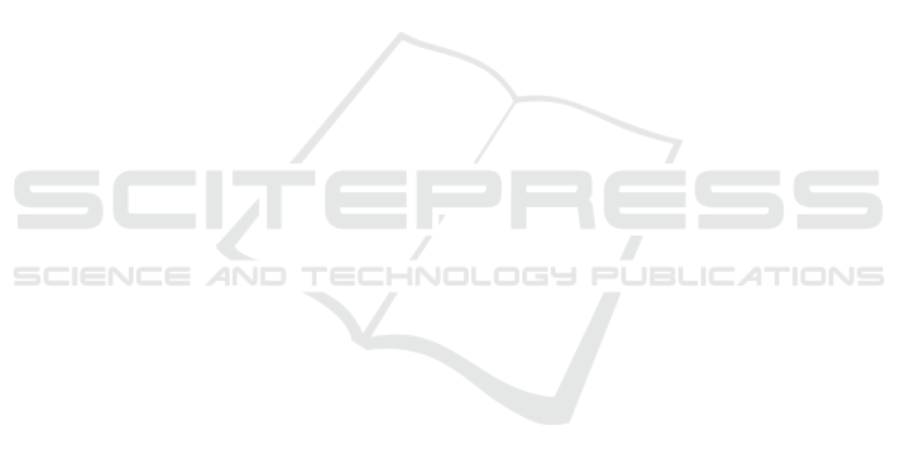
5 CONCLUSIONS
The results showed that the multi-model schema
MMSPGPC presented has a great potential of
application in uncertain systems. Even in presence
of significant noise background, the presented
approach has shown a reasonable result and could
be applied, as first approach, in tests with animals.
Others basic controllers, in order to attenuate the
delay effect, may be considered.
The simulation results showed that, changes
small in V can induce large changes in settling time
(T
s
). Where, for the patient 3, was obtained T
s
=
195s using V = 0,05 and, T
s
= 510s using V = 0,4,
with the Root Mean Square Error (RMSE) varying
less than 1% and small change in RMSU.
Moreover, the sensitivity analysis shows that for
high values de V, had a slower convergence of the
weight factors, thus an increase of T
s
. However for
low values de V, for example V = 0,05, has a faster
convergence, reducing T
s
, in most cases with small
change in RMSU and RMSE. Although, overly
faster convergence, for example V ≤ 0,01, impair
control, increasing the values of T
s
, RMSE and
RMSU.
In addition, the patient 3, which is closer to a
model in models bank (see Figure 2), presented the
lowest settling time (T
s
= 195s) among all simulated
patients (see Table 5). This suggests that, the
nearness of the patient to a model in models bank,
can provide a faster convergence.
In the future, robustness tests must be
implemented with the submission of the system to a
larger range of disturbances and parameters.
Comparative studies with other control algorithms,
such as robust adaptive control, they would also be
important to accomplish in order to obtain the
accuracy of the MMSPGPC presented
REFERENCES
Cavalcanti, A. L., Fontes, A. B., Maitelli, A. L., 2007.
Generalized Predictive Control Based in
Multivariable Bilinear Multimodel. Proceedings of
8th International IFAC Symposium on Dynamics and
Control of Process Systems, pp. 91-96, Cancún.
Cavalcanti, A. L., Silva, H. A., Maitelli, A. L., 2009.
Multiple Model GPC for Blood Pressure Control. XVI
Congresso Internacional De Ingenieria Eletrónica,
Eléctrica Y Computación, INTERCON, Arequipa,
Peru.
He, W. G., Kaufman, H., Roy, R., 1986. Multiple Model
Adaptive Control Procedure for Blood Pressure
Control. IEEE Transactions on Biomedical
Engineering, vol. BME 33, no. 1.
Kochar, M. S.,Woods, K. D., 1990. Controle da
hipertensão: para enfermeiras e demais profissionais
da saúde.. 2. ed. São Paulo: Andrei, 317 pp.
Maitelli, A. L.,Yoneyama, T., 1997.Suboptimal Dual
Adaptive Control for blood pressure management.
IEEE Transactions on Biomedical Engineering, vol.
44, no. 6.
Mion, Jr. D., CA. Machado, M. Gomes et al., 2016. VII
diretrizes brasileiras de hipertensão arterial. Brazilian
Journal of Hypertension, vol. 107, no. 3, pp. 2-19.
Silva, H. A., Cavalcanti A. L. O., Maitelli, A. L., 2010.
SPGPC Multi-Modelo para controle de Pressão
Arterial. XVIII Congresso Brasileiro de Automática,
CBA, Bonito, MS, Brazil.
Silva, H. A., 2010. Multi-Model Generalized Predictive
Controller Applied to Blood Pressure Control (in
portuguese). Master's Thesis, Department of
Electrical and Computer Engineering, Universidade
Federal do Rio Grande do Norte, Rio Grande do
Norte, Brazil.
Silva, H. A., Maitelli, A. L., Leão, C. P., Seabra, E. A.,
2015. Multiple Model SPGPC for Blood Pressure
Control. 12th International Conference on
Informatics in Control, Automation and Robotics,
ICINCO, Colmar, France.
Slate, J. B., 1980. Model-based design of a controller for
infusing nitroprusside during postsurgical
hypertension. PhD thesis, University of Wisconsin-
Madison.
Sowparnika, G. C., Thirumarimurugan, M., Sivakumar V.
M., 2017. Metaphorical analysis of Tuning rules for
PI and PID Controllers in modeling an Automatic
Drug Delivery System to control Mean Arterial Blood
Pressure. International Conference on Advanced
Computing and Communication Systems, ICACCS,
Coimbatore, India.
ICINCO 2018 - 15th International Conference on Informatics in Control, Automation and Robotics
516