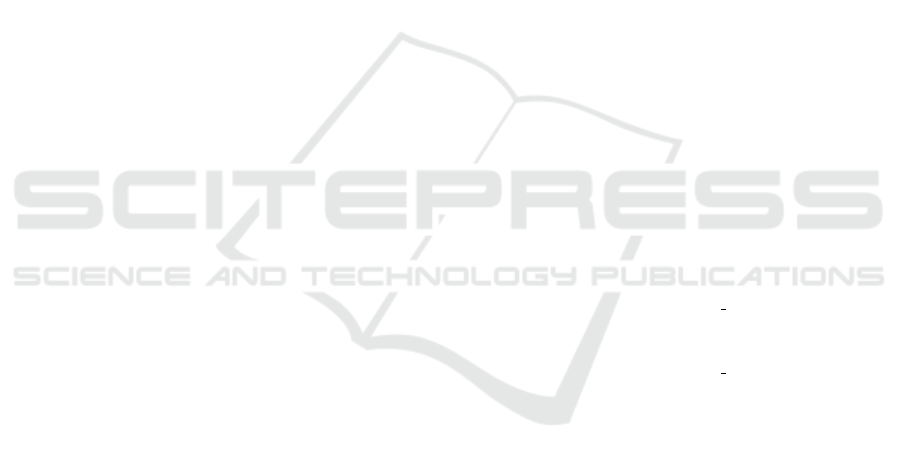
ducing the number of models required for detection—
while still retaining a usable degree of accuracy would
be a worthy result.
It would also be interesting to study this problem
from the perspective of malware type. That is, can
we achieve greater success if we restrict our attention
to a specific class of malware, such as botnets, tro-
jans, or worrms, for example? It seems plausible that
there will be more similarity between families that all
belong to the same class than families that span dif-
ferent classes. If so, we would expect to effectively
deal with larger numbers of families within a single
model.
Another basic problem is the need for an extre-
mely large, labeled, and publicly available malware
dataset. While the dataset used here is quite substan-
tial, with 18,495 samples, a dataset with a much larger
number of families (as well as sporadic non-family
malware samples) would be invaluable for research
of the type considered here, as well as for many other
research problems. We are currently in the process of
assembling just such a dataset.
REFERENCES
Adware:Win32/Lollipop (2018). Adware:Win32/Lollipop
threat description. https://www.microsoft.com/en-us/
wdsi/threats/malware-encyclopedia-description?
Name=Adware:Win32/Lollipop.
Bradley, A. P. (1997). The use of the area under the
roc curve in the evaluation of machine learning algo-
rithms. Pattern Recognition, 30(7):1145–1159.
Kaggle (2015). Kaggle: Microsoft malware classification
challenge (BIG 2015). https://www.kaggle.com/c/
malware-classification/ data.
Liangboonprakong, C. and Sornil, O. (2013). Classification
of malware families based on n-grams sequential pat-
tern features. In 2013 IEEE 8th Conference on Indus-
trial Electronics and Applications, ICIEA ’13, pages
777–782.
Malicia Project (2015). Malicia project. http://
malicia-project.com/.
Nappa, A., Rafique, M. Z., and Caballero, J. (2013). Dri-
ving in the cloud: An analysis of drive-by download
operations and abuse reporting. In Proceedings of the
10th Conference on Detection of Intrusions and Mal-
ware & Vulnerability Assessment, DIMVA 2013, pa-
ges 1–20.
Norton (2018). Norton Security Center — Malware.
https://us.norton.com/internetsecurity-malware.html.
Pearson, K. (1900). On the criterion that a given system of
deviations from the probable in the case of a correlated
system of variables is such that it can be reasonably
supposed to have arisen from random sampling. The
London, Edinburgh, and Dublin Philosophical Maga-
zine and Journal of Science, 50(302):157–175.
Plackett, R. L. (1983). Karl Pearson and the chi-squared
test. International Statistical Review / Revue Interna-
tionale de Statistique, 51(1):59–72.
Raff, E. et al. (2016). An investigation of byte n-gram fea-
tures for malware classification. Journal of Computer
Virology and Hacking Techniques, pages 1–20.
Reddy, D. K. S. and Pujari, A. K. (2006). N-gram analysis
for computer virus detection. Journal in Computer
Virology, 2(3):231–239.
Shabtai, A., Moskovitch, R., Elovici, Y., and Glezer, C.
(2009). Detection of malicious code by applying ma-
chine learning classifiers on static features: A state-of-
the-art survey. Information Security Technical Report,
14(1):16 – 29.
Singh, T., Troia, F. D., Visaggio, C. A., Austin, T. H., and
Stamp, M. (2016). Support vector machines and mal-
ware detection. Journal of Computer Virology and
Hacking Techniques, 12(4):203–212.
Stamp, M. (2017). Introduction to Machine Learning with
Applications in Information Security. Chapman and
Hall/CRC, Boca Raton.
Symantec (2017). Symantec internet security threat report.
https://www.symantec.com/content/dam/symantec/
docs/reports/istr-22-2017-en.pdf.
Tabish, S. M., Shafiq, M. Z., and Farooq, M. (2009). Mal-
ware detection using statistical analysis of byte-level
file content. In Proceedings of the ACM SIGKDD
Workshop on CyberSecurity and Intelligence Informa-
tics, CSI-KDD ’09, pages 23–31, New York.
Trojan:Win32/Gatak (2018). Trojan:Win32/Gatak threat
description. https://www.microsoft.com/en-us/wdsi/
threats/malware-encyclopedia-description?
Name=Trojan%3AWin32%2FGatak.
Trojan.Zbot (2010). Trojan.Zbot. http://
www.symantec.com/security response/writeup.jsp?
docid=2010-011016-3514-99.
Trojan.Zeroaccess (2011). Trojan.Zeroaccess. https://
www.symantec.com/security response/writeup.jsp?
docid=2011-071314-0410-99.
VirTool:Win32/Obfuscator.ACY (2018). VirTool:Win32/
Obfuscator.ACY threat description. https://
www.microsoft.com/en-us/wdsi/threats/malware-
encyclopedia-description?Name=VirTool:Win32/
Obfuscator.ACY.
Win32/Kelihos (2018). Win32/Kelihos threat descrip-
tion. https://www.microsoft.com/en-us/wdsi/threats/
malware-encyclopedia-description?Name=Win32
%2fKelihos.
Win32/Ramnit (2018). Win32/Ramnit threat descrip-
tion. https://www.microsoft.com/en-us/wdsi/threats/
malware-encyclopedia-description?Name=Win32/
Ramnit.
Win32/Winwebsec (2017). Win32/Winwebsec. https://
www.microsoft.com/security/portal/threat/encyclopedia/
entry.aspx?Name=Win32%2fWinwebsec.
Wong, W. and Stamp, M. (2006). Hunting for metamorphic
engines. Journal in Computer Virology, 2(3):211–229.
BASS 2018 - International Workshop on Behavioral Analysis for System Security
450