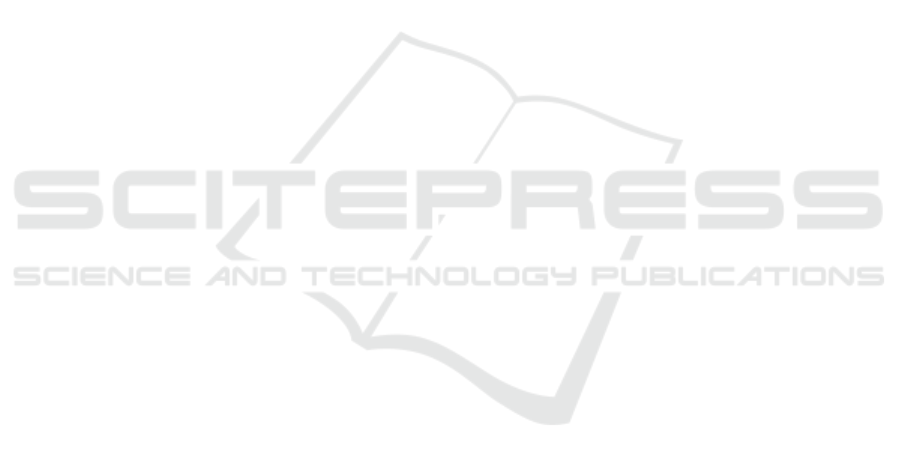
outperforms the others. However, as expected, the
mean confusion rate rises to 0.3515, while, as de-
scribed above, for the 2-class framework it is 0.2385.
5 CONCLUSIONS
This work was motivated by the awareness that one-
day ahead forecasting of k
t
time series cannot be re-
liably performed by using autoregressive models, due
to the lack of autocorrelation at daily scale, as pointed
out in section 2. To overcome this drawback, we tried
to perform 1-step ahead forecasting of the k
t
class.
To this purpose, we have implemented five different
forecasting models. One of them is still a nonlinear
autoregressive model (NNM), while two are proba-
bilistic (the HMM and the NMB). Finally, the two re-
maining are the well-known persistent model (PersM)
and the surrogate model (SurM), based on the genera-
tion of random sequences with predefined probability
density functions. Results, obtained processing time
series of a significant number of recording stations,
point out that forecasting 1-step ahead the class of
daily k
t
in a 2-class framework can be done with rel-
ative high accuracy. In other terms, we can forecast if
the daily k
t
for tomorrow, will be lower or higher than
the threshold value, that in this paper was assumed to
be equal to 0.70, for all the stations. For this purpose,
based on our experience, we recommend the HMM
approach which gives CR ≤ 0.3, for all the stations,
with an average value of about 0.25. Forecasting in
a 3-class framework is still possible but with lower
accuracy, since the CR is, on average, of about 0.35.
However, we believe that improvements are possible
by incorporating into the models some peculiar fea-
tures of the individual stations (e.g. the latitude), an
aspect that in this work has not been taken into ac-
count. Indeed, as we have seen in section 2.4, k
t
prob-
ability density functions are strictly related to latitude
and therefore a classification with fixed thresholds, as
done in this work, can not guarantee the best results.
Work is in progress to this end.
REFERENCES
Antonanzas, J., Osorio, N., Escobar, R., Urraca, R., de Pi-
son, F. M., and Antonanzas-Torres, F. (2016). Re-
view of photovoltaic power forecasting. Solar Energy,
136:78 – 111.
Barbieri, F., Rajakaruna, S., and Ghosh, A. (2017). Very
short-term photovoltaic power forecasting with cloud
modeling: A review. Renewable and Sustainable En-
ergy Reviews, 75:242 – 263.
Ineichen, P. and Perez, R. (2002). A new airmass inde-
pendent formulation for the linke turbidity coefficient.
Physica A, 73:151–157.
Kisi, O. (2014). Modeling solar radiation of mediterranean
region in turkey by using fuzzy genetic approach. En-
ergy Conversion and Management, 64:429–436.
Lauret, P., Boland, J., and Ridley, B. (2013). Bayesian sta-
tistical analysis applied to solar radiation modelling.
Renewable Energy, 49:124–127.
Nian, Z., Behera, P., and Williams, C. (2013). Solar ra-
diation prediction based on particle swarm optimiza-
tion and evolutionary algorithm using recurrent neu-
ral networks. IEEE International Systems Conference
(SysCon) 2013.
Notton, G. and Voyant, C. (2018). Chapter 3 - forecasting
of intermittent solar energy resource. In Yahyaoui, I.,
editor, Advances in Renewable Energies and Power
Technologies, pages 77 – 114. Elsevier.
Perez, R. (2002). A new operational model for satellite-
derived irradiances: Description and validation. Solar
Energy, 73:307–317.
Piri, J. and Kisi, O. (2015). Modelling solar radiation
reached to the earth using anfis, nn-arx, and empirical
models (case studies: Zahedan and bojnurd stations).
Journal of Atmospheric and Solar-Terrestrial Physics,
123:39–47.
Prema, V. and Rao, K. U. (2015). Development of statistical
time series models for solar power prediction. Renew-
able Energy, 83:100–109.
Rabiner, L. R. (1989). A tutorial on hidden markov models
and selected applications in speech recognition. Math-
ematics of Control, Signals, and Systems, 77:257–286.
Raza, M. Q., Mithulananthan, N., and Summerfield, A.
(2018). Solar output power forecast using an ensemble
framework with neural predictors and bayesian adap-
tive combination. Solar Energy, 166:226 – 241.
Sun, H., Yan, D., Zhao, N., and Zhou, J. (2015). Empirical
investigation on modeling solar radiation series with
armagarch models. Energy Conversion and Manage-
ment, 92:385–395.
Viterbi, A. J. (1967). Error bounds for convolutional codes
and an asymptotically optimum decoding algorithm.
IEEE Transactions on Information Theory, 13:260–
269.
Voyant, C., Notton, G., Kalogirou, S., Nivet, M., Paoli, C.,
Motte, F., and Fouilloy, A. (2017). Machine learning
methods for solar radiation forecasting - a review. Re-
newable Energy, 105:569–582.
Forecasting the Class of Daily Clearness Index for PV Applications
179