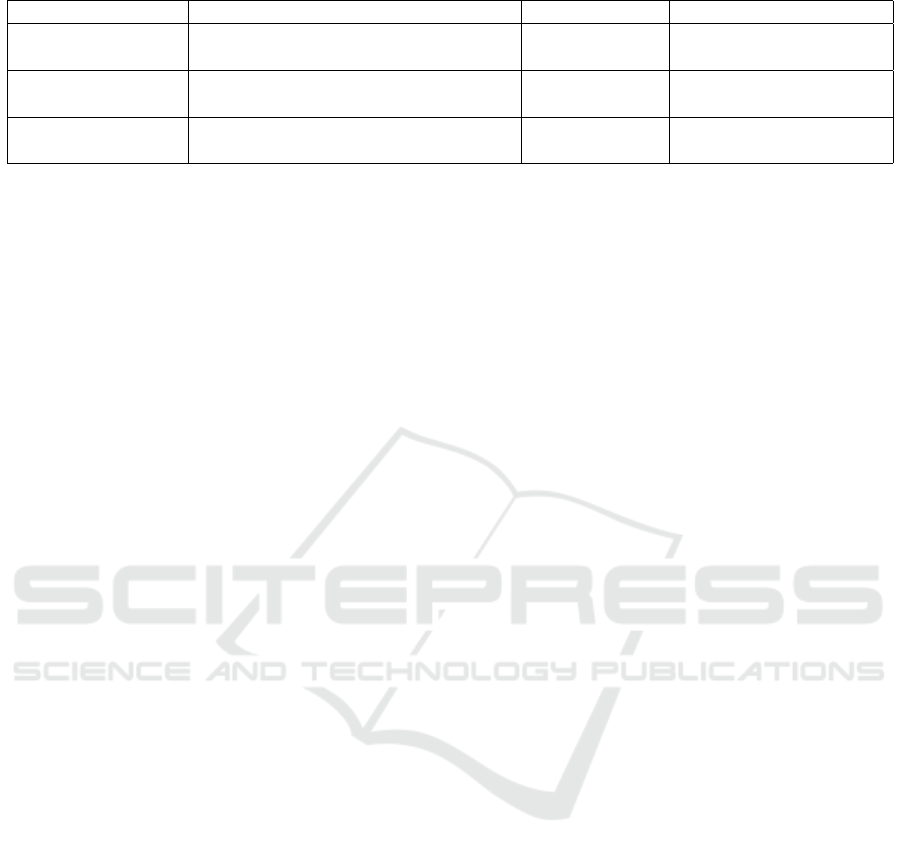
Table 4: Relationship between task type and intellectual concentration states.
Task Type Details Cognitive Load Concentration States
Task A (Slow Pace)
Solve the Receipt-Classification Task
Middle Somewhat Concentrated
as Slow as Possible
Task B (High Pace)
Solve the Reccipt-Classification Task
High Very Concentrated
as Fast as Possible
Task C (Click)
Conduct the Click Task (Do Not
Low Not Concentrated at All
Solve the Receipt-Classification Task )
4 RESULT
In this experiment, six out of the 31 participants were
excluded from the later analysis as invalid data.The
reasons why there were invalid data was because their
heart beat data was lost due to the failure or irregu-
lar power off of the ECG measuring device, or pupil
diameter data was lost due to their dozing during mea-
surement. Finally 25 participants excluding the above
six were analyzed. 10 out of 25 participants were in-
terrupted half way because an error occurred in which
the response of the task was interrupted at the transi-
tion of the task screen, and their measurements re-
started from just before the error occurred. However,
since there was no data loss of these participants, their
measured data were included in the later analysis.
4.1 Evaluation Method of Estimation
Accuracy
60 second pupil diameter data and heart rate data ex-
tracted as a frame and the frames were shifted every
30 seconds, and the average value of each frame ex-
cluding the beginning 30 seconds was taken as the ex-
tracted feature value. In this experiment, the total time
of each task was set to 5 minutes, so the number of
feature values per variable was 24. The explanatory
variables are the left pupil diameter, the right pupil
diameter, heart rate, LF, HF, LF / HF in total, and the
objective variable is the type of tasks described in Ta-
ble 2. The ratio of the number of the objective varia-
bles that can be correctly estimated, that is, the correct
estimation rate, is evaluated as estimation accuracy in
this method.
Next, an evaluation method of estimation accu-
racy will be described. First, for all 24 training data,
all classification learning methods shown in Table 2
were applied. Then, the highest generalization per-
formance for each participant was defined as a trai-
ned model of the participant. Finally, an unknown
test data of the participant was estimated based on the
model and the estimation accuracy was calculated. In
this experiment total 2 sets of similar protocols were
carried out. Thus, estimation accuracy in this study
was defined as average value of accuracy when set-
ting set 1 as training data, set 2 as test data and accu-
racy when setting set 1 as test data, set 2 as training
data. The cross validation method was used to evalu-
ate the generalization performance of the trained mo-
del. Generalization performance of trained models of
all valid data was 91.1% in average. The most applied
classification method was linear classifier, and at the
next point was SVM.
4.2 Estimation Accuracy of Intellectual
Concentration States and
Disccusion on Estimated Error
By using the estimation method discribed in 4.1
section, the estimation accuracy of all valid test data
wa 57.3% in average. It was significantly higher than
the random expected value (p < 0.001). However, the
percentage of correct answer was depending on the
individual participants. In the following, giving re-
presentative examples of participants who were high
in correct answer rate and those who were low, and
discuss the differences in estimation results.
First, considering the participant who was estima-
ted with the highest accuracy with a correct answer
rate of 88%. Figure 5 shows a scatter diagram for each
physiological index where the horizontal axis shows
those of set1 while the vertical axis shows those of
set2 for each of the 8 feature values in each of the
three intellectual concentration states. If the same fe-
ature value appeared between set1 and set2, a feature
point is displayed on the straight line y = x. In the case
of this participant, obvious differences of pupil dia-
meters and heart rate were shown between three con-
centration states, where they increased when the cog-
nitive load of the task increased. Regarding LF and
HF, although there was no such obvious differences
between concentration states, there was a tendency to
show the smallest value in task B: very concentrated
status. From the above results, in case of this parti-
cipant it seems that the difference in intellectual con-
centration state due to the task difference appeared in
CHIRA 2018 - 2nd International Conference on Computer-Human Interaction Research and Applications
66