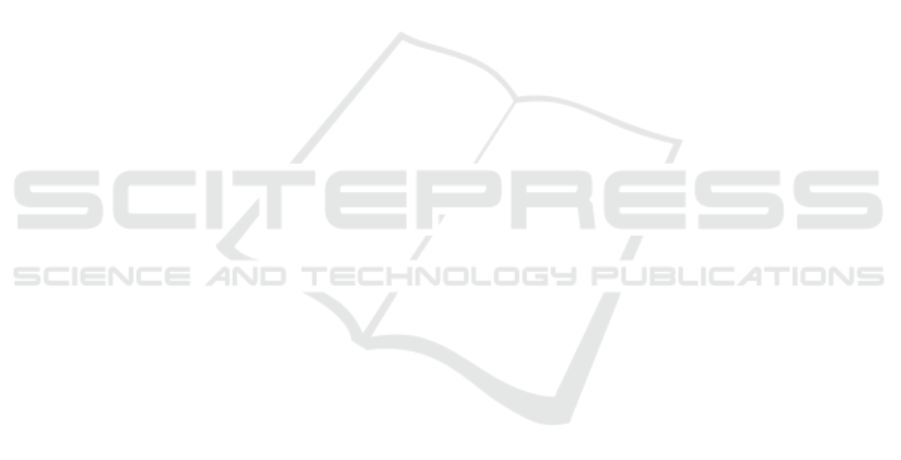
ACKNOWLEDGEMENTS
This research has been supported by the grant of Rus-
sian Ministry of Education and Science, agreement:
No14.606.21.0007, ID: RFMEFI60617X0007, "An-
droid Technics" company and Innopolis University.
REFERENCES
The tests of the hand-gesture-based control interface
with wearable glove system. Innopolis University:
https://youtu.be/f9dfKCUuUvY.
Afanasyev, I. and De Cecco, M. (2013). 3d gesture recogni-
tion by superquadrics. In International Conference on
Computer Vision Theory and Applications (VISAPP),
volume 2, pages 429–433. INSTICC.
Alfimtsev, A. (2008). Research and development of meth-
ods for dynamic gesture capture, tracking and recog-
nition. PhD thesis, Bauman Moscow State Technical
University.
Arkenbout, E. A., de Winter, J. C., and Breedveld, P. (2015).
Robust hand motion tracking through data fusion of
5dt data glove and nimble vr kinect camera measure-
ments. Sensors, 15(12):31644–31671.
Bansal, M., Saxena, S., Desale, D., and Jadhav, D. (2011).
Dynamic gesture recognition using hidden markov
model in static background. International Journal of
Computer Science Issues (IJCSI), 8(1).
Battaglia, E., Bianchi, M., Altobelli, A., Grioli, G., Cata-
lano, M. G., Serio, A., Santello, M., and Bicchi, A.
(2016). Thimblesense: a fingertip-wearable tactile
sensor for grasp analysis. IEEE Transactions on Hap-
tics, 9(1):121–133.
Bezdek, J. C., Ehrlich, R., and Full, W. (1984). Fcm: The
fuzzy c-means clustering algorithm. Computers &
Geosciences, 10(2-3):191–203.
Devyatkov, V. and Alfimtsev, A. (2007). Recognition of ma-
nipulative gestures. Bulletin of the Bauman Moscow
State Technical University. Series of «Instrument mak-
ing». In Russian., (3):56–74.
Dipietro, L., Sabatini, A. M., and Dario, P. (2008). A survey
of glove-based systems and their applications. IEEE
Transactions on Systems, Man, and Cybernetics, Part
C (Applications and Reviews), 38(4):461–482.
Dominio, F., Donadeo, M., and Zanuttigh, P. (2014). Com-
bining multiple depth-based descriptors for hand ges-
ture recognition. Pattern Recognition Letters, 50:101–
111.
