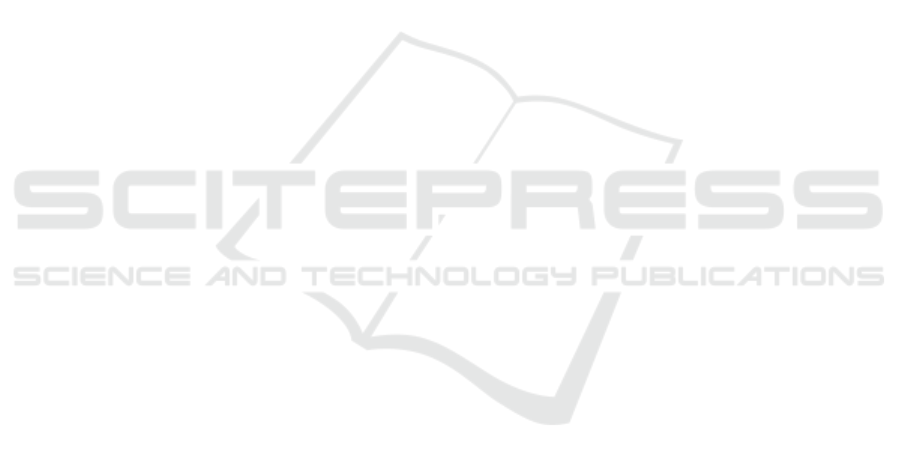
lity of the experts, which implies relatively high eco-
nomic costs, a certain degree of subjectivity associa-
ted with the sensory fatigue, and, in addition of the
arduous organisational task. This restrictions impose
that the quality control are reduced to certain lots.
As an alternative approach, the machine learning
techniques along with instrumental measures appear
as an intelligent solution to obtain a reliable estimate
of the sensory parameters of the food products.
In this work, a monitoring tool supported on a
cloud platform, has been presented. The core of
the ICatador tool is a suite of intelligent algorithms
(ANNs) which calculate organoleptic attributes esti-
mates using NIR spectrometry data from samples as
input.
To make possible that the ICatador monitoring
tool had all the ham and shoulders quality data avai-
lable, the main agents (quality manager, tasters, tas-
ting organizer, quality inspector) collaborate and ex-
change information from different points and, in ad-
dition, instrumental data are systematically incorpora-
ted. This collaborative model based on the data, will
allow to have a wide range of data coming from the
production process itself. In this way, the intelligent
algorithm suite can be tuned and adapted to the pro-
cess itself to avoid deviations.
Through the proposed approach, iberian ham ma-
nufacturing companies can carry out an intelligent
production based on the data, they can get that final
product gathers the same sensory characteristics fixed
in the production goals.
The Icatador platform that has been presented in
this paper combines the use of Information and Com-
munication Technologies with Artificial Intelligence
techniques such as ANNS. Therefore, it can be in-
cluded within the concept of Industry 4.0, and contri-
butes to the digitization of the industry. Its goals are
to improve efficiency, quality control times, flexibility
and enables a distributed responsibilities within of the
industrial sector.
ACKNOWLEDGEMENTS
This work has been supported by the General Founda-
tion of the University of Salamanca through the Plan
TCUE 2015-2017, co-financed by European Regional
Development Fund (ERDF) and the Castilla - Le
´
on
Council.
REFERENCES
(1994). ISO 11035: 1994:sensory analysis – identification
and selection of descriptors for establishing a sensory
profile by a multidimensional approach.
(2003). ISO 4121: 2003: Sensory analysis – guidelines for
the use of quantitative response scales.
(2014). Real decreto 4/2014, de 10 de enero, por el que se
aprueba la norma de calidad para la carne, el jam
´
on,
la paleta y la ca
˜
na de lomo ib
´
erico.
Balabin, R. M. and Safieva, R. Z. (2011). Biodiesel classi-
fication by base stock type (vegetable oil) using near
infrared spectroscopy data. Analytica Chimica Acta,
689(2):190–197.
Boccorh, R. K. and Paterson, A. (2002). An artificial neu-
ral network model for predicting flavour intensity in
blackcurrant concentrates. Food Quality and Prefe-
rence.
B
´
arcenas, P., Elortondo, F. P., and Albisu, M. (2003).
Sensory changes during ripening of raw ewes’ milk
cheese manufactured with and without the addition of
a starter culture. Food Science.
Cancilla, J. C., Wang, S. C., D
´
ıaz-Rodr
´
ıguez, P., Matute, G.,
Cancilla, J. D., Flynn, D., and Torrecilla, J. S. (2014).
Linking chemical parameters to sensory panel results
through neural networks to distinguish olive oil qua-
lity. Journal of Agricultural and Food Chemistry.
Della-Lucia, S. M. and Minim, L. A. (2010). Redes neurais
artificiais: fundamentos e aplicac¸
˜
oes. In UFV, V. E.,
editor, An
´
alise sensorial: estudos com consumidores,
chapter 9, pages 258–279. Springer-Verlag New York,
Inc.
Efendioglu, N. and Woitsch, R. (2017). A modelling met-
hod for digital service design and intellectual property
management towards Industry 4.0 caxman case. Inter-
national Conference on Serviceology.
Grassi, S. and Alamprese, C. (2017). Advances in NIR
spectroscopy applied to process analytical technology
in food industries. Current Opinion in Food Science.
Mourtzis, D. and Vlachou, E. (2016). Cloud-based cyber-
physical systems and quality of services. The TQM
Journal.
Murray, J. M., Delahunty, C., and Baxter, I. A. (2001).
Descriptive sensory analysis: past, present and future.
Food Research International.
Oio, K.-B., Lee, V.-H., Tan, G. W.-H., Hew, T.-S., and Hew,
J.-J. (2017). Cloud computing in manufacturing: The
next industrial revolution in malaysia? Expert Systems
with Applications.
Piggot, J. R. and Mowat, R. G. (1991). Sensory aspects of
maturation of cheddar cheese by descriptive analysis.
Journal of Sensory Studies.
Rathore, A. S. and Kapoor, G. (2017). Implementation of
quality by design towards processing of food products.
Preparative Biochemistry and Biotechnology.
Ruohomaa, H., Kantola, J., and Salminen, V. (2018). Va-
lue network development in Industry 4.0 environment.
Advances in Intelligent Systems and Computing.
Siegrist, M. and Cousin, M.-E. (2009). Expectations influ-
ence sensory experience in a wine tasting. Appetite.
ICINCO 2018 - 15th International Conference on Informatics in Control, Automation and Robotics
634