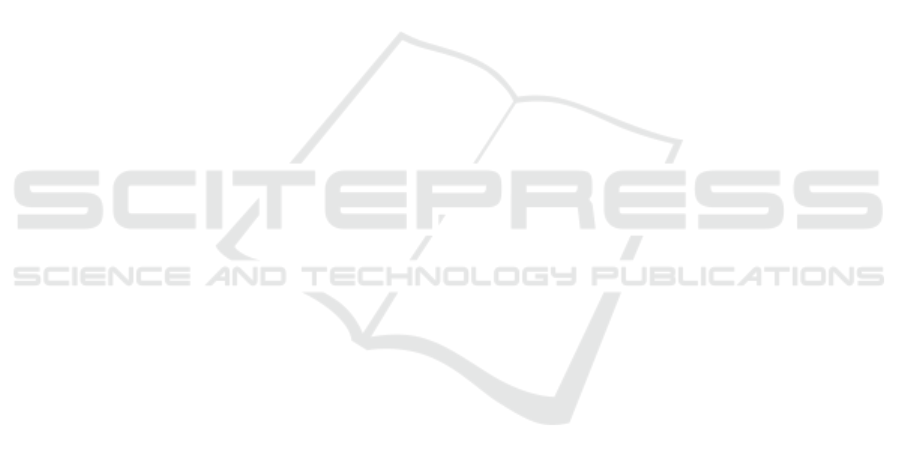
of Nanjing and the sub-centers. The result can be used
to support and examine the development agenda of
Nanjing City Council, where city officials proposed
to further grow sub-centers to release the pressure
from the central business area. In addition, we also
explored the temporal dynamics of different urban
regions based on a DTW algorithm. The extracted
outliers demonstrated the importance of
incorporating human mobility and activity data to
refine small-area land use classification. Further
research can focus on incorporating more indicators
from the taxi trajectories to improve the accuracy of
the analysis. It is also important to cross-validate the
results with other public data, such as census and
urban demographic data. Also, further research may
involve extending the study period to analyze
seasonal time series patterns when the data becomes
available. The methodology discussed in this paper
can be applied to other cities to identify urban
functional regions and provide useful input for policy
makers.
REFERENCES
Ahas, R. 2005. Mobile phones and geography: Social
Positioning Method. Power over Time-Space:
Inaugural Nordic Geographers Meeting. Lund,
Sweden.
Atmaji, F. T. D. & Sig, K. Y. 2016. Mining the GPS big
data to optimize the taxi dispatching management. 2016
4th International Conference on Information and
Communication Technology (ICoICT).
Calabrese, F., Diao, M., Di Lorenzo, G., Ferreira, J. & Ratti,
C. 2013. Understanding individual mobility patterns
from urban sensing data: A mobile phone trace
example. Transportation Research Part C-Emerging
Technologies, 26, 301-313.
Cao, G., Wang, S., Hwang, M., Padmanabhan, A., Zhang,
Z. & Soltani, K. 2015. A scalable framework for
spatiotemporal analysis of location-based social media
data. Computers, Environment and Urban Systems, 51,
70-82.
Castro, P. S., Zhang, D. Q., Chen, C., Li, S. J. & Pan, G.
2013. From Taxi GPS Traces to Social and Community
Dynamics: A Survey. ACM Computing Surveys, 46.
Chen, B. Y., Yuan, H., Li, Q., Shaw, S.-L., Lam, W. H. K.
& Chen, X. 2015. Spatiotemporal data model for
network time geographic analysis in the era of big data.
International Journal of Geographical Information
Science, 1-31.
Chen, Z. L., Gong, X. & Xie, Z. 2017. An analysis of
movement patterns between zones using taxi GPS data.
Transactions in GIS, 21, 1341-1363.
Cui, J. X., Liu, F., Janssens, D., An, S., Wets, G. & Cools,
M. 2016. Detecting urban road network accessibility
problems using taxi GPS data. Journal of Transport
Geography, 51, 147-157.
Delafontaine, M., Versichele, M., Neutens, T. & Van De
Weghe, N. 2012. Analysing spatiotemporal sequences
in Bluetooth tracking data. Applied Geography, 34,
659-668.
Fanhas, R. S. & Saptawati, G. a. P. 2016. Discovering
Frequent Origin-Destination Flow from Taxi GPS Data.
Proceedings of 2016 International Conference on Data
and Software Engineering (ICoDSE).
Ferreira, N., Poco, J., Vo, H. T., Freire, J. & Silva, C. T.
2013. Visual Exploration of Big Spatio-Temporal
Urban Data: A Study of New York City Taxi Trips. Ieee
Transactions on Visualization and Computer Graphics,
19, 2149-2158.
Ge, W., Shao, D., Xue, M., Zhu, H. & Cheng, J. 2017.
Urban Taxi Ridership Analysis in the Emerging
Metropolis: Case Study in Shanghai. Transportation
Research Procedia, 25, 4916-4927.
Hu, X. W., An, S. & Wang, J. 2014. Exploring Urban Taxi
Drivers' Activity Distribution Based on GPS Data.
Mathematical Problems in Engineering, 708482.
Hu, X. W., An, S. & Wang, J. 2018. Taxi Driver's Operation
Behavior and Passengers' Demand Analysis Based on
GPS Data. Journal of Advanced Transportation,
6197549.
Jiang, B., Yin, J. J. & Zhao, S. J. 2009. Characterizing the
human mobility pattern in a large street network.
Physical Review E, 80, 021136-1 - 021136-11.
Jiang, W. W. & Zhang, L. 2018. The Impact of the
Transportation Network Companies on the Taxi
Industry: Evidence from Beijing's GPS Taxi Trajectory
Data. IEEE Access, 6, 12438-12450.
Lee, J. H., Davis, A. W., Yoon, S. Y. & Goulias, K. G.
2016. Activity space estimation with longitudinal
observations of social media data. Transportation, 43,
955-977.
Liu, X., Kang, C. G., Gong, L. & Liu, Y. 2016.
Incorporating spatial interaction patterns in classifying
and understanding urban land use. International
Journal of Geographical Information Science, 30, 334-
350.
Liu, Y., Kang, C. G., Gao, S., Xiao, Y. & Tian, Y. 2012.
Understanding intra-urban trip patterns from taxi
trajectory data. Journal of Geographical Systems, 14,
463-483.
Mardia, K. V., Kent, J. T. & Bibby, J. M. 1979. Multivariate
analysis, London & New York, Academic Press.
Mazimpaka, J. D. & Timpf, S. 2015. Exploring the
Potential of Combining Taxi GPS and Flickr Data for
Discovering Functional Regions. AGILE 2015:
Geographic Information Science as an Enabler of
Smarter Cities and Communities, 3-18.
Nanjing City Council. 1995. The development agenga of
Nanjing: 1991-2010 [Online]. Available:
http://www.njghj.gov.cn [Accessed].
Santi, P., Resta, G., Szell, M., Sobolevsky, S., Strogatz, S.
H. & Ratti, C. 2014. Quantifying the benefits of vehicle
pooling with shareability networks. Proceedings of the