Gawande, S. D. and Chopde, N. R. (2013). Neural network
based hand gesture recognition. International Journal
of Emerging Research in Management and Technol-
ogy, 3:2278–9359.
Gudhmundsson, S. A., Sveinsson, J. R., Pardas, M.,
Aanaes, H., and Larsen, R. (2010). Model-based hand
gesture tracking in tof image sequences. In Inter-
national Conference on Articulated Motion and De-
formable Objects (AMDO), pages 118–127. Springer.
Kenn, H., Van Megen, F., and Sugar, R. (2007). A glove-
based gesture interface for wearable computing appli-
cations. In 4th International Forum on Applied Wear-
able Computing (IFAWC), pages 1–10. VDE.
Kumar, P., Verma, J., and Prasad, S. (2012). Hand
data glove: A wearable real-time device for human-
computer interaction. International Journal of Ad-
vanced Science and Technology, 43:15–26.
Li, X. (2003). Gesture recognition based on fuzzy c-means
clustering algorithm. Department Of Computer Sci-
ence The University Of Tennessee Knoxville.
Luzhnica, G., Simon, J., Lex, E., and Pammer, V. (2016).
A sliding window approach to natural hand gesture
recognition using a custom data glove. In IEEE Sym-
posium on 3D User Interfaces (3DUI), pages 81–90.
Manresa, C., Varona, J., Mas, R., and Perales, F. J. (2005).
Hand tracking and gesture recognition for human-
computer interaction. ELCVIA Electronic Letters on
Computer Vision and Image Analysis, 5(3):96–104.
Mavridis, N., Giakoumidis, N., and Machado, E. L. (2012).
A novel evaluation framework for teleoperation and
a case study on natural human-arm-imitation through
motion capture. International Journal of Social
Robotics, 4(1):5–18.
Nayak, J., Naik, B., and Behera, H. (2015). Fuzzy c-
means (fcm) clustering algorithm: a decade review
from 2000 to 2014. In Computational intelligence in
data mining-volume 2, pages 133–149. Springer.
Park, Y., Lee, J., and Bae, J. (2015). Development of a wear-
able sensing glove for measuring the motion of fingers
using linear potentiometers and flexible wires. IEEE
Trans. on Industrial Informatics, 11(1):198–206.
Rautaray, S. S. and Agrawal, A. (2015). Vision based hand
gesture recognition for human computer interaction: a
survey. Artificial Intelligence Review, 43(1):1–54.
Ren, Z., Yuan, J., Meng, J., and Zhang, Z. (2013). Robust
part-based hand gesture recognition using kinect sen-
sor. IEEE Trans. on Multimedia, 15(5):1110–1120.
Suarez, J. and Murphy, R. R. (2012). Hand gesture recogni-
tion with depth images: A review. In 21st IEEE Inter-
national Symposium on Robot and Human Interactive
Communication (RO-MAN), pages 411–417. IEEE.
Suk, H.-I., Sin, B.-K., and Lee, S.-W. (2010). Hand ges-
ture recognition based on dynamic bayesian network
framework. Pattern recognition, 43(9):3059–3072.
Suryanarayan, P., Subramanian, A., and Mandalapu, D.
(2010). Dynamic hand pose recognition using depth
data. In Pattern Recognition (ICPR), 20th Interna-
tional Conference on, pages 3105–3108. IEEE.
Wachs, J., Kartoun, U., Stern, H., and Edan, Y. (2002).
Real-time hand gesture telerobotic system using fuzzy
c-means clustering. In Automation Congress, 2002
Proceedings of the 5th Biannual World, volume 13,
pages 403–409. IEEE.
Wachs, J. P., Kölsch, M., Stern, H., and Edan, Y. (2011).
Vision-based hand-gesture applications. Communica-
tions of the ACM, 54(2):60–71.
Zabulis, X., Baltzakis, H., and Argyros, A. (2009). Vision-
based hand gesture recognition for human-computer
interaction. 34:30.
The Hand-gesture-based Control Interface with Wearable Glove System
455