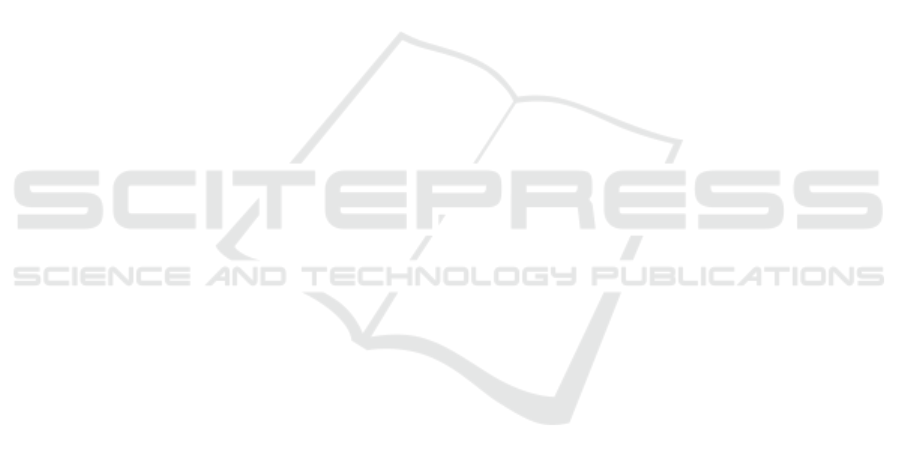
provement in classification performance. An analysis
of the salient regions extracted on images belonging
to the SAD
IT
dataset is reported in Figure 7. In this
Figure, original images (left column) are compared
with the corresponding salient regions (central col-
umn) and the resided version of the original images
(right column). The first two rows represent images
where the salient regions extracted significantly corre-
spond to the salient visual content. Instead for images
with a high dominance of text (fourth row) the salient
region could not be related to the visual content, ex-
tracting prevalently the text. Finally for several im-
ages, the salient region is more or less equivalent to
the original image itself (third row). This analysis can
justify the small differences, in terms of performance,
obtained on this dataset by applying the two different
deep strategies.
6 CONCLUSIONS
Considering the task of classifying the sexist con-
tent of advertisements, we have proved that a multi-
modal approach that considers both visual and textual
features permits good classification performance es-
pecially when compared with unimodal approaches.
To best of our knowledge this is the first work that
deals with this task. Within this context we provide
a dataset of advertisements with images and text that
will be available to the research community. Starting
from the promising results here obtained, significant
improvements can be reached with further analysis.
In particularly, we plan to increase the dataset of both
images and texts, and we plan to train a textual clas-
sifier on a more specific task. To automatically ex-
tract the texts, we plan to investigate different ocr, in
fact within this preliminary analysis, texts have been
manually extracted. The extraction of more signifi-
cant salient regions in presence of text should also be
investigated. Finally, other strategies to obtain a mul-
timodal classification will be investigated, consider-
ing both early fusion and late fusion approaches.
ACKNOWLEDGEMENTS
We gratefully acknowledge the support of NVIDIA
Corporation with the donation of the Tesla K40 GPU
used for this research.
REFERENCES
Anzovino, M., Fersini, E., and Rosso, P. (2018). Automatic
Identification and Classification of Misogynistic Lan-
guage on Twitter. In 23rd International Conference on
Natural Language & Information Systems.
Bhattacharya, S., Nojavanasghari, B., Chen, T., Liu, D.,
Chang, S.-F., and Shah, M. (2013). Towards a com-
prehensive computational model foraesthetic assess-
ment of videos. In Proceedings of the 21st ACM inter-
national conference on Multimedia, pages 361–364.
ACM.
Ciocca, G., Corchs, S., and Gasparini, F. (2016). Ge-
netic programming approach to evaluate complexity
of texture images. Journal of Electronic Imaging,
25(6):061408–061408.
Comaniciu, D. and Meer, P. (2002). Mean shift: A robust
approach toward feature space analysis. Pattern Anal-
ysis and Machine Intelligence, IEEE Transactions on,
24(5):603–619.
Corchs, S., Fersini, E., and Gasparini, F. (2017). Ensemble
learning on visual and textual data for social image
emotion classification. International Journal of Ma-
chine Learning and Cybernetics, pages 1–14.
Corchs, S. E., Ciocca, G., Bricolo, E., and Gasparini,
F. (2016). Predicting complexity perception of real
world images. PloS one, 11(6):e0157986.
Gasparini, F., Corchs, S., and Schettini, R. (2008). Recall
or precision-oriented strategies for binary classifica-
tion of skin pixels. Journal of electronic imaging,
17(2):023017–023017.
Hasler, D. and Suesstrunk, S. E. (2003). Measuring col-
orfulness in natural images. In Electronic Imaging
2003, pages 87–95. International Society for Optics
and Photonics.
Hewitt, S., Tiropanis, T., and Bokhove, C. (2016). The
problem of identifying misogynist language on twit-
ter (and other online social spaces). In Proceedings of
the 8th ACM Conference on Web Science, pages 333–
335. ACM.
Hu, W., Wu, O., Chen, Z., Fu, Z., and Maybank, S. (2007).
Recognition of pornographic web pages by classify-
ing texts and images. IEEE transactions on pattern
analysis and machine intelligence, 29(6):1019–1034.
Itti, L. and Koch, C. (2001). Computational modelling of vi-
sual attention. Nature reviews neuroscience, 2(3):194.
Junior, O. L., Delgado, D., Gonc¸alves, V., and Nunes, U.
(2009). Trainable classifier-fusion schemes: An ap-
plication to pedestrian detection. In Intelligent Trans-
portation Systems, 2009. ITSC’09. 12th International
IEEE Conference on, pages 1–6. IEEE.
Krizhevsky, A., Sutskever, I., and Hinton, G. E. (2012). Im-
agenet classification with deep convolutional neural
networks. In Advances in neural information process-
ing systems, pages 1097–1105.
Mack, M. and Oliva, A. (2004). Computational estimation
of visual complexity. In the 12th Annual Object, Per-
ception, Attention, and Memory Conference.
Mikolov, T., Sutskever, I., Chen, K., Corrado, G. S., and
Dean, J. (2013). Distributed representations of words
Multimodal Classification of Sexist Advertisements
405