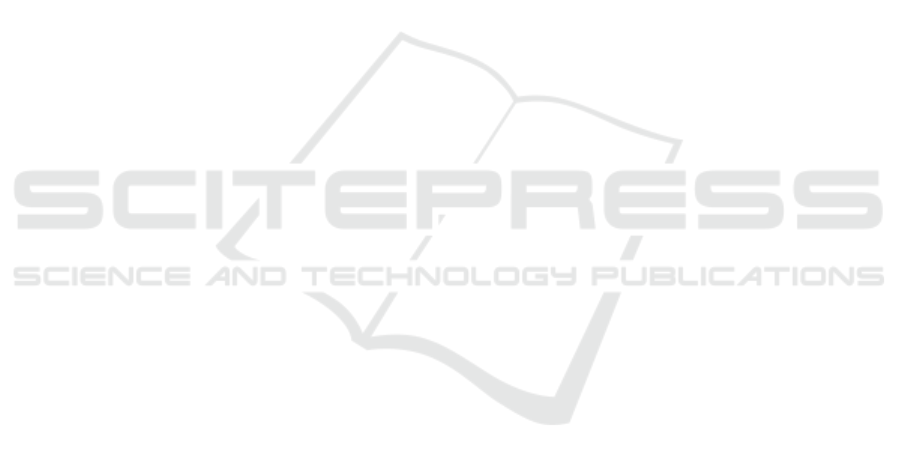
tonomous vehicles. In Intelligent Transportation
Systems-(ITSC), 2013 16th International IEEE Con-
ference on, pages 881–886. IEEE.
Comaniciu, D. and Meer, P. (2002). Mean shift: A robust
approach toward feature space analysis. IEEE Tran-
sactions on pattern analysis and machine intelligence,
24(5):603–619.
Douillard, B., Underwood, J., Vlaskine, V., Quadros, A.,
and Singh, S. (2014). A pipeline for the segmentation
and classification of 3d point clouds. In Experimental
robotics, pages 585–600. Springer.
Fritsch, J., Kuhnl, T., and Geiger, A. (2013). A new per-
formance measure and evaluation benchmark for road
detection algorithms. In Intelligent Transportation
Systems-(ITSC), 2013 16th International IEEE Con-
ference on, pages 1693–1700. IEEE.
Fukunaga, K. and Hostetler, L. (1975). The estimation of
the gradient of a density function, with applications in
pattern recognition. IEEE Transactions on informa-
tion theory, 21(1):32–40.
Geiger, A., Lenz, P., Stiller, C., and Urtasun, R. (2013).
Vision meets robotics: The kitti dataset. International
Journal of Robotics Research (IJRR).
Geiger, A., Lenz, P., and Urtasun, R. (2012). Are we re-
ady for autonomous driving? the kitti vision bench-
mark suite. In Computer Vision and Pattern Recogni-
tion (CVPR), 2012 IEEE Conference on, pages 3354–
3361. IEEE.
Gupta, S., Girshick, R., Arbel
´
aez, P., and Malik, J. (2014).
Learning rich features from rgb-d images for object
detection and segmentation. In European Conference
on Computer Vision, pages 345–360. Springer.
Habermann, D., Hata, A., Wolf, D., and Os
´
orio, F. S.
(2013). Artificial neural nets object recognition for 3d
point clouds. In Intelligent Systems (BRACIS), 2013
Brazilian Conference on, pages 101–106. IEEE.
Held, D., Guillory, D., Rebsamen, B., Thrun, S., and Sava-
rese, S. (2016). A probabilistic framework for real-
time 3d segmentation using spatial, temporal, and se-
mantic cues. In Proceedings of Robotics: Science and
Systems.
Himmelsbach, M., Luettel, T., and Wuensche, H.-J. (2009).
Real-time object classification in 3d point clouds
using point feature histograms. In Intelligent Robots
and Systems, 2009. IROS 2009. IEEE/RSJ Internatio-
nal Conference on, pages 994–1000. IEEE.
