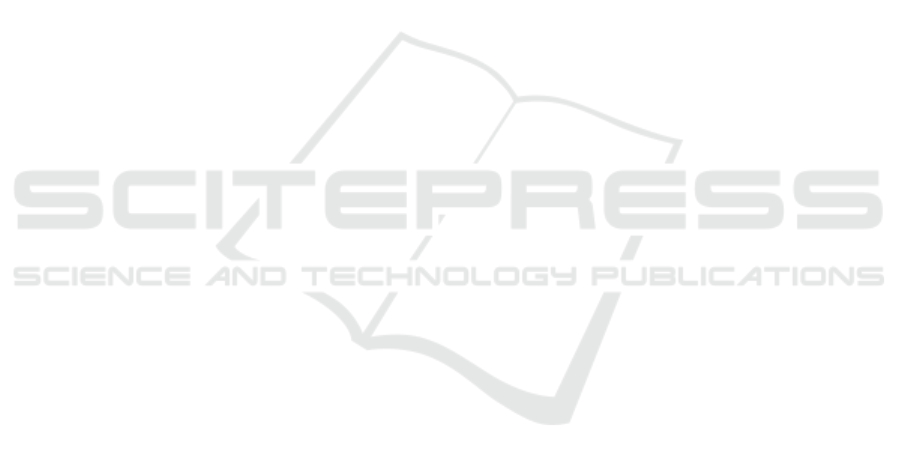
Intelligence. Semantic Web, Social Networks and
Multiagent Systems. Lecture notes in computer
science, 5796, 163-173.
Atmosukarto, I., Ghanem, B., Ahuja, S., Muthuswamy, K.,
Ahuja, N. (2013). Automatic recognition of offensive
team formation in American Football plays. 2013 IEEE
Conference on computer vision and pattern
recognition, 991-998.
Barrick, M.R., Stewart, G.L., Neubert, M.J. (1998). Mount
relating member ability and personality to work-team
processes and team effectiveness. Journal of Applied
Psychology, 83, 377-391.
Bialkowski, A., Lucey, P., Carr, P., Yue, Y., Matthews, I.
(2014). “Win at Home and Draw Away”: Automatic
Formation Analysis Highlighting the Differences in
Home and Away Team Behaviors. Disney Research
Boon, B.H., and Sierksma, G. (2003). Team formation:
Matching quality supply and quality demand. European
Journal of Operational Research, 148(2), 277-292.
Bogaert, P., Van de Weghe, N., Cohn., A.G., Witlox, F., De
Maeyer, P. (2007). The Qualitative Trajectory Calculus
on Networks. In Barkowsky, T., Knauff, M., Ligozat,
G., Montello, D. (Eds). Spatial cognition V reasoning,
action, interaction. Lecture notes in computer science,
4387, 20-38. Springer, Berlin, Heidelberg.
Carling, C. (2011). Influence of opposition team formation
on physical and skill-related performance in a
professional soccer team. European Journal of Sport
Science, 11(3), 155-164.
Colleen Stuart, H. (2017). Structural disruption,
experimentation and performance in professional
hockey teams: A network perspective on member
change. Organization Science, 28(2), 283-300.
Dezman, B., Trinic, S., Dizdar, D. (2001). Expert model of
decision making system for efficient orientation of
basketball players to positions and roles in the game -
empirical verification. Collegium Antropologicum,
25(1), 141-152.
D’Orazio, T., and Leo, M. (2010). A review of vision-based
systems for soccer video analysis. Pattern Recognition,
43(8), 2911-2926.
Feuerhake, U. (2016). Recognition of Repetitive movement
patterns-the case of football analysis. International
Journal of Geo-Information, 5(11), 208.
Frencken, W., de Poel, H., Visscher C., Lemmink, K.
(2012). Variability of inter-team distances associated
with match events in elite standard-soccer. Journal of
Sport Science, 30(12), 1207-1213.
