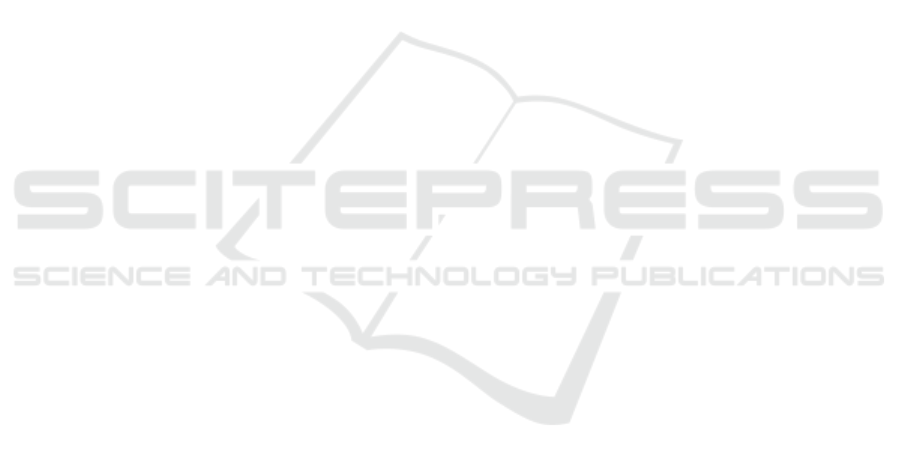
6 CONCLUSION AND FUTURE
WORK
In this work we showed that routinely collected
EMR data has clinical utility in predicting future RA
flare probability in patients treated with biological
DMARDs in daily practice. Several predictive
machine learning models were developed and tested
with the best one having an AUROC of about 80%.
This relatively good predictive power could enable
decision support for physicians and patients to guide
tapering of bDMARDs once low disease activity or
remission is reached. This offers potential to lower
the risk of adverse events, meet patients’ desire for
drug holidays, lower the overall costs for expensive
biological drug treatment and retain good control of
disease activity in RA patients.
In the future we plan to validate, calibrate and
test the generalizability of developed models and
approaches using external patient data, coming from
different clinics.
ACKNOWLEDGEMENTS
This project was made possible by the Applied Data
Analytics in Medicine (ADAM) programme of the
University Medical Center Utrecht, Utrecht, the
Netherlands. The authors would like to specifically
acknowledge ir. Hyleco H. Nauta and Harry Pijl,
MBA for their organizational support. Additionally,
the authors would like to acknowledge Arjan
Westrik from Accenture as well as Heike Bollmann
and Bas Idzenga from Siemens Healthineers for their
overall support to the ADAM-RA Project. We are
grateful to rheumatologists of the UMC Utrecht for
their valuable input regarding clinical definitions
and suggestions for implementation during the
project. Moreover, we thank the pharmacy of the
UMC Utrecht for their valuable insights in the
process of medication handling.
REFERENCES
World Health Organization, 2018. www.who.int. Online
resource.
Smolen, J. S., Aletaha, D., McInnes, I. B., 2016.
Rheumatoid arthritis. In Lancet, volume 388, pages
2023-2038.
RheumatologyAdvisor, 2018. www.rheumatologyadvisor.
com. Online resource.
Bouman, C. A. M., van Herwaarden, N., van den Hoogen,
F. H. J., et al., 2017. Long-term outcomes after disease
activity-guided dose reduction of TNF inhibition in
rheumatoid arthritis: 3 year data of the DRESS study –
a randomised controlled pragmatic non-inferiority
strategy trial. In Ann Rheum Dis, volume 76, pages
1716–1722.
Singh, J. A., Wells, G. A., Christensen, R., et al., 2011.
Adverse effects of biologics: a network meta-analysis
and cochrane overview. In Cochrane Database Syst
Rev., volume 2.
Singh, J. A., Cameron, C., Noorbaloochi, S., et al., 2015.
Risk of serious infection in biological treatment of
patients with rheumatoid arthritis: a systematic review
and meta-analysis. In Lancet, pages 258-265.
Edwards, C. J., Fautrel, B., Schulze-Koops, H., et al.,
2017. Dosing down with biologic therapies: a
systematic review and clinicians’ perspective. In
Rheumatology, volume 56(11), pages 1847-1856.
Verhoef, L. M., Tweehuysen, L., Hulscher, M. E., et al.,
2017. bDMARD Dose Reduction in Rheumatoid
Arthritis: A Narrative Review with Systematic
Literature Search. In Rheumatology and Therapy,
volume 4(1), pages 1-24.
Shiezadeh, Z., Sajedi, H., Aflakie, E., 2015. Diagnosis of
Rheumatoid Arthritis Using an Ensemble Learning
Approach. In Intl. Conf. on Advanced Information
Technologies and Applications, pages 139-148.
Lin, C., Karlson, E., W., Canhao, H., et al., 2013.
Automatic prediction of rheumatoid arthritis disease
activity from the electronic medical records. In PLoS
ONE, 8(8): e69932.
Prevoo, M. L. L., van't Hof, M. A., Kuper, H. H., et al.,
1995. Modified disease activity scores that include
twenty-eight-joint counts. In Arthritis Rheum, volume
38, pages 44-48.
Saleem, B., Brown, A., K., Quinn, M., et al., 2012. Can
flare be predicted in DMARD treated RA patients in
remission, and is it important? A cohort study In
Annals of the Rheumatic Diseases, 71:1316-1321.
Hastie, T., Tibshirani, R., Friedman, J., 2009. The
Elements of Statistical Learning, Springer, Stanford,
CA, 2
nd
edition.
Fawcett, T., 2006. An introduction to ROC analysis. In
Patt. Rec. Letters, volume 27, pages 861-874.
Niculescu-Mizil, A., Caruana, R., 2005. Predicting Good
Probabilities With Supervised Learning. In Proc. Intl.
Conf. on Machine Learning, pages 625-632.
DATA 2018 - 7th International Conference on Data Science, Technology and Applications
192