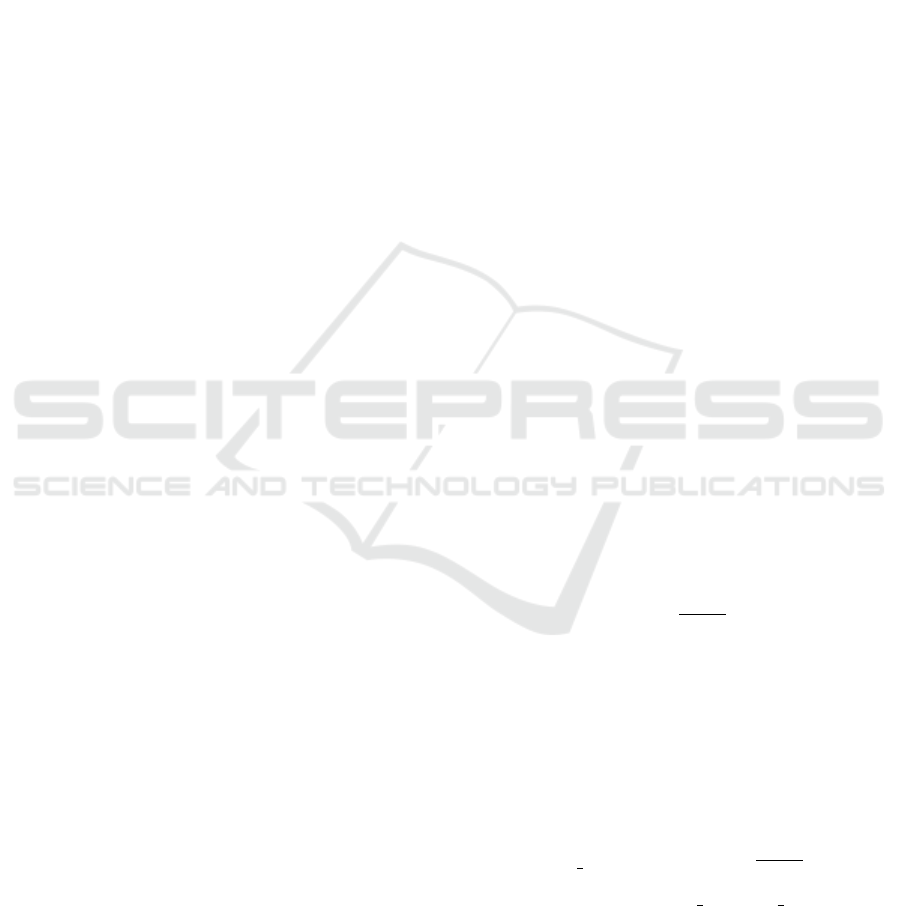
Local Lyapunov Functions for Nonlinear Stochastic
Differential Equations by Linearization
Hj
¨
ortur Bj
¨
ornsson
1
, Peter Giesl
2
, Skuli Gudmundsson
3
and Sigurdur Hafstein
1
1
Science Institute and Faculty of Physical Sciences, University of Iceland, Dunhagi 5, 107 Reykjav
´
ık, Iceland
2
Department of Mathematics, University of Sussex, Falmer, BN1 9QH, U.K.
3
Svensk Exportkredit, Klarabergsviadukten 61-63, 11164 Stockholm, Sweden
Keywords:
Stochastic Differential Equation, Lyapunov Function, Linearization, Asymptotic Stability in Probability.
Abstract:
We present a rigid estimate of the domain, on which a Lyapunov function for the linearization of a nonlinear
stochastic differential equation is a Lyapunov function for the original system. By using this estimate the
demanding task of computing a lower bound on the γ-basin of attraction for a nonlinear stochastic systems is
greatly simplified and the application of a resent numerical method for the same purpose facilitated.
