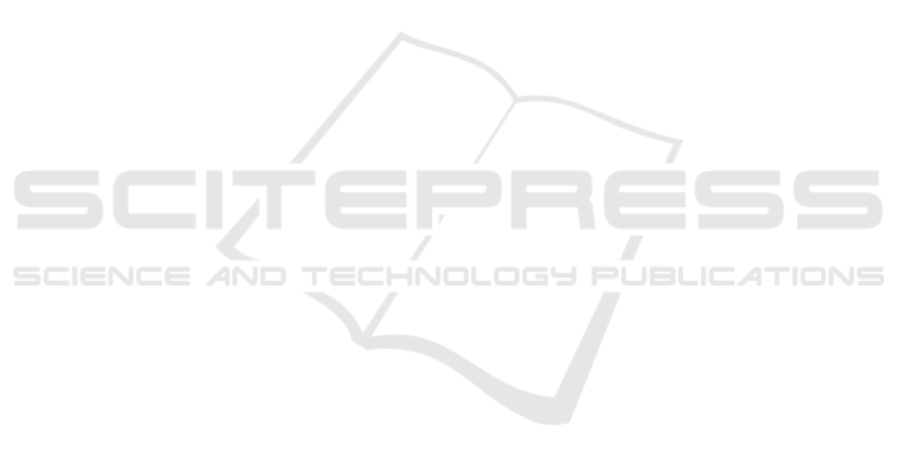
(3285+1200), that is a long consecutive test period of
decreasing of the inverted DJIA. This highlights that
the solution has also learned when it is better to re-
frain from trading.
5 CONCLUSION
In this work, we have analysed the predictive capa-
bilities on the DJIA index of a simple solution based
on novel deep recurrent neural networks, which in se-
veral research areas have shown superior capabilities
of detecting long term dependencies in sequences of
data, such as in speech recognition and in text under-
standing. The aim of our work was to move away
from the latest complex trends, in terms of stock mar-
ket prediction based on the use of non-structured data
(tweets, financial news, etc.), in order to focus more
simply on the stock time series. From this viewpoint
the work follows the philosophy of the ARIMA ap-
proach proposed in 1970 by Box and Jenkins but ex-
perimenting advanced approaches.
Our tests have shown that the proposed solution is
able to obtain a prediction accuracy of about 83% and
a profit of more than 5 times the initial capital, out-
performing the state-of-the-art. The tests have also
shown how the predictions benefit from a lower va-
riability compared to that obtained with an approach
operating random choices.
The work can be extended to a scenario of paral-
lel trading with multiple stocks, also investigating and
exploiting possible correlations among different mar-
ket indexes. Another possible improvement is to pre-
dict the best trading action according to forecasts of
stock price movements referred to two or more days
in the future. This strategy may lead to the identifica-
tion of additional recurring patterns able to exploit the
time lag in the reactivity of stock market as supposed
in (LeBaron et al., 1999).
REFERENCES
Abe, M. and Nakayama, H. (2018). Deep learning for fore-
casting stock returns in the cross-section. arXiv pre-
print arXiv:1801.01777.
Akita, R., Yoshihara, A., Matsubara, T., and Uehara, K.
(2016). Deep learning for stock prediction using nu-
merical and textual information. In Computer and In-
formation Science (ICIS), 2016 IEEE/ACIS 15th In-
ternational Conference on, pages 1–6. IEEE.
Atsalakis, G. S. and Valavanis, K. P. (2009a). Forecasting
stock market short-term trends using a neuro-fuzzy
based methodology. Expert systems with Applicati-
ons, 36(7):10696–10707.
Atsalakis, G. S. and Valavanis, K. P. (2009b). Surveying
stock market forecasting techniques–part ii: Soft com-
puting methods. Expert Systems with Applications,
36(3):5932–5941.
Bao, W., Yue, J., and Rao, Y. (2017). A deep learning
framework for financial time series using stacked au-
toencoders and long-short term memory. PLOS ONE,
12(7):1–24.
Bollen, J., Mao, H., and Zeng, X. (2011). Twitter mood
predicts the stock market. Journal of computational
science, 2(1):1–8.
Box, G. E. P. and Jenkins, G. (1970). Time Series Analy-
sis, Forecasting and Control. Holden-Day, Inc., San
Francisco, CA, USA.
Cao, Q., Leggio, K. B., and Schniederjans, M. J. (2005).
A comparison between fama and french’s model and
artificial neural networks in predicting the chinese
stock market. Computers & Operations Research,
32(10):2499–2512.
Cho, K., van Merri
¨
enboer, B., G
¨
ulc¸ehre, C¸ ., Bahdanau, D.,
Bougares, F., Schwenk, H., and Bengio, Y. (2014).
Learning phrase representations using rnn encoder–
decoder for statistical machine translation. In Pro-
ceedings of the 2014 Conference on Empirical Met-
hods in Natural Language Processing (EMNLP), pa-
ges 1724–1734, Doha, Qatar. Association for Compu-
tational Linguistics.
di Lena, P., Domeniconi, G., Margara, L., and Moro, G.
(2015). GOTA: GO term annotation of biomedical li-
terature. BMC Bioinformatics, 16:346:1–346:13.
Ding, X., Zhang, Y., Liu, T., and Duan, J. (2015). Deep
learning for event-driven stock prediction. In Ijcai,
pages 2327–2333.
Domeniconi, G., Masseroli, M., Moro, G., and Pinoli, P.
(2016). Cross-organism learning method to discover
new gene functionalities. Computer Methods and Pro-
grams in Biomedicine, 126:20–34.
Domeniconi, G., Moro, G., Pagliarani, A., and Pasolini, R.
(2017a). Learning to predict the stock market dow
jones index detecting and mining relevant tweets.
Domeniconi, G., Moro, G., Pagliarani, A., and Pasolini, R.
(2017b). On deep learning in cross-domain sentiment
classification. In Proceedings of the 9th Internatio-
nal Joint Conference on Knowledge Discovery, Know-
ledge Engineering and Knowledge Management - (Vo-
lume 1), Funchal, Madeira, Portugal, November 1-3,
2017., pages 50–60.
Esteva, A., Kuprel, B., Novoa, R. A., Ko, J., Swetter, S. M.,
Blau, H. M., and Thrun, S. (2017). Dermatologist-
level classification of skin cancer with deep neural net-
works. Nature, 542(7639):115–118.
Fama, E. F. (1970). Efficient capital markets: A review of
theory and empirical work. The journal of Finance,
25(2):383–417.
Fischer, T. and Krauss, C. (2017). Deep learning with long
short-term memory networks for financial market pre-
dictions. European Journal of Operational Research.
Fisher, I. E., Garnsey, M. R., and Hughes, M. E. (2016). Na-
tural language processing in accounting, auditing and
finance: A synthesis of the literature with a roadmap
DATA 2018 - 7th International Conference on Data Science, Technology and Applications
152