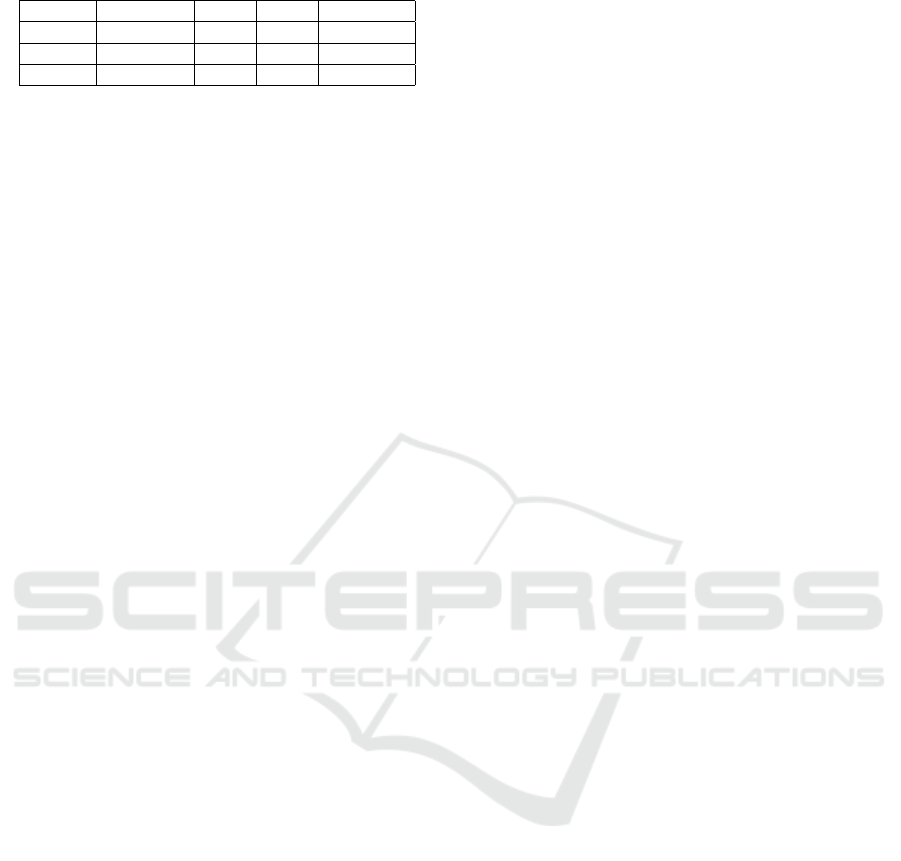
Table 6: Table of vehicles.
Model Brand Mass CdA Efficiency
Twizy Renault 460 0.40 0.8
i-MiEV Mitsubishi 1110 0.75 0.8
Leaf Nissan 1474 0.57 0.8
5 CONCLUSIONS
In this work we propose a new simulation framework
in which simulation environment is much closer to the
real world conditions. Here in the simulation sce-
nario it is possible to consider the altitude and re-
lated roads’ slope coefficient as well as classic pa-
rameters in the modeling of the EVs. Moreover, it
has been proved that the altitude heavily influences
the results and in particular the Energy Consumption
model. Therefore, for considering EV simulation en-
vironment and to evaluate overall behavior it is im-
portant to include the road slope coefficient during the
EV journey. In order to achieve more detailed simu-
lation results, in this work we propose a driver clas-
sification by introducing four driver classes. In fact,
drivers may influences performances in terms of trav-
eling time, road congestion and collisions because of
their behaviors. Moreover, in this work the model was
extending to classic vehicles for better monitoring en-
ergy consumption and emissions. In this way will be
possible to extend this work to design and the develop
of Intelligent Transportation System (ITS) policies in
a further work.
REFERENCES
(2018). Google maps api.
(2018). Open street map.