1 INTRODUCTION
When analysing the stability of an equilibrium of a
nonlinear deterministic system
˙
x = f(x), f : R
d
→R
d
,
one often resorts to linearization around the equilib-
rium. Assuming, without restriction of generality, that
the equilibrium in question is at the origin, then one
analyzes the stability of the origin for the system
˙
x =
Ax, where A := Df(0) is the Jacobian of f at the origin.
Now, if the matrix A is Hurwitz, i.e. the real-parts of
the eigenvalues of A are all strictly negative, then one
can solve the Lyapunov equation A
>
P + PA = −Q,
where Q ∈ R
d×d
is an arbitrary symmetric and pos-
itive definite matrix. The solution P ∈ R
d×d
is then
symmetric and positive definite and V (x) = x
>
Px is
a Lyapunov function for the system, i.e. V has a min-
imum at the equilibrium at the origin and the deriva-
tive of V along solution trajectories of the linearized
system fulfills
∇V (x)
•
Ax = −x
>
Qx
and is thus negative on R
d
\{0}. The function V will
also be a Lyapunov function for the original nonlinear
system
˙
x = f(x) on a neighbourhood N of the origin
where
V
0
(x) = ∇V (x)
•
f(x) < 0 for x ∈N \{0}.
Here V
0
denotes the orbital derivative of the system.
The size of the set N is of great importance because
compact sublevel sets of V that are within N are
lower bounds on the equilibrium’s basin of attraction,
i.e. the set of points which converge to the equilibrium
as time goes to infinity. Explicit bounds for the size of
N are quite easily derived, cf. e.g. (Hafstein, 2004).
In this paper we will derive such an estimate, but for
the considerably more demanding case of stochastic
differential equations.
Notation: We denote by kxk the Euclidian norm
of a vector x ∈ R
d
and for A ∈ R
d×d
by kAk =
max
kxk=1
kAxk the matrix norm induced by the Eu-
clidian vector norm. Vectors are assumed to be col-
umn vectors.We denote by κ(A) := kAkkA
−1
k the
condition number with respect to the k · k norm of
the nonsingular matrix A ∈ R
d×d
. For a symmet-
ric and positive definite Q ∈ R
d×d
we define the en-
ergetic norm kxk
Q
:=
p
x
>
Qx and the correspond-
ing induced matrix norm kAk
Q
:= max
kxk
Q
=1
kAxk
Q
.
Recall that a symmetric and positive definite Q ∈
R
d×d
can be factorized as Q = ODO
>
where O ∈
R
d×d
is orthogonal, i.e. O
>
O = O
>
O = I and D =
diag(λ
1
, λ
2
, . . ., λ
d
) ∈ R
d×d
is a diagonal matrix with
0 < λ
1
≤ λ
2
≤ . . . ≤ λ
d
. For every a ∈ R we define
the matrix Q
a
= O diag(λ
a
1
, λ
a
2
, . . . , λ
a
d
)O
>
. It is not
difficult to see that for a > 0 we have kQ
a
k = λ
a
d
and
kQ
−a
k = λ
−a
1
. Further,
kQ
−
1
2
k
−1
kxk ≤ kxk
Q
=
p
x
>
Qx
= kQ
1
2
xk ≤ kQ
1
2
kkxk.
We consider d-dimensional systems and in all sums
where the upper and lower bounds of the sum are
omitted they are assumed to be 1 and d respectively,
i.e.
∑
i
:=
∑
d
i=1
,
∑
i, j
:=
∑
d
i, j=1
etc.
A function α : R
+
→R
+
is said to be of class K
∞
Björnsson, H., Giesl, P., Gudmundsson, S. and Hafstein, S.
Local Lyapunov Functions for Nonlinear Stochastic Differential Equations by Linearization.
DOI: 10.5220/0006944505790586
In Proceedings of the 15th International Conference on Informatics in Control, Automation and Robotics (ICINCO 2018) - Volume 1, pages 579-586
ISBN: 978-989-758-321-6
Copyright © 2018 by SCITEPRESS – Science and Technology Publications, Lda. All rights reserved
579