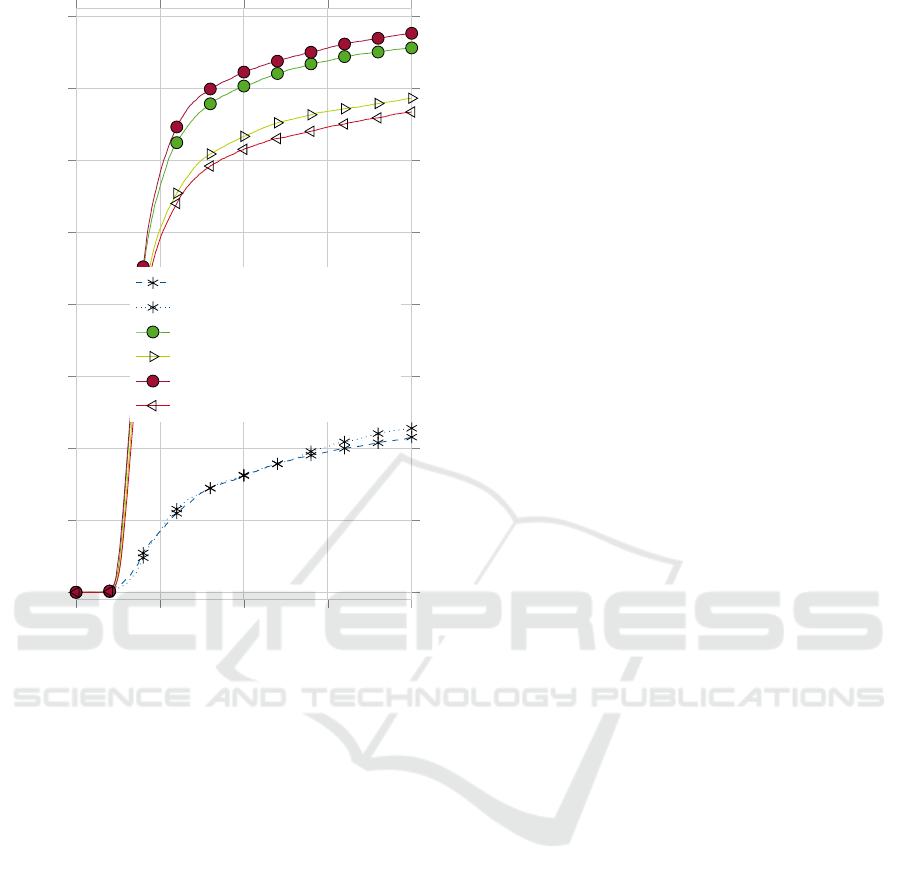
0
0.05
0.1
0.15
0.2
0
10
20
30
40
50
60
70
80
Error
Percentage of Images
image-wide (no descriptor)
pixel-wise (no descriptor)
image-wide, intensity only
image-wide, all channels
pixel-wise, intensity only
pixel-wise, all channels
Figure 3: Cumulative error distribution plot comparing
feature-based and regular AAMs, weight maps computed
on all channels or on the intensity channel only and global
vs. per-pixel intensity maps.
either image-wide or pixel-wise intensity map com-
putation on the fitting performance is small. There-
fore, image-wide intensity map computation is a vi-
able approach especially when additionally consider-
ing its drastically reduced computation time.
5 CONCLUSION
We have shown that AAM fitting precision can be
improved by combining robust cost functions with
feature-based AAMs. We presented and evaluated
two methods to estimate the standard deviations and
two methods to compute weight maps that are both
needed for robust fitting. An extensive evaluation
has shown that our proposed approach allows highly
improved tracking performance in video sequences
compared to regular AAMs.
REFERENCES
Alabort-i-Medina, J. and Zafeiriou, S. (2016). A Unified
Framework for Compositional Fitting of Active Ap-
pearance Models. International Journal of Computer
Vision, pages 1–39.
Antonakos, E., Alabort-i-Medina, J., Tzimiropoulos, G.,
and Zafeiriou, S. (2014). HOG Active Appearance
Models. In Image Processing (ICIP), 2014 IEEE In-
ternational Conference on, pages 224–228.
Antonakos, E., Alabort-i-Medina, J., Tzimiropoulos, G.,
and Zafeiriou, S. (2015). Feature-Based Lucas-
Kanade and Active Appearance Models. Image Pro-
cessing, IEEE Transactions on, 24:2617–2632.
Belhumeur, P. N., Jacobs, D. W., Kriegman, D. J., and Ku-
mar, N. (2013). Localizing parts of faces using a
consensus of exemplars. IEEE transactions on pat-
tern analysis and machine intelligence, 35(12):2930–
2940.
Cootes, T. F., Edwards, G. J., and Taylor, C. J. (1998). Ac-
tive appearance models. In European conference on
computer vision, pages 484–498. Springer.
Cootes, T. F., Edwards, G. J., and Taylor, C. J. (2001). Ac-
tive Appearance Models. IEEE Transactions on Pat-
tern Analysis & Machine Intelligence, pages 681–685.
Dalal, N. and Triggs, B. (2005). Histograms of oriented gra-
dients for human detection. In Computer Vision and
Pattern Recognition, 2005. CVPR 2005. IEEE Com-
puter Society Conference on, volume 1, pages 886–
893. IEEE.
G. S. Chrysos, E. Antonakos, S. Z. and Snape, P. (2015).
Offline deformable face tracking in arbitrary videos.
IEEE International Conference on Computer Vision
Workshops (ICCVW).
Gross, R., Matthews, I., and Baker, S. (2006). Active Ap-
pearance Models with Occlusion. Image and Vision
Computing, 24:593–604.
Huber, P. and Ronchetti, E. (1981). Robust Statistics. Wiley
and Sons.
Lowe, D. G. (1999). Object recognition from local scale-
invariant features. In Computer vision, 1999. The pro-
ceedings of the seventh IEEE international conference
on, volume 2, pages 1150–1157. Ieee.
Matthews, I. and Baker, S. (2004). Active Appearance
Models Revisited. International Journal of Computer
Vision, 60:135–164.
Sagonas, C., Antonakos, E., Tzimiropoulos, G., Zafeiriou,
S., and Pantic, M. (2016). 300 faces in-the-wild chal-
lenge: Database and results. Image and Vision Com-
puting, 47:3–18.
Shen, J., Zafeiriou, S., Chrysos, G. S., Kossaifi, J., Tz-
imiropoulos, G., and Pantic, M. (2015). The first facial
landmark tracking in-the-wild challenge: Benchmark
and results. IEEE International Conference on Com-
puter Vision Workshops (ICCVW).
Theobald, B.-J., Matthews, I., and Baker, S. (2006). Evalu-
ating Error Functions for Robust Active Appearance
Models. In Automatic Face and Gesture Recogni-
tion, 2006. FGR 2006. 7th International Conference
on, pages 149–154. IEEE.
Robust Statistics for Feature-based Active Appearance Models
425