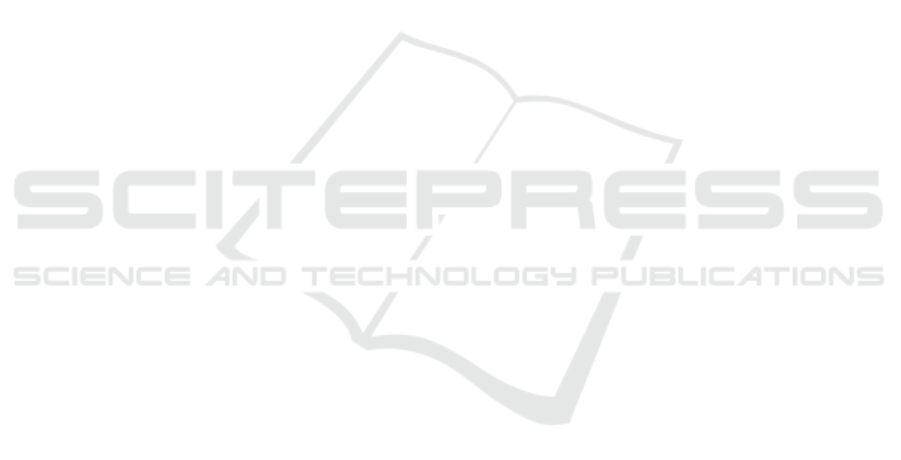
possible activities in a given situation and they does
not leave it to the user to decide which one he
prefers to perform.
Regarding process recommendation, we can
mention the following contribution: (Hornung et al.,
2007), (Macé-Ramette et al., 2013), (Ribeiro et al.,
2014) and (Ariouat et al., 2018). Note that process
recommendation is rather useful at design-time, i.e.
before process execution. Both (Macé-Ramette et
al., 2013) and (Ariouat et al., 2018) have the same
objective: the recommendation of a process model to
deal with observed facts during a crisis. Both use
business knowledge of crisis actors to deduce the
crisis resolution process to be performed for
reducing the crisis in the field. Unfortunately, none
of them highlight the possible options to deal with
observed facts and leave it to the user to decide
which one he prefers to perform. In (Hornung et al.,
2007), recommendation for designing processes is
based on process reuse. Indeed, in this work, there is
an ontology-based comparison between a process
model being designed, expressed as a Petri net, and a
set of already existing process models, also
expressed as Petri net. The result of this comparison
is the process model closest syntactically to the one
being designed. Finally (Ribeiro et al., 2014)
describes a recommender system that help users in
choosing the best discovery algorithm for their data.
This system uses as input a log file (data) and the
different process discovery techniques.
Measurements such as fitness and generalisation are
used for the evaluation of the performance and the
quality of these techniques. The system recommends
process discovery techniques according to the best
measures.
This work, led in the context of the GéNéPi
project in collaboration with crisis cell of county 45
in France, focuses on process recommendation, i.e.
recommendation of a set of coordinated activities, to
deal with observed facts in the field. Indeed, crisis
cells have two mains needs. First they need to have a
comprehensive view of the set of activities to be
performed (and their coordination) to cope with each
observed fact (e.g., to be able to evaluate the
resource required to carry out all the activities and
ask for help to other countys if necessary). Second,
as they are responsible for the response in the field,
crisis cells want to know the possible options
(strategies) to deal with each fact observed, to assess
these strategies and decide by themselves the ones
that are the most suitable. Our solution meets these
two needs as it recommends strategies and processes
implementing them to cope with each facts
observed. It orders these strategies (and processes)
comparing the context of the current situation and
the context of possible strategies. Our solution
differs from the existing ones cited above. The closet
ones are (Negre, 2013) and (Ariouat et al., 2018):
the first one recommends activities to be performed
when unexpected situations occur during crisis while
the other one describes a solution to deduce from
facts observed in the field the crisis resolution
process to be performed to cope with these facts.
However, (Negre, 2013) does not meet the first
need. Moreover, it advocates a context-based
comparison but conditions featuring contexts are
only equalities, which is really a drawback as
illustrated in Section 5. As for (Ariouat et al., 2018),
it does not meet the second need as it does not offer
any options to crisis cells for their response in the
field.
3 FACTS AND BUSINESS
KNOWLEDGE MODELLING
This section introduces the recommended meta-
model for facts and business knowledge modelling.
This meta-model is given in Figure 1 as an UML
class diagram.
Regarding facts modelling, the main concept is
Observed Risk/Damage. This concept corresponds to
an observed fact in the field, which can either be risk
or damage. Damage is a negative situation affecting
for instance population (e.g., flooded house with
people inside), building (e.g., flooded school), road
(e.g., cut-off road)…, while a risk is the potential for
damage. For each risk or damage, we store whether
if it is risk or damage, and whether if it is known or
unknown. When it is known, we refer to the
knowledge base and more particularly we refer to
Intrinsic Risk/Damage (relationship correspond),
which corresponds to the known solution to the
considered damage or risk. When it is unknown, the
crisis cell has to specify how to deal with the new
risk or damage, indicating which services to be
deployed in the field. In addition, for each risk or
damage, we store a specific property indicating if the
risk or damage takes priority or not. A priority risk
or damage has to be considered in the
recommendation process, while a non-priority risk
or damage will be taken into account later, when
another recommendation is made. Crisis cell
members may change the value of this property
according to the urgency of risk or damage.
Process Recommendation using Context in Crisis Management: Application to Flood Management
113