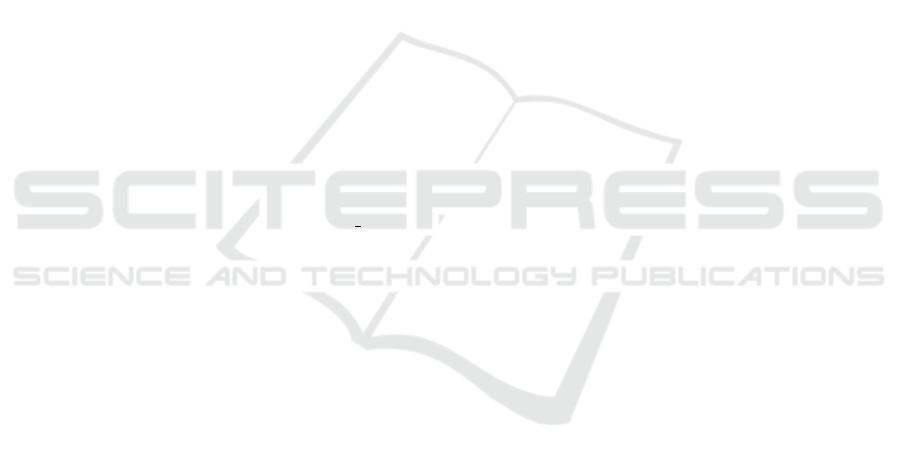
Berlin, E. and Van Laerhoven, K. (2012). Detecting leisure
activities with dense motif discovery. In Proc. of Ubi-
Comp’12, pages 250–259. ACM.
Bosch Sensortec (2017). BHI160 / BHI160B - Ultra
low-power sensor hub incl. integrated imu. BST-
BHI160(B)-DS000-01.
Bulling, A., Blanke, U., and Schiele, B. (2014). A tutorial
on human activity recognition using body-worn iner-
tial sensors. ACM Comput. Surv., 46(3):33:1–33:33.
Capela, N. A., Lemaire, E. D., and Baddour, N. (2015).
Feature selection for wearable smartphone-based hu-
man activity recognition with able bodied, elderly, and
stroke patients. PloS one, 10(4):e0124414.
Dama
ˇ
sevi
ˇ
cius, R., Vasiljevas, M.,
ˇ
Salkevi
ˇ
cius, J., and
Wo
´
zniak, M. (2016). Human activity recognition in
aal environments using random projections. Compu-
tational and mathematical methods in medicine, 2016.
Gordon, D., Czerny, J., Miyaki, T., and Beigl, M. (2012).
Energy-efficient activity recognition using prediction.
In Proc. of ISWC ’12, pages 29–36.
Gr
¨
utzmacher, F., Wolff, J.-P., Hein, A., Lepidis, P., Dorsch,
R., Kirste, T., and Haubelt, C. (2017). Towards energy
efficient sensor nodes for online activity recognition.
In Proc. of IECON’17, pages 8291–8296. IEEE.
Huynh, T. and Schiele, B. (2005). Analyzing features for
activity recognition. In Proc. of sOc-EUSAI ’05, pages
159–163, New York, NY, USA. ACM.
John D. Cook (visited 03/2018). Comput-
ing skewness and kurtosis in one pass.
https://www.johndcook.com/blog/skewness kurtosis/.
Kr
¨
uger, F., Nyolt, M., Yordanova, K., Hein, A., and Kirste,
T. (2014). Computational state space models for activ-
ity and intention recognition. a feasibility study. PloS
one, 9(11):e109381.
Laerhoven, K. V., Gellersen, H.-W., and Malliaris, Y. G.
(2006). Long term activity monitoring with a wearable
sensor node. In Proc. of BSN’06., pages 4–pp. IEEE.
Liang, Y., Zhou, X., Yu, Z., and Guo, B. (2014). Energy-
efficient motion related activity recognition on mobile
devices for pervasive healthcare. Mobile Networks
and Applications, 19(3):303–317.
Lorincz, K., Chen, B.-r., Challen, G. W., Chowdhury, A. R.,
Patel, S., Bonato, P., Welsh, M., et al. (2009). Mer-
cury: a wearable sensor network platform for high-
fidelity motion analysis. In SenSys, volume 9, pages
183–196.
Mamaghanian, H., Khaled, N., Atienza, D., and Van-
dergheynst, P. (2011). Compressed sensing for real-
time energy-efficient ecg compression on wireless
body sensor nodes. IEEE Transactions on Biomedi-
cal Engineering, 58(9):2456–2466.
Marcelloni, F. and Vecchio, M. (2008). A simple algo-
rithm for data compression in wireless sensor net-
works. IEEE communications letters, 12(6).
Pebay, P. P. (2008). Formulas for robust, one-pass parallel
computation of covariances and arbitrary-order statis-
tical moments. Technical report, Sandia National Lab-
oratories.
Rault, T., Bouabdallah, A., Challal, Y., and Marin, F.
(2017). A survey of energy-efficient context recogni-
tion systems using wearable sensors for healthcare ap-
plications. Pervasive and Mobile Computing, 37:23–
44.
Ruberg, P., Lass, K., and Ellervee, P. (2015). Microcon-
troller energy consumption estimation based on soft-
ware analysis for embedded systems. In Proc. of
NORCAS’15, pages 1–4. IEEE.
Russell, J. T. and Jacome, M. F. (1998). Software power
estimation and optimization for high performance, 32-
bit embedded processors. In Proc. of ICCD’98, pages
328–333. IEEE.
Van Laerhoven, K. and Aronsen, A. K. (2007). Memoriz-
ing what you did last week: Towards detailed actigra-
phy with a wearable sensor. In Proc. of ICDCSW’07.,
pages 47–47. IEEE.
Wang, Y., Lin, J., Annavaram, M., Jacobson, Q. A., Hong,
J., Krishnamachari, B., and Sadeh, N. (2009). A
framework of energy efficient mobile sensing for au-
tomatic user state recognition. In Proc. of Mobisys
’09, pages 179–192. ACM.
Yan, Z., Subbaraju, V., Chakraborty, D., Misra, A., and
Aberer, K. (2012). Energy-efficient continuous ac-
tivity recognition on mobile phones: An activity-
adaptive approach. In Proc. of ISWC ’12, pages 17–
24. IEEE.
Ye, W., Heidemann, J., and Estrin, D. (2002). An energy-
efficient mac protocol for wireless sensor networks. In
Proc. of INFOCOM ’02, volume 3, pages 1567–1576.
IEEE.
Younis, O. and Fahmy, S. (2004). Heed: a hybrid, energy-
efficient, distributed clustering approach for ad hoc
sensor networks. IEEE Transactions on mobile com-
puting, 3(4):366–379.
Zappi, P., Lombriser, C., Stiefmeier, T., Farella, E., Roggen,
D., Benini, L., and Tr
¨
oster, G. (2008). Activity recog-
nition from on-body sensors: accuracy-power trade-
off by dynamic sensor selection. In Wireless sensor
networks, pages 17–33. Springer.
PEC 2018 - International Conference on Pervasive and Embedded Computing
92