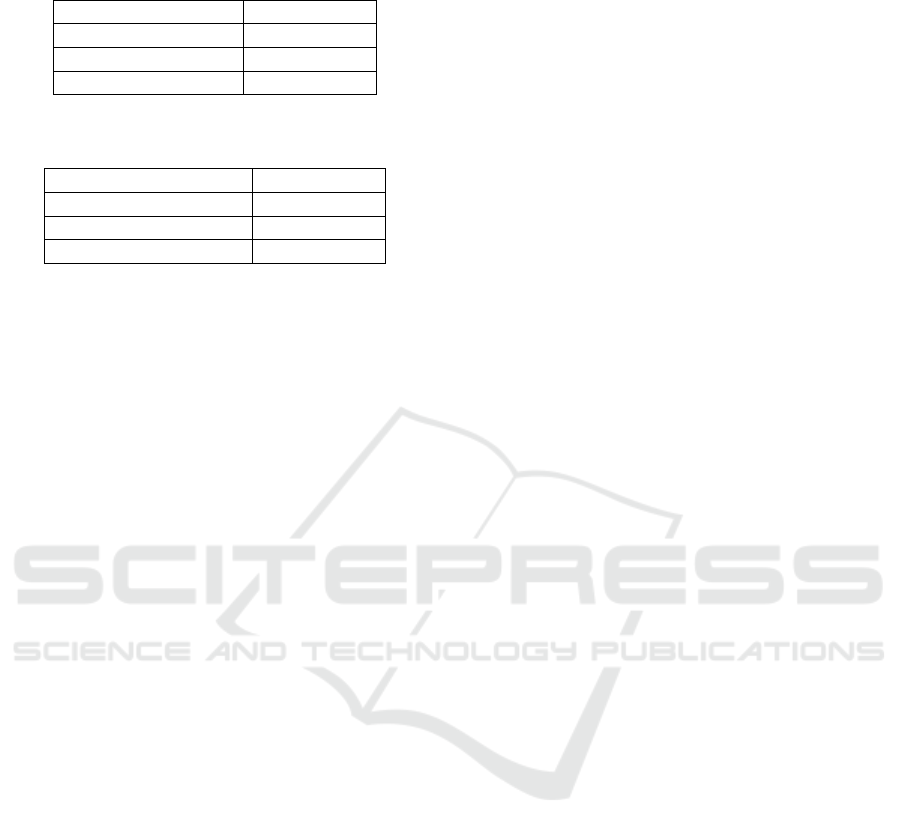
Table 3: Comparison of our results with those found in lite-
rature for Flexion/MVF ratios in healthy patients.
Flexion/MVF
Our results 3.07 ± 0.67
(Watson et al., 1997) 13.98 ± 11
(Neblett et al., 2013) 15.1 ± 7.7
Table 4: Comparison of our results with those found in lite-
rature for Flexion/MVF ratios in LBP patients.
Flexion/MVF
Our results 1.22 ± 0.33
(Watson et al., 1997) 2.72 ± 2.7
(Alschuler et al., 2009) 0.19 ± 0.47
5 CONCLUSION
Our algorithm helps automate the evaluation of the
athletes without any knowledge of sEMG nor of LFT
and our results agree with other studies. Our results
prove the validity of the algorithm for computing ra-
tios based on those presented in literature. This tool
would help sport specialists to evaluate their sports-
men and observe an objective progress over their re-
habilitation with little preprocessing that can be per-
formed with sEMG analysis software (Banos et al.,
2015).
Our algorithm could increase the objective asses-
sment during the sportsmen injury time as well as
a tool during injury free periods to evaluate if LBP
could be a plausible consequence of an overload trai-
ning. Automatically computed ratios can served as a
guide to perform changes in the recovery plan, decre-
asing injury’s time.
REFERENCES
(2017). Low Back Pain Fact Sheet. National Institute of
Neurological Disorders and Stroke.
Al Zaman, A., Sharmin, T., Khan, M. A. A., and Ferdjallah,
M. (2007). Muscle fatigue analysis in young adults
at different mvc levels using emg metrics. In Sout-
heastCon, 2007. Proceedings. IEEE, pages 390–394.