Himmelsbach, M. and Wuensche, H.-J. (2012). Tracking
and classification of arbitrary objects with bottom-
up/top-down detection. In Intelligent Vehicles Sym-
posium (IV), 2012 IEEE, pages 577–582. IEEE.
Kundu, A., Li, Y., Dellaert, F., Li, F., and Rehg, J. M.
(2014). Joint semantic segmentation and 3d recon-
struction from monocular video. In European Confe-
rence on Computer Vision, pages 703–718. Springer.
Lai, K., Bo, L., Ren, X., and Fox, D. (2012). Detection-
based object labeling in 3d scenes. In Robotics and
Automation (ICRA), 2012 IEEE International Confe-
rence on, pages 1330–1337. IEEE.
Lalonde, J.-F., Vandapel, N., Huber, D. F., and Hebert,
M. (2006). Natural terrain classification using three-
dimensional ladar data for ground robot mobility.
Journal of field robotics, 23(10):839–861.
Morton, P., Douillard, B., and Underwood, J. (2011). An
evaluation of dynamic object tracking with 3d lidar.
In Proc. of the Australasian Conference on Robotics
& Automation (ACRA).
Petrovskaya, A. and Thrun, S. (2009). Model based vehicle
detection and tracking for autonomous urban driving.
Autonomous Robots, 26(2-3):123–139.
Pitman, J. et al. (2002). Combinatorial stochastic processes.
Technical Report 621, Dept. Statistics, UC Berkeley,
2002. Lecture notes for St. Flour course.
Sch
¨
olkopf, B., Smola, A. J., Williamson, R. C., and Bartlett,
P. L. (2000). New support vector algorithms. Neural
computation, 12(5):1207–1245.
Sengupta, S., Greveson, E., Shahrokni, A., and Torr, P. H.
(2013). Urban 3d semantic modelling using stereo vi-
sion. In Robotics and Automation (ICRA), 2013 IEEE
International Conference on, pages 580–585. IEEE.
Serna, A. and Marcotegui, B. (2014). Detection, segmenta-
tion and classification of 3d urban objects using mat-
hematical morphology and supervised learning. IS-
PRS Journal of Photogrammetry and Remote Sensing,
93:243–255.
Spinello, L., Arras, K. O., Triebel, R., and Siegwart, R.
(2010). A layered approach to people detection in 3d
range data. In AAAI, volume 10, pages 1–1.
Teichman, A., Levinson, J., and Thrun, S. (2011). Towards
3d object recognition via classification of arbitrary ob-
ject tracks. In Robotics and Automation (ICRA), 2011
IEEE International Conference on, pages 4034–4041.
IEEE.
Tuncer, M. A. C¸ . and Schulz, D. (2015). Monte carlo ba-
sed distance dependent chinese restaurant process for
segmentation of 3d lidar data using motion and spatial
features. In Information Fusion (FUSION), 2015 18th
International Conference on, pages 112–118. IEEE.
Tuncer, M. A. C¸ . and Schulz, D. (2016a). Integrated ob-
ject segmentation and tracking for 3d lidar data. In
Proceedings of the 13th International Conference on
Informatics in Control, Automation and Robotics - Vo-
lume 2: ICINCO, pages 344–351.
Tuncer, M. A. C¸ . and Schulz, D. (2016b). Sequential dis-
tance dependent chinese restaurant processes for mo-
tion segmentation of 3d lidar data. In Information Fu-
sion (FUSION), 2016 19th International Conference
on, pages 758–765. IEEE.
Tuncer, M. A. . and Schulz, D. (2017). A hybrid method
using temporal and spatial information for 3d lidar
data segmentation. In Proceedings of the 14th Interna-
tional Conference on Informatics in Control, Automa-
tion and Robotics - Volume 2: ICINCO, pages 162–17.
Wang, D. Z., Posner, I., and Newman, P. (2012). What could
move? finding cars, pedestrians and bicyclists in 3d
laser data. In Robotics and Automation (ICRA), 2012
IEEE International Conference on, pages 4038–4044.
IEEE.
ICINCO 2018 - 15th International Conference on Informatics in Control, Automation and Robotics
332