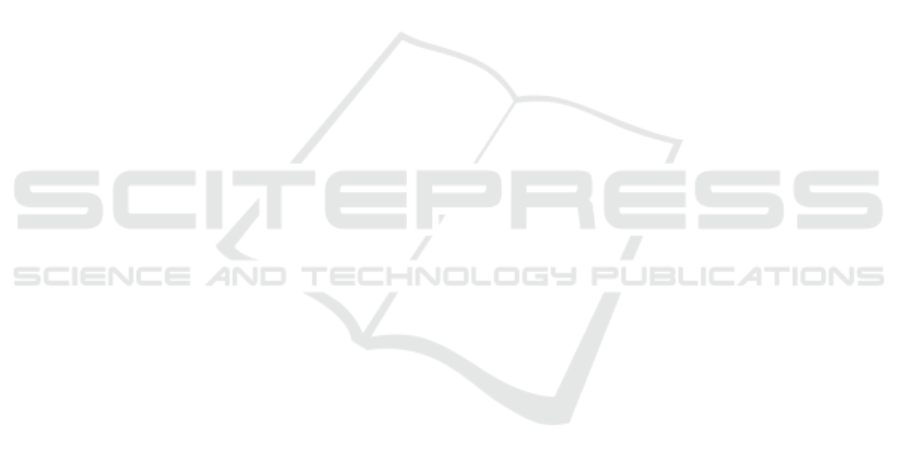
a variety of different user states: ambulatory or dis-
tracting settings, during physical exertion or exercise,
under the influence of caffeine or alcohol, etc., as well
as over longer periods of time or in multiple recording
sessions. While these additional conditions may limit
the performance of the system, it is interesting to con-
sider which if any limiations might be advantageous
in some way. For example, a system that prevents or
allows access only when a user is in a certain state
of mind or setting, or enforces a biologically-based
expiration that requires classifier re-training and thus
offers protection in a scenario where a user’s original
EEG pattern was somehow leaked or surreptitiously
stored.
Finally, our work leaves room for some clear user
experience improvements. Future work should test
the performance of this system using dry electrodes,
which are commonly found in consumer EEG devi-
ces and have shown recent promise for ear EEG sy-
stems (Kappel et al., 2018), as eliminating the need
for conductive gel would very likely improve com-
fort and usability and it is unlikely any system invol-
ving gel will be widely adopted. Future work should
also attempt a closed-loop (or online) passthought sy-
stem, in which users receive immediate feedback on
the result of their authentication attempt. A closed-
loop BCI system would assist in understanding how
human learning effects might impact authentication
performance, as the human and machine co-adapt.
8 CONCLUSION
We build a case that using personalized, custom-fit
ear-EEG earpieces in conjunction with a passthoughts
authentication paradigm offers a viable and attractive
path to one-step three-factor authentication. The ear-
piece form factor provides a discreet yet robust met-
hod for acquiring EEG signals, and we are able to
achieve a 99.82% authentication accuracy using a sin-
gle earpiece with three small electrodes, showing the
potential for integration with technology already used
in everyday life (like earphones). By expanding our
corpus of EEG readings (in population size, time, and
diversity of settings), we can better understand the un-
derlying distribution of EEG signals and security pro-
perties of passthoughts, as well as interrogate usabi-
lity issues that may arise in different contexts.
REFERENCES
Akkermans, A. H. M., Kevenaar, T. A. M., and Schobben,
D. W. E. (2005). Acoustic ear recognition for person
identification. In Proceedings - Fourth IEEE Works-
hop on Automatic Identification Advanced Technolo-
gies, AUTO ID 2005, volume 2005, pages 219–223.
Armstrong, B. C., Ruiz-Blondet, M. V., Khalifian, N.,
Kurtz, K. J., Jin, Z., and Laszlo, S. (2015). Brainprint:
Assessing the uniqueness, collectability, and perma-
nence of a novel method for erp biometrics. Neuro-
computing, 166:59–67.
Ashby, C., Bhatia, A., Tenore, F., and Vogelstein, J. (2011).
Low-cost electroencephalogram (EEG) based authen-
tication. In 2011 5th International IEEE/EMBS Con-
ference on Neural Engineering, NER 2011, pages
442–445.
Braz, C. and Robert, J.-M. (2006). Security and usability:
the case of the user authentication methods. In Procee-
dings of the 18th Conference on l’Interaction Homme-
Machine, pages 199–203. ACM.
Chen, T. and Guestrin, C. (2016). XGBoost : Reliable
Large-scale Tree Boosting System. arXiv, pages 1–
6.
Chen, Y., Sun, J., Zhang, R., and Zhang, Y. (2015). Your
song your way: Rhythm-based two-factor authenti-
cation for multi-touch mobile devices. In Compu-
ter Communications (INFOCOM), 2015 IEEE Con-
ference on, pages 2686–2694. IEEE.
Chuang, J. (2014). One-Step Two-Factor Authentication
with Wearable Bio-Sensors.
Chuang, J., Nguyen, H., Wang, C., and Johnson, B. (2013).
I think, therefore I am: Usability and security of au-
thentication using brainwaves. In International Confe-
rence on Financial Cryptography and Data Security,
pages 1–16.
Curran, M. T., Yang, J.-k., Merrill, N., and Chuang, J.
(2016). Passthoughts authentication with low cost ea-
reeg. In Engineering in Medicine and Biology Society
(EMBC), 2016 IEEE 38th Annual International Con-
ference of the, pages 1979–1982. IEEE.
Frey, J. (2016). Comparison of an open-hardware electroen-
cephalography amplifier with medical grade device in
brain-computer interface applications. Proceedings
of the 3rd International Conference on Physiological
Computing Systems, (PhyCS):105–114.
Garrett, D., Peterson, D. A., Anderson, C. W., and Thaut,
M. H. (2003). Comparison of linear, nonlinear, and fe-
ature selection methods for EEG signal classification.
IEEE Transactions on Neural Systems and Rehabili-
tation Engineering, 11(2):141–144.
Genaro Motti, V. and Caine, K. (2014). Understanding the
wearability of head-mounted devices from a human-
centered perspective. In Proceedings of the 2014 ACM
International Symposium on Wearable Computers, pa-
ges 83–86. ACM.
Holz, C. and Knaust, M. (2015). Biometric touch sen-
sing: Seamlessly augmenting each touch with conti-
nuous authentication. In Proceedings of the 28th An-
nual ACM Symposium on User Interface Software &
Technology, pages 303–312. ACM.
Kappel, S. L., Rank, M. L., Toft, H. O., Andersen, M., and
Kidmose, P. (2018). Dry-contact electrode ear-eeg.
IEEE Transactions on Biomedical Engineering.
PhyCS 2018 - 5th International Conference on Physiological Computing Systems
40