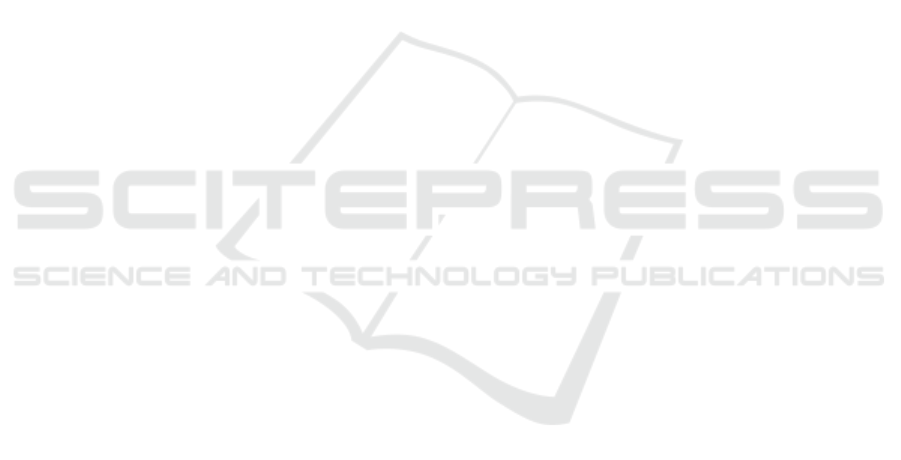
fluence of supra-laryngeal structures, notably the vo-
cal tract and nasal tract resonances. Dynamic aspects
of voice expression are also idiosyncratic and include
articulation gestures related for example to formants
trajectories (i.e. trajectories of the vocal and nasal
tract resonant frequencies and that can be easily iden-
tified, for example, in spectrograms of diphthong re-
gions of the speech signal) and consonant-to-vowel
(and vowel-to-consonant) co-articulation gestures.
Concerning the signal analysis and feature ex-
traction front-end, the dominant approach in current
speaker identification technology involves capturing
speaker-specific voice and articulation traits by means
of signal features that are extracted from the speech
signal. Feature extraction is usually performed using
i) a simple signal segmentation strategy that simply
tries to exclude silence and non-speech sounds, and
ii) using exclusively the magnitude of a spectral rep-
resentation of short signal segments -in the order of
20 ms-, for example through such features as Mel-
Frequency Cepstral Coefficients (MFCCs) (Davis and
Mermelstein, 1980) or parameters extracted from Lin-
ear Predictive Coding (LPC) analysis (Rabiner and
Juang, 1993).
This approach reflects however two assumptions
we believe are not entirely correct. The first assump-
tion is that the same idiosyncratic voice traits per-
sist in all phonetic realizations by the same speaker,
irrespective of their nature being voiced vowels or
unvoiced consonants, for example. In a previous
study, we have shown that using phonetic-oriented
signal segmentation and conventional MFCC and
GMM speaker modeling, speaker identification per-
formance improves significantly relatively to the case
where just uniform, blind, signal segmentation is used
(Mendes and Ferreira, 2012). We therefore argue that
speaker-specific vocal traits are highly localized in
time according to the specificity of the categorical
phonetic realization. It can be argued however that
GMM accommodates this because in a GMM ‘A uni-
modal Gaussian density can be thought of as mod-
eling an acoustic class representing a phonetic event
(like vowel, nasals and fricatives)’ (Ramachandran
et al., 2002, page 2808). Although this is plausible,
to a significant extent we think this can also be a mat-
ter of belief since MFCCs have an intrinsic low res-
olution at high frequencies and are therefore not tai-
lored to capture the subtleties that make for example a
sibilant a special case of a fricative. Although strate-
gies for phonetic-oriented signal segmentation are not
the focus of this paper, we presume one such strat-
egy exists that leads to the segmentation of vowel-like
sounds only, which are those for which it makes sense
to extract features that are related to the phases of the
signal harmonics.
The second incorrect assumption is that phase is
irrelevant in the voice signal analysis and feature ex-
traction process and, thus, has no useful potential in
speaker discrimination. Fortunately, this aspect has
been extensively tackled in the literature and many
authors have addressed the speaker discriminative po-
tential of phase-related features, with positive results,
e.g. (Alam et al., 2015; Wang et al., 2010; Wang
et al., 2009; Nakagawa et al., 2007; Rajan et al.,
2013; Padmanabhan et al., 2009). Taking as a ref-
erence the speaker identification performance that is
achieved using MFCC-related features alone, which
have proven so far to be the most effective acous-
tic features and, therefore, constitute a benchmark-
ing standard easily offering above around 70% correct
identification, it has been concluded that, in general,
phase related features help to improve the MFCC-
based scores between 0.0% and less than 10.0%, e.g.
(Wang et al., 2010).
The novelty of our paper lies in the nature of
the phase-related features. In fact, our approach is
based on a phase-related feature involving the ac-
curate phase relationships between the relevant har-
monic components in a quasi-periodic signal. We
named this feature as Normalized Relative Delay
(NRD). In short, NRD coefficients are equivalent to
the parametric phase of the harmonics when a Fourier
analysis is performed for any periodic signal. To-
gether with the accurate magnitudes of those harmon-
ics, they fully characterize the shape invariance of any
periodic waveform. Section 3.1 provides additional
information on the NRD feature.
This paper is a follow-up to a previous paper (Fer-
reira, 2014) in which we concluded NRD coefficients
(NRDs) possess the potential to discriminate between
speakers because NRDs exhibit essentially the same
profile even for different vowels uttered by the same
speaker, i.e. they reflect the idiosyncratic nature of
the glottal pulse of a specific speaker rather than the
contribution of the vocal/nasal tract filter which obvi-
ously changes for different vowels because it is their
spectral envelope that conveys the linguistic meaning.
This is a conclusion which we believe also brings in-
novative insights as we discuss in (Ferreira and Tri-
bolet, 2018). In this paper, however, we take as a ref-
erence other results in the literature that also address
phase-related features.
For example, (Nakagawa et al., 2007; Wang et al.,
2009; Wang et al., 2010) use the phase of the first 12
coefficients of a DFT analysis which is made relative
to the phase of the 1 kHz reference DFT bin. This
relative phase is projected into the coordinates of a
unit circle and the result is subject to Gaussian Mix-
SIGMAP 2018 - International Conference on Signal Processing and Multimedia Applications
348