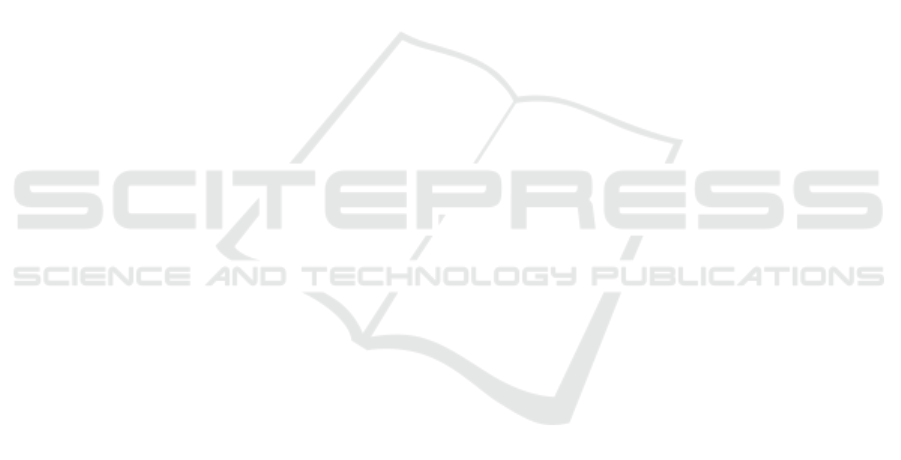
which was developed in this paper. This method is
easy to apply and it makes the number of CPT’s asses-
sments linear instead of exponential. However, it pre-
sents two major issues when the expert must give the
compatible parental configuration. The first is where
the expert cannot select a single compatible parental
configuration for a given state. This issue was solved
in the literature. By contrast, the second isn’t. The
problem is when expert still unable to give compatible
parental configuration for each state with other states
of other parents. As a solution for this issue, we pro-
posed, in this paper, a cluster approach by including
a intermediate node. We applied the WSA on a gene-
rated cluster and we used Raw method of elicitation
for the rest of nodes. Then, we applied our method
on a part of BN of our case study. To facilitate the
application of WSA, we have combine various diffe-
rent concepts. Among which we elaborate a table for
compatible parental configurations which is easy to
complete by the expert. In addition, we use a scale
of probabilities and a scale of weights to facilitate the
task of elicitation to him. In addition, we developed
two practices equations to calculate the number of in-
formation needed from expert one for the WSA and
the other for our method. After that, we compared in-
formation number needed obtained from our method
with WSA and the Raw method by varying the cluster
size and states number of intermediate node. Through
using our method, the information number to elicit
is less important than that obtained by the Raw met-
hod. But this is not always the case compared with
the WSA. This comparison allows us to admit that
our clustering method is better than the Raw method
but it’s still not good sometimes compared to WSA
knowing that it has remedied the scientific issue en-
countered.
It is true that our clustering approach reduces the
number of information to be elicited in order to deve-
lop our CPT and remedied the scientific issue encoun-
tered with WSA, nevertheless like other methods, it
has some limits. In our next research, we will try to
find them and to compare our method with other exis-
ting methods. Moreover, in this paper, we present our
method to remedy the problem of compatible paren-
tal configuration and we have treated a single cluster.
As a prospect, we may wonder what will become our
method and results if we have several clusters.
REFERENCES
Baker, S. and Mendes, E. (2010). Assessing the weighted
sum algorithm for automatic generation of probabili-
ties in bayesian networks. In Information and Auto-
mation (ICIA), 2010 IEEE International Conference
on, pages 867–873. IEEE.
Bolt, J. H. and van der Gaag, L. C. (2010). An empiri-
cal study of the use of the noisy-or model in a real-
life bayesian network. In International Conference
on Information Processing and Management of Un-
certainty in Knowledge-Based Systems, pages 11–20.
Springer.
Burgman, M., Fidler, F., Mcbride, M., Walshe, T., and
Wintle, B. (2006). Eliciting expert judgments: lite-
rature review.
Constantinou, A. C., Fenton, N., and Neil, M. (2016). In-
tegrating expert knowledge with data in bayesian net-
works: Preserving data-driven expectations when the
expert variables remain unobserved. Expert systems
with applications, 56:197–208.
Cooke, R. M. and Goossens, L. H. (2000). Procedures
guide for structural expert judgement in accident con-
sequence modelling. Radiation Protection Dosimetry,
90(3):303–309.
Das, B. (2004). Generating conditional probabilities for
bayesian networks: Easing the knowledge acquisition
problem. arXiv preprint cs/0411034.
Druzdel, M. and Van Der Gaag, L. C. (2000). Building
probabilistic networks:” where do the numbers come
from?”. IEEE Transactions on knowledge and data
engineering, 12(4):481–486.
Fenton, N. and Neil, M. (2012). Risk assessment and deci-
sion analysis with Bayesian networks. Crc Press.
Kemp-Benedict, E. (2008). Elicitation techniques for bay-
esian network models. In Forthcoming SEI Working
Paper.
Knochenhauer, M., Swaling, V. H., Dedda, F., Hansson, F.,
Sj
¨
okvist, S., and Sunnegaerd, K. (2013). Using bay-
esian belief network (bbn) modelling for rapid source
term prediction–final report.
Kraaijeveld, P., Druzdzel, M. J., Onisko, A., and Wasyluk,
H. (2005). Genierate: An interactive generator of di-
agnostic bayesian network models. In Proc. 16th Int.
Workshop Principles Diagnosis, pages 175–180. Cite-
seer.
Kuhnert, P. M., Martin, T. G., and Griffiths, S. P. (2010). A
guide to eliciting and using expert knowledge in bay-
esian ecological models. Ecology letters, 13(7):900–
914.
Mkrtchyan, L., Podofillini, L., and Dang, V. N. (2016).
Methods for building conditional probability tables of
bayesian belief networks from limited judgment: an
evaluation for human reliability application. Reliabi-
lity Engineering & System Safety, 151:93–112.
Na
¨
ım, P., Wuillemin, P., Leray, P., Pourret, O., and Becker,
A. (1999). R
´
eseaux bay
´
esiens. paris: Eyrolles.
Nguyen, X. (2007). Algorithmes probabilistes appliqu
´
es
`
a
la durabilit
´
e et
`
a la m
´
ecanique des ouvrages de G
´
enie
Civil, Ph. D. PhD thesis, thesis, Toulouse.
Pearl, J. (2014). Probabilistic reasoning in intelligent sys-
tems: networks of plausible inference. Elsevier.
Renooij, S. (2001). Probability elicitation for belief net-
works: issues to consider. The Knowledge Engineer-
ing Review, 16(3):255–269.