelling and texture mapping. As future work, we
are planning to improve pose estimation and recon-
struction results by implementing non-rigid model
transformation techniques.
Figure 6: Keyframe pose estimation using 3D registra-
tion between subvolumes. Generated texture mapping
model(left) and mesh model(right).
ACKNOWLEDGEMENTS
This work was supported by ’The Cross-Ministry
Giga KOREA Project’ grant funded by the Korea gov-
ernment(MSIT) (No.GK17P0300, Real-time 4D re-
construction of dynamic objects for ultra-realistic ser-
vice).
REFERENCES
Chen, Y. and Medioni, G. (1992). Object modelling by reg-
istration of multiple range images. Image and vision
computing, 10(3):145–155.
Curless, B. and Levoy, M. (1996). A volumetric method for
building complex models from range images. In Pro-
ceedings of the 23rd annual conference on Computer
graphics and interactive techniques, pages 303–312.
ACM.
Dai, A., Chang, A. X., Savva, M., Halber, M., Funkhouser,
T., and Nießner, M. (2017a). Scannet: Richly-
annotated 3d reconstructions of indoor scenes. In
Proc. IEEE Conf. on Computer Vision and Pattern
Recognition (CVPR), volume 1.
Dai, A., Nießner, M., Zollh
¨
ofer, M., Izadi, S., and Theobalt,
C. (2017b). Bundlefusion: Real-time globally consis-
tent 3d reconstruction using on-the-fly surface rein-
tegration. ACM Transactions on Graphics (TOG),
36(3):24.
Maier, R., Sturm, J., and Cremers, D. (2014). Submap-
based bundle adjustment for 3d reconstruction from
rgb-d data. In German Conference on Pattern Recog-
nition, pages 54–65. Springer.
Newcombe, R. A., Izadi, S., Hilliges, O., Molyneaux, D.,
Kim, D., Davison, A. J., Kohi, P., Shotton, J., Hodges,
S., and Fitzgibbon, A. (2011). Kinectfusion: Real-
time dense surface mapping and tracking. pages 127–
136.
Nießner, M., Zollh
¨
ofer, M., Izadi, S., and Stamminger, M.
(2013). Real-time 3d reconstruction at scale using
voxel hashing. ACM Transactions on Graphics (ToG),
32(6):169.
Accurate Pose Estimation of a Hand-held RGBD Camera based on Sub-volume Matching for 3D Modeling
335