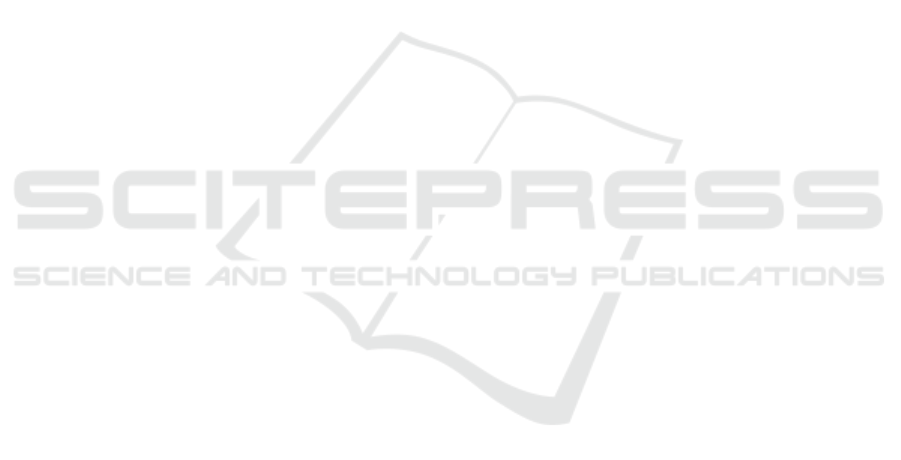
cal features (tempo, mode, harmony, loudness, pitch,
etc.) an emotions categories (happiness, sadness, an-
ger, fear, tenderness) is reported, each independent
parameter is presumably insufficient to decide about
one emotion; on the contrary this may need a lush of
musical descriptors. Many studies have demonstrated
that emotions from music are not too subjective, in-
deed within a common culture, the responses can be
greatly consonant among listeners, such that it may
be possible to replicate this in machines. The goal in
(Laurier et al., 2012) is built a system to assess mu-
sical emotions from a song. For this, supervised ma-
chine learning techniques are used.
Related with these concepts in (Fritz et al., 2009) a
cross-cultural study is done. Two sets of subjects par-
ticipated: native African population (Mafa) and Wes-
tern population. Each group listened the music of the
other respective culture is done. The skill to identify
three basic emotion (joy, sad, fear) from Western mu-
sic is investigated in experiment 1. Results show that
emotions from Western songs are universally recog-
nized (Mafa identified the three basic emotions). The
second experiment analyzed pleasantness levels chan-
ges due to spectral manipulation of music in both sub-
ject sets. Several spectral features were altered, like
sensory dissonance. The manipulated songs were un-
preferred with respect to original versions, such that
consonance and dissonance of the music may univer-
sally influence in the pleasantness level.
In (Vieillard et al., 2008) the aim is validated 56
musical extracts.The stimuli were composed with film
genre music. These transmitted four emotions depen-
ding of music features (happy, sad, threat and peace-
fulness), so the study provides suitable material for
research on emotions. In Ekman’s classification sets
happiness, sadness and threat as basic emotions (Ek-
man et al., 1972). The fourth emotion, peacefulness,
was added as oppositeness to threat. These emotions
can be defined in the 2-dimensional space from va-
lence and arousal model.
In (McAdams et al., 2017) is said that ” Of interest
to both music psychology and music informatics from
a computational point of view is the relation between
the acoustic properties that give rise to the timbre at
a given pitch and the perceived emotional quality of
the tone. Musician and non musician listeners heard
137 tones generated at a set dynamic marking (forte)
playing tones at pitch class D across each instru-
ment’s whole pitch interval and with several playing
techniques for standard orchestral instruments drawn
from the brass, woodwind, string, and pitched per-
cussion families”. They scored each tone on six
analogical-categorical scales in terms of valence (po-
sitive/negative and pleasant/unpleasant), energy arou-
sal (awake/tired), tension arousal (excited/calm), pre-
ference (like/dislike), and familiarity. Twenty-three
audio descriptors from the ”Timbre Toolbox” were
processed for each audio and analyzed in two ways
(Peeters et al., 2011): linear partial least squares re-
gression and nonlinear artificial neural net modeling.
These two analyses coincided in terms of the signi-
ficance of various audio descriptors in revealing the
emotion ratings, but some differences were found,
such that, distinct acoustic properties are being sug-
gested.
In (Soleymani et al., 2013) a dataset contains 1000
songs, each one annotated by a minimum of 10 sub-
jects is presented, which is larger than many currently
available music emotion dataset. This study supplies
a dataset for music emotion recognition research and
a baseline system. The dataset consists entirely of cre-
ative commons music from the Free Music Archive,
which as the name suggests, can be shared freely wit-
hout penalty.
The aims of (Rodà et al., 2014) are: check how
music excerpt are grouped as a function of the con-
straints applied to the stimuli; to study which dimen-
sions, accompanied by valence and arousal, can be
employed to represent emotional features of music; to
establish computable musical parameters related with
those dimensions in classification activities. The uses
of verbal labels to express emotions is avoided. Parti-
cipant were asked to completely focused on their own
feelings from musical extracts and to group that trans-
mitted similar subjective emotions.
During recent years neuroscientific research on
music-evoked emotions have increased and in (Koel-
sch, 2014) it can be found a recompilation of studies
of brain structures involve in this. In this work is esta-
blished that the emotional effects caused by music can
be motivated by memory associated with music but a
part of them are induced only by the music itself. In
the previous works, the emotions aroused by the mu-
sic were evaluated using two sources of information:
on the one hand, the extraction of the musical cha-
racteristics of the audios and on the other, the testing
of the users where the feeling of being causes a cer-
tain piece of music This definition can be made using
either the dimensional representation of the emotions
or the categorical one. One way to objectively mea-
sure the emotion desperate for music is to measure the
physiological response that the hearing causes.
The objective of (Goshvarpour et al., 2016) is to
propose an accurate emotion recognition methodo-
logy. To this end, a novel fusion framework based on
wavelet transform, and matching pursuit (MP) algo-
rithm is chosen. Electrocardiogram (ECG) and galva-
nic skin response (GSR) of 11 healthy students were
A Preliminary Study about the Music Influence on EEG and ECG Signals
101