Bedogni, L., Bononi, L., D’Elia, A., Felice, M. D., Nicola,
M. D., and Cinotti, T. S. (2014). Driving without anx-
iety: A route planner service with range prediction for
the electric vehicles. In 2014 International Confer-
ence on Connected Vehicles and Expo (ICCVE), pages
199–206.
Bieker, L., Krajzewicz, D., Morra, A., Michelacci, C., and
Cartolano, F. (2015). Traffic simulation for all: A real
world traffic scenario from the city of bologna. In
Behrisch, M. and Weber, M., editors, Modeling Mo-
bility with Open Data, pages 47–60, Cham. Springer
International Publishing.
Fazio, P., De Rango, F., and Sottile, C. (2012). An on de-
mand interference aware routing protocol for vanets.
7.
Fazio, P., De Rango, F., Tropea, M., and Voznak, M. (2017).
Cell permanence time and mobility analysis in infras-
tructure networks: Analytical/statistical approaches
and their applications. Computers and Electrical En-
gineering, 64:506–523.
Fazio, P., Rango, F. D., and Sottile, C. (2011). A new in-
terference aware on demand routing protocol for ve-
hicular networks. In 2011 International Symposium
on Performance Evaluation of Computer Telecommu-
nication Systems, pages 98–103.
Gipps, P. (1981). A behavioural car-following model for
computer simulation. Transportation Research Part
B: Methodological, 15(2):105 – 111.
Hemmerle, P., Koller, M., Rehborn, H., Kerner, B. S., and
Schreckenberg, M. (2016). Fuel consumption in em-
pirical synchronised flow in urban traffic. IET Intelli-
gent Transport Systems, 10(2):122–129.
Knospe, W., Santen, L., Schadschneider, A., and Schreck-
enberg, M. (2000). Towards a realistic microscopic
description of highway traffic. Journal of Physics A:
Mathematical and General, 33(48):L477.
Krajzewicz, D., Erdmann, J., Behrisch, M., and Bieker-
Walz, L. (2012). Recent development and applications
of sumo - simulation of urban mobility.
Kurmis, M., Andziulis, A., Dzemydiene, D., Jakovlev, S.,
Voznak, M., and Gricius, G. (2015). Cooperative con-
text data acquisition and dissemination for situation
identification in vehicular communication networks.
Wireless Personal Communications, 85(1):49–62.
Kurmis, M., Voznak, M., Kucinskas, G., Drungilas, D.,
Lukosius, Z., Jakovlev, S., and Andziulis, A. (2017).
Development of method for service support manage-
ment in vehicular communication networks. 15, book-
title =.
Maia, R., Silva, M., Arajo, R., and Nunes, U. (2011). Elec-
tric vehicle simulator for energy consumption studies
in electric mobility systems. In 2011 IEEE Forum on
Integrated and Sustainable Transportation Systems,
pages 227–232.
Olascuaga, L. M. H., Garcia, J. A. R., and Pinzon, J. E. P.
(2015). Evs mass adoption in colombia ; a first ap-
proach model. In 2015 IEEE 15th International Con-
ference on Environment and Electrical Engineering
(EEEIC), pages 1285–1290.
Packer, N. (2011). A beginner s guide to energy and power.
Waraich, R. A., Charypar, D., Balmer, M., and Axhausen,
K. W. (2015). Performance Improvements for Large-
Scale Traffic Simulation in MATSim, pages 211–233.
Springer International Publishing, Cham.
Zhou, X., Tanvir, S., Lei, H., Taylor, J., Liu, B., Rouphail,
N. M., and Frey, H. C. (2015). Integrating a simplified
emission estimation model and mesoscopic dynamic
traffic simulator to efficiently evaluate emission im-
pacts of traffic management strategies. Transportation
Research Part D: Transport and Environment, 37:123
– 136.
A Vehicular Traffic Simulator Model for Evaluating Electrical Vehicle Performances in a Configurable Mobility Scenario
205