Gonalves, B.V., Figueira, B.E., Mas, V., Sampaio, J.
(2014). Effect of player position on movement
behaviour, physical and physiological performances
during an 11-a-side football game. Journal of Sports
Sciences, 32(2), 191-199.
Grunz, A., Memmert, D., Perl, J. (2012). Tactical pattern
recognition in soccer games by means of special self-
organizing maps. Human Movement Science, 31(2),
334-343.
Jäger, M.J., and Schöllhorn, W.I. (2012). Identifying
individuality and variability in team tactics by means of
statistical shape analysis and multilayer perceptrons.
Human Movement Science, 31(2), 303-317.
Kaminka, G.A., Fidanboylu, M., Chang, A., Veloso, M.M.
(2003). Learning the sequential coordinated behavior of
teams from observations. In Kaminka, G.A., Lima,
P.U., Rojas, R. (Eds.). RoboCup 2002: Robotic soccer
world cup VI. Lecture notes in computer science, 111-
125. Springer, Berlin, Heidelberg.
Kuhlmann, G., Stone, P., Lallinger, J. (2005). The UT
Austin villa 2003 champion simulator coach: A
machine learning approach. In Nardi, D., Riedmiller,
M., Sammut, C., Santos-Victor, J. (Eds.). RoboCup
2004: Robot Soccer World Cup VIII. Lecture notes in
computer science, 3276, 636-644. Springer, Berlin,
Heidelberg.
Lemmink, K., and Frencken, W. (2013). Tactical
performance analysis in invasion games: Perspectives
from a dynamical system approach with examples from
soccer. In McGarry, T., O’Dono-ghue, P., Sampaio, J.
(Eds.). Routledge Handbook of Sports Performance
Analysis (pp. 89-100). London: Routledge.
Lucey, P., Bialkowski, A., Carr, P., Morgan, S., Matthews,
I., Sheikh, Y. (2013). Representing and discovering
adversarial team behaviors using player roles. 2013
IEEE Conference on computer vision and pattern
recognition, 2706-2713.
Lucey, P., Bialkowski, A., Carr, P., Yue, Y., Matthews, I.
(2014). “How to get an open shot”: Analyzing team
movement in basketball using tracking data. Disney
research.
Mavridis, N., Belloto, N., Iliopoulos, K., Van de Weghe, N.
(2015). QTC
3D
: extending the qualitative trajectory
calculus to three dimensions. Information Sciences,
322(1), 20-30.
McCulloch, W.S., and Pitts, W. (1943). A logical calculus
of ideas immanent in nervous activity. Bulletin of
Mathematical Biophysics, 5(4), 115-133.
Memmert, D., Lemmink, K.A.P.M., Sampaio, J. (2017).
Current Approaches to Tactical Performance Analysis
in Soccer. Sports Medicine, 47(1), 1-10.
Perin, C., Vuillemot, R., Fekete, J.D. (2013). SoccerStories:
A kick-off for visual soccer analysis. IEEE Trans. Vis.
Comput. Graph, 19(12), 2506-2515.
Rein, R., and Memmert, D. (2016). Big data and tactical
analysis in elite soccer: future challenges and
opportunities for sports science. Springerplus, 5(1),
1410.
Sampaio, J., and Macãs, V. (2012). Measuring tactical
behaviour in football. International Journal of Sports
Medicine, 33(5), 395-401.
Sarmento, H., Pereira, A., Anguera, M., Campanico, J.,
Leitao, J. (2014). The coaching process in football – A
qualitative perspective. Montenegrin Journal of Sports
Science and Medicine, 3(1), 9-16.
Tierney, P.J., Young, A., Clarke, N.D., Duncan, M.J.
(2016). Match play demands of 11 versus 11
professional football using global positioning, system
tracking: Variations across common playing
formations. Human Movement Science, 49(1), 1-8.
Analysing Team Formations in Football with the Static Qualitative Trajectory Calculus
21