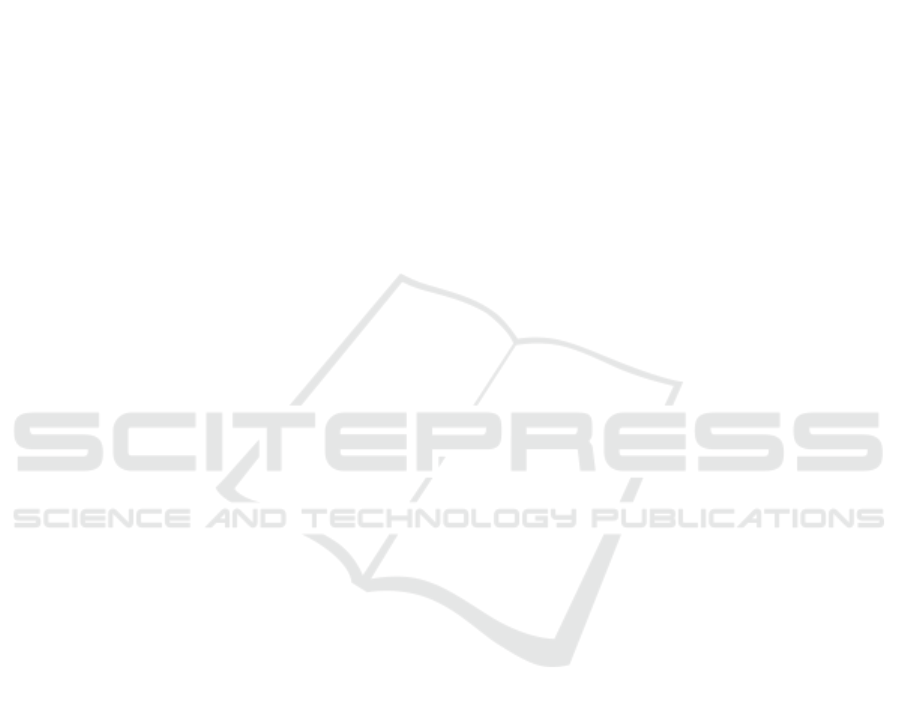
Variation of the EEG-Energy in a Second Language Class
Freddy L. Bueno-Palomeque
1
, Efr
´
en L. Lema-Condo
1
, Susana E. Castro-Villalobos
1,2
,
Luis J. Serpa-Andrade
1
and Esteban F. Ordo
˜
nez-Morales
1
1
Grupo de Investigaci
´
on en Ingenier
´
ıa Biom
´
edica GIIB-UPS, Universidad Polit
´
ecnica Salesiana, Cuenca, Ecuador
2
Departamento de Idiomas, Universidad Polit
´
ecnica Salesiana, Cuenca, Ecuador
Keywords:
Electroencephalographic Signals, Active Attention, Correlation, Signal Energy, Alpha Waves, Beta Waves.
Abstract:
The attention, concentration, and anxiety of the students are fundamental in the learning process of a second
language. This study proposes to register EEG signals during a second language class to quantify the energy
level of the EEG signal and associate it with the attention level along the time. Data was registered while
attending to a level 1 English language through 12 minutes. The signals were filtered using a wavelet transform
Symlet 6 to analyze two frequency bands: Alpha (8-16 Hz) and Beta (16-32 Hz). The results revealed an
increment in the energy on the electrodes AF3, AF4, F3, and F4 in the Alpha and Beta bands between 37.25 and
43.41% of the students, and a decrement between 25.49 and 43.13%. Finally, when analyzing the electrodes
T7 y T8, there were an increment of the energy in 35.30% of the students and decrements between 39.22 and
47.60% of theirs.
1 INTRODUCTION
The knowledge and domain of English as a second
language in Ecuador has presented a slight impro-
vement in the last year according to the English Pro-
fiency Index (EPI) presented by English First (EF)
in 2017, locating it in the 55th position, and consi-
dering it as a low domain category of the language
(First, 2017). The attention, concentration, memory,
and anxiety of the students is fundamental in the lear-
ning of a second language process; however, the made
efforts by the teachers to keep the students’ attention
during a class are quantified and related to each ot-
her using traditional tools as questionnaires, visual
manual reports, or monitoring the students behavior
through the use of information and communication
technologies (ICTs). Monitoring a class through vi-
deo recordings can provide information about the
students-teacher interaction with the finality of trai-
ning and preparing a teacher for a determined class
(Jamil et al., 2015); nevertheless, the behavior in a
class does not provide evidence of the attention level
that each student presents in the class.
The analysis of electroencephalographic signals
(EEG) recorded in people subjected to different ex-
ternal stimuli can reveal important information about
the neural processes involved in a learning process. A
physiological EEG signal can be recorded second by
second, accurately showing changes in attention, fa-
tigue or mental workload in practical work or study
environments (Berka et al., 2007). Determining the
level of attention of a user using EEG signals has a
wide range of applications such as the improvement
of a brain computer interface or from the perspective
of learning, for the development of linguistic skills,
where the attention given to a class is a fundamental
element of the process (Cohen et al., 1990; Aliakba-
ryhosseinabadi et al., 2017). In particular, the lear-
ning of a second language has been analyzed from
different perspectives, such as the influence of age on
learning (Kroll and Tokowicz, 2005), or the applica-
tion of emotions recognition systems, from another
perspective, seeking to reduce anxiety based on lan-
guage for better development of a conversation in the
class environment (Chen and Lee, 2011).
The use of a commercial and portable EEG sy-
stem is presented in (Poulsen et al., 2017), where the
inter-subject correlation of the signals generated by
observing a video in a real class is analyzed, demon-
strating that this method can be used in a commer-
cial system as biofeedback for the tutor. The imple-
mentation of neurofeedback on children with atten-
tion deficit is presented in (Kim et al., 2014) through
the analysis of the relationship between the relative
spectrum of Beta/Theta. Despite the fact that no sig-
nificant changes were observed in the EEG signal, the
effects of the neurofeedback treatment could be evi-
denced.
Bueno-Palomeque, F., Lema-Condo, E., Castro-Villalobos, S., Serpa-Andrade, L. and Ordoñez-Morales, E.
Variation of the EEG-Energy in a Second Language Class.
DOI: 10.5220/0006895800410045
In Proceedings of the 6th International Congress on Neurotechnology, Electronics and Informatics (NEUROTECHNIX 2018), pages 41-45
ISBN: 978-989-758-326-1
Copyright © 2018 by SCITEPRESS – Science and Technology Publications, Lda. All rights reserved
41