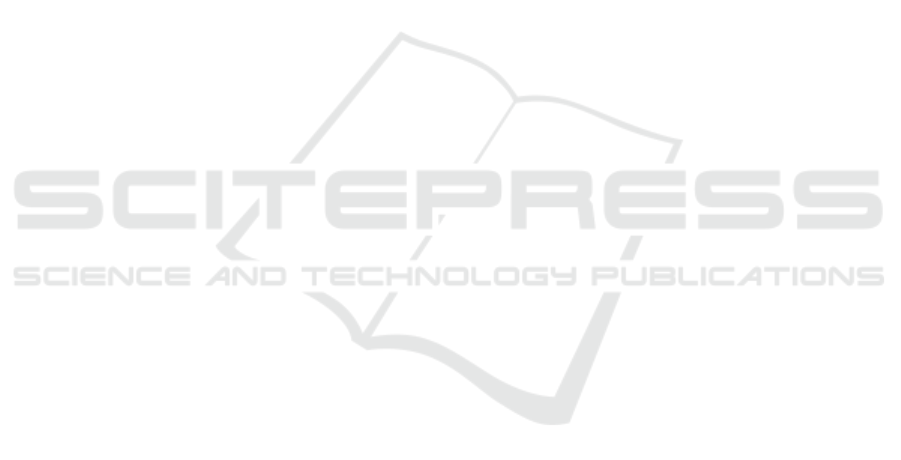
ure 12 confirms that with corese-cent or alleg-cent in
the Educlever industrial context, the execution time
of queries with a few property paths in the graph pat-
tern, like it is the case for Q
12
, remains under 200
ms in average, which is acceptable for a Web appli-
cation. But, for more complex queries, like Q
13
and
Q
14
, the execution time can reach up to to 4000 ms
(4s), which is not acceptable in the Educlever indus-
trial context. This is among our next challenges to
find a convenient architecture to handle such queries,
with pre-processed results.
6 CONCLUSIONS
In this paper, we reported a knowledge modelling
experience in an industrial context to propose an
e-Education solution compliant with public educa-
tion specifications based on semantic Web models
and technologies. We briefly presented the ontology
Eduprogression which describes a shared conceptual-
ization of knowledge pieces and skill in the educa-
tional context and we showed how we used it and
extended it to model the specific needs of a com-
pany (Educlever) for the E-Education solution they
develop. Then we described the proof of concept
we developed and deployed in the real industrial con-
text of Educlever. It relies on two ontologies, Ref-
erential populated by all the elements of knowledge
and skill (Cocons) available on the Educlever learning
platform, and Corpus populated by all the pedagog-
ical resources available on the Educlever platform.
We developed a base of SPARQL queries to imple-
ment the Educlever uses cases and we proposed two
software architectures based on Semantic Web tech-
nologies designed for an e-Education systems. We
upgraded the Educlever software architecture follow-
ing these propositions and implemented these archi-
tectures with four triplestores Corese, Allegrograph,
GraphDB and Virtuoso in order to benchmark them
and compare them to the existing solution on real data
and real queries.
We presented a complete evaluation of the quality
of service and response time in an industrial context
with a real-world tesbed showing that the Semantic
Web based solution meets the industrial requirements,
both in terms of functionalities and efficiency com-
pared to existing operational solutions. Moreover, by
relying on semantic Web we can reuse, extend and
align with existing vocabularies to increase interop-
erability. We showed this by implementing the in-
troduction of the standard ScolomFR with links to
the Educlever ontologies. With our propositions, it
is also now possible to share OPDs and integrate Co-
cons with other e-Education systems, provided that
they comply with the Eduprogression modeling.
In this context we also showed that an ontology-
oriented modelling opens up new opportunities. One
of the next challenges for us is the modeling of
learner profiles as an additional populated ontology
integrated with Referential and Corpus and the devel-
opment of SPARQL queries and rule-based reasoning
mechanisms for resource recommendation and adap-
tive learning. We also plan to link pedagogical re-
sources from several educational organizations in or-
der to build an integrated educational solution offer-
ing the learner a coherent learning path across a set of
educational systems, based on dynamically federated
endpoints.
REFERENCES
Al-Yahya, M., Al-Faries, A., and George, R. (2013).
Curonto: An ontological model for curriculum repre-
sentation. In Proceedings of the 18th ACM Conference
on Innovation and Technology in Computer Science
Education, ITiCSE ’13, pages 358–358, New York,
NY, USA. ACM.
Al-Yahya, M., George, R., and Al-Faries, A. (2015). On-
tologies in e-learning: Review of the literature. In-
ternational Journal of Software Engineering and its
Applications, 9:67–84.
Alsultanny, Y. A. (2006). e-learning system overview
based on semantic web. The Electronic Journal of e-
Learning, 4:111–118.
Arias, M., Fern
´
andez, J. D., Mart
´
ınez-Prieto, M. A., and
de la Fuente, P. (2011). An empirical study of real-
world SPARQL queries. CoRR, abs/1103.5043.
committee, I.-L.-L. (2002). IEEE Standard for Learn-
ing Object Metadata. IEEE Standards Association.
https://standards.ieee.org/findstds/standard/1484.12.1-
2002.html.
Gascue
˜
na, J. M., Fern
´
andez-Caballero, A., and Gonz
´
alez, P.
(2006). Domain ontology for personalized e-learning
in educational systems. In Advanced Learning Tech-
nologies, IEEE International Conference on (ICALT),
pages 456–458.
Guangzuo, C., Fei, C., Hu, C., and Shufang, L. (2004). On-
toedu: a case study of ontology-based education grid
system for e-learning. In In: GCCCE2004 Interna-
tional conference, Hong Kong.
Gueffaz, M., Deslis, J., and Moissinac, J.-C. (2014). Cur-
riculum data enrichment with ontologies. In Proceed-
ings of the 4th International Conference on Web In-
telligence, Mining and Semantics (WIMS14), WIMS
’14, pages 441–446, New York, NY, USA. ACM.
Hyun-Sook, C. and Jung-Min, K. (2012). Ontology de-
sign for creating adaptive learning path in e-learning
environment. In Proceedings of International Mul-
tiConference of Engineers and Computer Scientists,
(IMECS 2012), IMECS’2012, pages 585–5886.
Semantic Models in Web based Educational System Integration
87