IEEE.
Alschuler, K. N., Neblett, R., Wiggert, E., Haig, A. J.,
and Geisser, M. E. (2009). Flexion-relaxation and
clinical features associated with chronic low back
pain: a comparison of different methods of quanti-
fying flexion-relaxation. The Clinical journal of pain,
25(9):760–766.
Banos, O., Damas, M., Pomares, H., Prieto, A., and Ro-
jas, I. (2012). Daily living activity recognition based
on statistical feature quality group selection. Expert
Systems with Applications, 39(9):8013–8021.
Banos, O., Damas, M., Pomares, H., Rojas, F., Delgado-
Marquez, B., and Valenzuela, O. (2013). Human acti-
vity recognition based on a sensor weighting hierar-
chical classifier. Soft Computing, 17(2):333–343.
Banos, O., Moral-Munoz, J. A., Diaz-Reyes, I., Arroyo-
Morales, M., Damas, M., Herrera-Viedma, E., Hong,
C. S., Lee, S., Pomares, H., Rojas, I., et al. (2015).
mdurance: a novel mobile health system to support
trunk endurance assessment. Sensors, 15(6):13159–
13183.
Banos, O., Toth, M. A., Damas, M., Pomares, H., and Ro-
jas, I. (2014). Dealing with the effects of sensor dis-
placement in wearable activity recognition. Sensors,
14(6):9995–10023.
Colloca, C. J. and Hinrichs, R. N. (2005). The biomechani-
cal and clinical significance of the lumbar erector spi-
nae flexion-relaxation phenomenon: a review of lite-
rature. Journal of Manipulative & Physiological Ther-
apeutics, 28(8):623–631.
Desai, H. S. and Bisen, R. S. (2017). Lumbar flexion re-
laxation phenomenon in the patients with acute and
subacute mechanical low back pain and normal sub-
jects. International Journal of Research in Medical
Sciences, 5(3):1011–1014.
Harrison, H. (1999). Quaternions and rotation sequences: a
primer with applications to orbits, aerospace and vir-
tual reality, kuipers jb, princeton university press, 41
william street, princeton, nj 08540, usa. 1999. 372pp.
illustrated.£ 35.00. isbn 0-691-05872-5. The Aeronau-
tical Journal, 103(1021):175–175.
Kim, G., Ahad, M. A., Ferdjallah, M., and Harris, G. F.
(2007). Correlation of muscle fatigue indices between
intramuscular and surface emg signals. In Southeast-
Con, 2007. Proceedings. IEEE, pages 378–382. IEEE.
Kujala, U. M., Salminen, J. J., Taimela, S., Oksanen, A.,
and Jaakkola, L. (1992). Subject characteristics and
low back pain in young athletes and nonathletes. Me-
dicine and science in sports and exercise, 24(6):627–
632.
Lin, H.-C., Chiang, S.-Y., Lee, K., and Kan, Y.-C. (2015).
An activity recognition model using inertial sensor no-
des in a wireless sensor network for frozen shoulder
rehabilitation exercises. Sensors, 15(1):2181–2204.
Luinge, H. J., Veltink, P. H., and Baten, C. T. (2007). Am-
bulatory measurement of arm orientation. Journal of
biomechanics, 40(1):78–85.
Madgwick, S. O., Harrison, A. J., and Vaidyanathan, R.
(2011). Estimation of imu and marg orientation using
a gradient descent algorithm. In Rehabilitation Ro-
botics (ICORR), 2011 IEEE International Conference
on, pages 1–7. IEEE.
Mannini, A., Intille, S. S., Rosenberger, M., Sabatini,
A. M., and Haskell, W. (2013). Activity recogni-
tion using a single accelerometer placed at the wrist
or ankle. Medicine and science in sports and exercise,
45(11):2193.
Neblett, R., Brede, E., Mayer, T. G., and Gatchel, R. J.
(2013). What is the best surface emg measure of lum-
bar flexion-relaxation for distinguishing chronic low
back pain patients from pain-free controls? The Cli-
nical journal of pain, 29(4):334.
icSPORTS 2018 - 6th International Congress on Sport Sciences Research and Technology Support
38