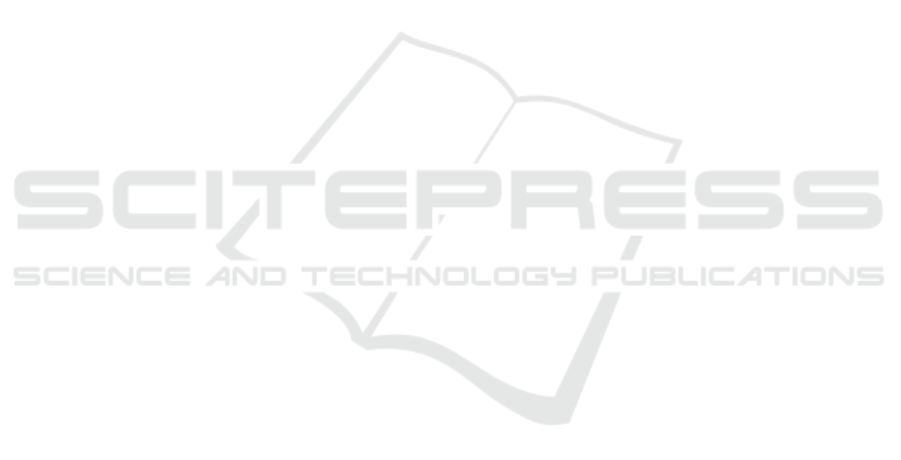
baseline. During this time interval the fixation point
was shown. The PSD of the events of each set was
estimated using the Welch’s method for each sub-
ject, and each electrode. Also the PSD of the corre-
sponding baselines were evaluated. One-way ANaly-
sis Of Variance (ANOVA) was then applied for each
of the three rhythms of interest, i.e. alpha, beta and
gamma, to determine significant brain activity varia-
tions between baseline and each of the four types of
events. This analysis was performed for each subject
and electrode. In Tables 2-5 the percentage of subjects
that shows a significant variation in PSD (p−value <
0.05) was reported for each electrode and rhythm, for
CIDT, CMODT, CNR, and ERR events respectively.
These percentages were in general higher for alpha
and beta rhythms, in particular for CNR events (cor-
rect identification of non-target stimulus) and erro-
neous answers (ERR) and were more evident in the
frontal or parietal electrodes. In case of CIDT events
(correct identification of neutral basic target stimulus)
it seems that the variation of brain activity with re-
spect to the baseline was less evident.
5 CONCLUSIONS
Differences were found in the EEG patterns when rec-
ognizing neutral target faces versus faces modified
with biological plausible changes. In particular brain
activity changes were mainly found for alpha and beta
rhythms in frontal and parietal areas. The analysis
on this database can be useful as baseline for further
studies on people affected by congenital prosopag-
nosia or autism performing the same experiment, hav-
ing identified the brain activities variation in a healthy
population. This preliminary analysis can be strength-
ened to better distinguish between the four different
types of events, taking into account more features to
describe the EEG patterns, besides the PSD here con-
sidered. Moreover, to better compare results from
different subjects, proper data normalization has to
be addressed, such as subtracting the average power
recorded on the scalp or standardize the sensors volt-
age by using the z-score.
REFERENCES
Barragan-Jason, G., Cauchoix, M., and Barbeau, E. (2015).
The neural speed of familiar face recognition. Neu-
ropsychologia, 75:390–401.
Caharel, S., Collet, K., and Rossion, B. (2015). The
early visual encoding of a face (N170) is viewpoint-
dependent: a parametric ERP-adaptation study. Bio-
logical psychology, 106:18–27.
Cevikalp, H. and Triggs, B. (2010). Face recognition
based on image sets. In Computer Vision and Pat-
tern Recognition (CVPR), 2010 IEEE Conference on,
pages 2567–2573. IEEE.
Chaumon, M., Bishop, D. V., and Busch, N. A. (2015). A
practical guide to the selection of independent compo-
nents of the electroencephalogram for artifact correc-
tion. Journal of neuroscience methods, 250:47–63.
Comparetti, C., Ricciardelli, P., and Daini, R. (2011). Carat-
teristiche invarianti di un volto, espressioni emotive
ed espressioni non emotive: una tripla dissociazione?
Giornale Italiano di Psicologia, (1):215–224.
Daini, R., Comparetti, C. M., and Ricciardelli, P. (2014).
Behavioral dissociation between emotional and non-
emotional facial expressions in congenital prosopag-
nosia. Frontiers in human neuroscience, 8.
Delorme, A. and Makeig, S. (2004). Eeglab: an open source
toolbox for analysis of single-trial eeg dynamics in-
cluding independent component analysis. Journal of
neuroscience methods, 134(1):9–21.
Dickter, C. L. and Kieffaber, P. D. (2013). EEG methods for
the psychological sciences. Sage.
Itier, R. J. and Taylor, M. J. (2004). Face recognition mem-
ory and configural processing: a developmental ERP
study using upright, inverted, and contrast-reversed
faces. Journal of cognitive neuroscience, 16(3):487–
502.
Jung, T.-P., Makeig, S., Humphries, C., Lee, T.-W., Mck-
eown, M. J., Iragui, V., and Sejnowski, T. J. (2000).
Removing electroencephalographic artifacts by blind
source separation. Psychophysiology, 37(2):163–178.
Klados, M. A., Papadelis, C., Braun, C., and Bamidis, P. D.
(2011). REG-ICA: a hybrid methodology combining
blind source separation and regression techniques for
the rejection of ocular artifacts. Biomedical Signal
Processing and Control, 6(3):291–300.
Li, Y., Ma, S., Hu, Z., Chen, J., Su, G., and Dou, W.
(2015). Single trial eeg classification applied to a face
recognition experiment using different feature extrac-
tion methods. In Engineering in Medicine and Biol-
ogy Society (EMBC), 2015 37th Annual International
Conference of the IEEE, pages 7246–7249. IEEE.
Malaspina, M., Albonico, A., Daini, R., and Ricciardelli, P.
(2014). Elaborazione delle espressioni facciali, emo-
tive e non, in soggetti affetti da sindrome di asperger
o autismo ad alto funzionamento. Giornale italiano di
psicologia, 41(3):573–590.
¨
Ozbeyaz, A. and Arıca, S. (2018). Familiar/unfamiliar face
classification from eeg signals by utilizing pairwise
distant channels and distinctive time interval. Signal,
Image and Video Processing, pages 1–8.
Parketny, J., Towler, J., and Eimer, M. (2015). The activa-
tion of visual face memory and explicit face recog-
nition are delayed in developmental prosopagnosia.
Neuropsychologia, 75:538–547.
Rad
¨
untz, T., Scouten, J., Hochmuth, O., and Meffert, B.
(2015). EEG artifact elimination by extraction of
ICA-component features using image processing al-
gorithms. Journal of neuroscience methods, 243:84–
93.
EEG Data of Face Recognition in Case of Biological Compatible Changes: A Pilot Study on Healthy People
419