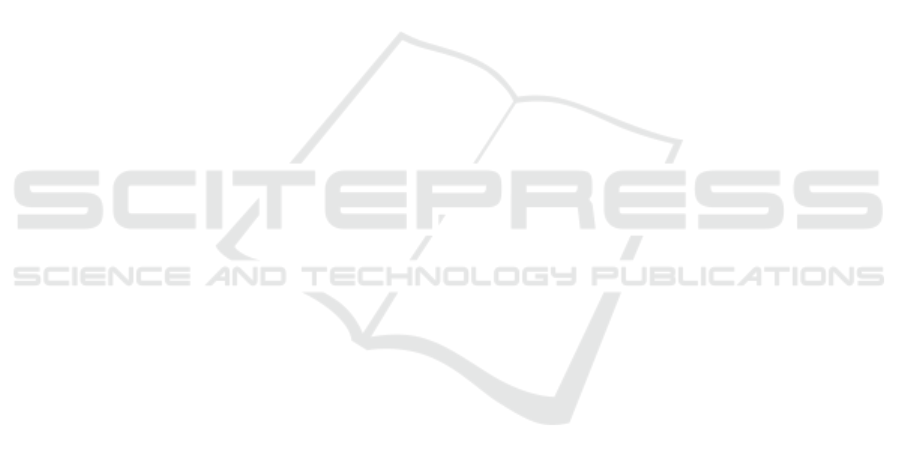
rameter showed that this parameter in most cases is
generated with an error below 17%, with the smallest
error observed in P
1
for Seq. 2 (δ = 1%), and the lar-
gest in P
2
for Seq. 1 (δ = 23%).
The presented method also allows for an observa-
tion of the analysed parameter changes over time. Fi-
gure 5 presents the parameters of hurdle clearance as
a function of time (frames). The key points of hurdle
clearance have been marked on the charts (P
1
—P
3
).
The charts present the mean value of the parameters
for 10 repetitions of the algorithm, additionally a mo-
ving average filter with the window equal to three was
used. The analysis showed that the hurdle clearance
parameters in the individual sequences are close to
each other, which indicates the repeatability of the
movement performed by the competitor.
4 CONCLUSIONS
This paper has proposed a human motion tracking
method that can be deployed and run on a mobile de-
vice. The method can be used by coaches for the eva-
luation of the athlete’s technique. This system was
tested on two mobile development platforms and three
image sequences of an athlete clearing a hurdle which
were recorded using a smartphone. In the performed
experiments, the hurdle clearance parameters were es-
timated based on the human poses obtained. An ana-
lysis of the errors received showed that the most accu-
rately estimated parameter was the height of the cen-
tre of mass h, while the biggest errors were observed
for the bending angle of the knee for the trail leg α.
Our future work will focus on improving the pro-
posed method and preparing the application for the
Android platform.
ACKNOWLEDGEMENTS
This work has been partially supported by the Polish
Ministry of Science and Higher Education within the
research project ”Development of Academic Sport”
in the years 2016-2019, project No. N RSA4 00554.
REFERENCES
Baca, A., Kornfeind, P., Preuschl, E., Bichler, S., Tampier,
M., and Novatchkov, H. (2010). A server-based mo-
bile coaching system. Sensors, 10(12):10640–10662.
ˇ
Coh, M. (2003). Biomechanical analysis of Colin Jackson’s
hurdle clearance technique. New Studies in Athletics,
1:33–40.
Deutscher, J. and Reid, I. (2005). Articulated body motion
capture by stochastic search. Int. J. Comput. Vision,
61(2):185–205.
Gutierrez-Martinez, J.-M., Castillo-Martinez, A., Medina-
Merodio, J.-A., Aguado-Delgado, J., and Martinez-
Herraiz, J.-J. (2017). Smartphones as a light measure-
ment tool: Case of study. Applied Sciences, 7(6).
Jensen, U., Schmidt, M., Hennig, M., Dassler, F. A., Jaitner,
T., and Eskofier, B. M. (2015). An IMU-based mo-
bile system for golf putt analysis. Sports Engineering,
18(2):123–133.
John, V., Trucco, E., and Ivekovic, S. (2010). Markerless
human articulated tracking using hierarchical particle
swarm optimisation. Image and Vision Computing,
28(11):1530–1547.
KaewTraKulPong, P. and Bowden, R. (2002). An impro-
ved adaptive background mixture model for realtime
tracking with shadow detection. In Proceedings of 2nd
European Workshop on Advanced Video-Based Sur-
veillance Systems; September 4, 2001; London, U.K.
Kennedy, J. and Eberhart, R. (1995). Particle swarm opti-
mization. In Proc. of IEEE Int. Conf. on Neural Net-
works, volume 4, pages 1942–1948. IEEE Press, Pis-
cataway, NJ.
Koyama, Y. and Watanabe, K. (2014). Auditory golf co-
aching system using perceptive sportswear based on
hetero-core optics. In 2014 IEEE 3rd Global Confe-
rence on Consumer Electronics (GCCE), pages 643–
646.
Kranz, M., M
¨
oller, A., Hammerla, N., Diewald, S., Pl
¨
otz,
T., Olivier, P., and Roalter, L. (2013). The mobile fit-
ness coach: Towards individualized skill assessment
using personalized mobile devices. Pervasive and Mo-
bile Computing, 9(2):203–215. Special Section: Mo-
bile Interactions with the Real World.
Krzeszowski, T., Przednowek, K., Wiktorowicz, K., and
Iskra, J. (2016). Estimation of hurdle clearance pa-
rameters using a monocular human motion tracking
method. Computer Methods in Biomechanics and
Biomedical Engineering, 19(12):1319–1329. PMID:
26838547.
Kwolek, B., Krzeszowski, T., Gagalowicz, A., Wojciechow-
ski, K., and Josinski, H. (2012). Real-time multi-view
human motion tracking using particle swarm optimi-
zation with resampling. In Perales, F., Fisher, R., and
Moeslund, T., editors, Articulated Motion and Defor-
mable Objects, volume 7378 of Lecture Notes in Com-
puter Science, pages 92–101. Springer Berlin Heidel-
berg.
Mitchell, E., Monaghan, D., and O’Connor, N. E. (2013).
Classification of sporting activities using smartphone
accelerometers. Sensors, 13(4):5317–5337.
Moeslund, T., Hilton, A., and Kr
¨
uger, V. (2006). A sur-
vey of advances in vision-based human motion cap-
ture and analysis. Computer Vision and Image Under-
standing, 104(2-3):90–127.
M
¨
oller, A., Scherr, J., Roalter, L., Diewald, S., Hammerla,
N., Pl
¨
otz, T., Olivier, P., and Kranz, M. (2011). Gym-
skill: mobile exercise skill assessment to support per-
sonal health and fitness. In Adjunct Proceedings of
icSPORTS 2018 - 6th International Congress on Sport Sciences Research and Technology Support
54