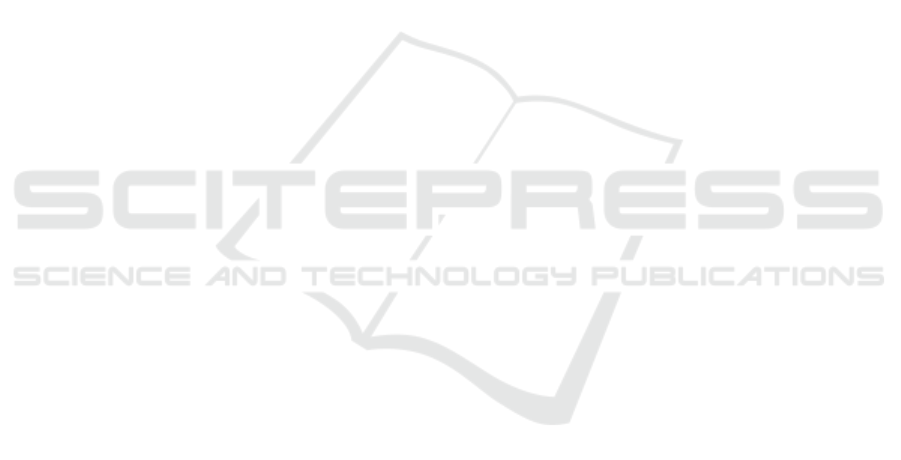
Bonferroni post-hoc test confirms that only the best
human-generated solution available in OpenML
performs significantly better than Auto-WEKA with
5 min running time. This means that there’s no
evidence arising from this experiment that confirms
that increasing the running time from 5 minutes to 15
or 30 minutes will improve the precision of the
results.
4 DISCUSSION OF RESULTS
Auto-WEKA’s default values, memory bound of
1GB and 15 minutes of overall time budget available,
suggests that a classification task running in a
commodity machine would achieve a reasonable
performance for suitable datasets. Experiments were
conducted using 18 datasets with a limited number of
observations and features.
The results show that even for such classification
tasks, Auto-WEKA with low computing time
performs poorly when compared to the best human-
generated solution available in OpenML. Also,
running times up to 30 min seem to achieve the same
performance. Either the selected classification tasks
are harder than expected or Auto-WEKA’s default
values should be revised so that the user is not misled.
However, from another point of view, one can
argue that the difference between the best human-
generated solution available in OpenML and Auto-
WEKA is less than 6 percentage points for 66% of the
datasets. That might be a good result for such a little
computation time, at least for a baseline precision.
5 CONCLUSIONS AND FUTURE
WORK
Auto-WEKA is a powerful tool that allows anyone to
fit a model to a dataset, giving, not only the best
model for a giving time budget but also an
attribute/feature selection approach. It is user-friendly
and multi-platform, both must have characteristics for
nowadays apps. While looking for a tool that can
provide a model without spending too much time,
Auto-WEKA may be the solution, despite the
precision of the chosen model may not be the best of
the best, it can give a solid start.
The results were promising, and one can
obviously expect Auto-WEKA to find a better model
using High-Performance Computers (HPC) clusters.
As far as we know there are no other studies on
the performance on Auto-WEKA in a commodity
machine using the default values. Increasing the
overall time budget available from 5 to 15 and 30
minutes didn’t improve significantly classifiers’
performance. Therefore, increasing only by a few
minutes doesn’t seem to have an impact on Auto-
WEKA’s performance. Thus, Auto-WEKA’s default
values (15 minutes and 1GB) might be misleading for
the user as there is no evidence that a good
classification performance is achieved with such low
running times and memory limit.
In this study, only the best human-generated
solution available in OpenML, that is related to huge
accumulated computation time performed signify-
cantly better than Auto-WEKA’s 5 minutes run.
There are some questions that we intend to
explore in the future. For each dataset how many
algorithms and hyperparameter configurations were
evaluated in the provided time budget. Which
characteristics of the dataset affect Auto-WEKA's
performance. What’s the effect of increasing running
time to more than 30 minutes.
As future work, we plan to run several
experiments in parallel using a HPC cluster. We
intend to understand how much running time is
needed for Auto-WEKA to converge to the best
human-generated solution.
ACKNOWLEDGEMENTS
This work was supported by Coimbra Polytechnic –
ISEC, Coimbra, Portugal. The authors are also
grateful to the reviewers who kindly suggested
improvements on the present paper.
REFERENCES
Almeida P., Gruenwald L. and Bernardino J. 2016.
Evaluating Open Source Data Mining Tools for
Business.In Proceedings of the 5th International
Conference on Data Management Technologies and
Applications - Volume 1: DATA, ISBN 978-989-758-
193-9, pages 87-94. DOI: 10.5220/0005939900870094
Almeida P., and Bernardino J., 2015. A Comprehensive
Overview of Open Source Big Data Platforms and
Frameworks. International Journal of Big Data, Vol. 2,
No. 3, pp. 1-19.
Demsar, J., 2006. Statistical Comparisons of Classifiers
over Multiple Data Sets. Journal of Machine Learning
Research, 7: 1-30.
Feurer, M., Klein, A., Eggensperger, K., Springenberg, J.,
Blum, M., Hutter, F., 2015. Efficient and Robust
Automated Machine Learning. In Advances in Neural
Benchmarking Auto-WEKA on a Commodity Machine
185